Variation In Model Performance By Data Cleanliness And Classification Methods In The Prediction Of 30-Day Icu Mortality, A Us Nationwide Retrospective Cohort And Simulation Study
BMJ OPEN(2020)
摘要
Objective There has been a proliferation of approaches to statistical methods and missing data imputation as electronic health records become more plentiful; however, the relative performance on real-world problems is unclear. Materials and methods Using 355 823 intensive care unit (ICU) hospitalisations at over 100 hospitals in the nationwide Veterans Health Administration system (2014-2017), we systematically varied three approaches: how we extracted and cleaned physiologic variables; how we handled missing data (using mean value imputation, random forest, extremely randomised trees (extra-trees regression), ridge regression, normal value imputation and case-wise deletion) and how we computed risk (using logistic regression, random forest and neural networks). We applied these approaches in a 70% development sample and tested the results in an independent 30% testing sample. Area under the receiver operating characteristic curve (AUROC) was used to quantify model discrimination. Results In 355 823 ICU stays, there were 34 867 deaths (9.8%) within 30 days of admission. The highest AUROCs obtained for each primary classification method were very similar: 0.83 (95% CI 0.83 to 0.83) to 0.85 (95% CI 0.84 to 0.85). Likewise, there was relatively little variation within classification method by the missing value imputation method used-except when casewise deletion was applied for missing data. Conclusion Variation in discrimination was seen as a function of data cleanliness, with logistic regression suffering the most loss of discrimination in the least clean data. Losses in discrimination were not present in random forest and neural networks even in naively extracted data. Data from a large nationwide health system revealed interactions between missing data imputation techniques, data cleanliness and classification methods for predicting 30-day mortality.
更多查看译文
关键词
health informatics, statistics &, research methods, adult intensive &, critical care
AI 理解论文
溯源树
样例
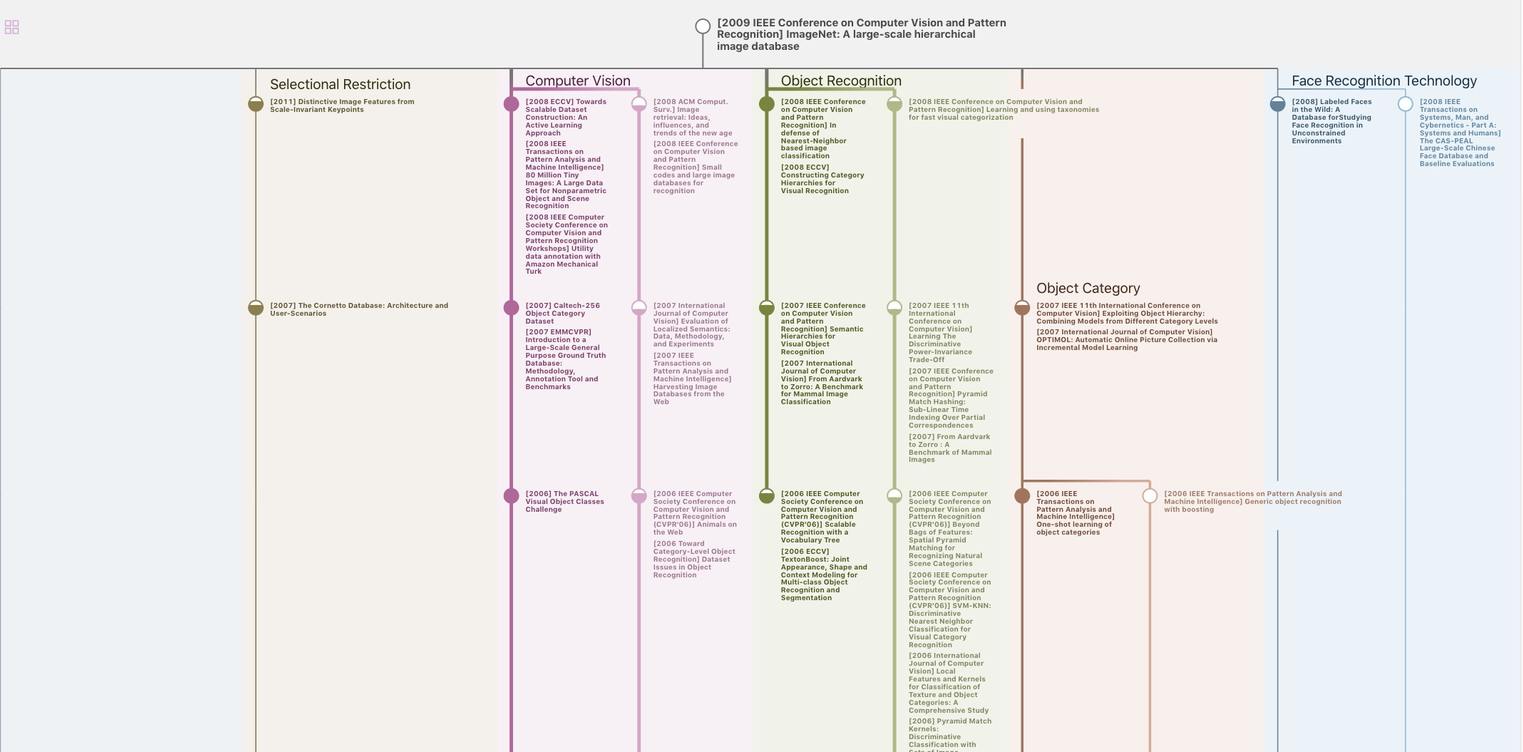
生成溯源树,研究论文发展脉络
Chat Paper
正在生成论文摘要