Deep Inverse Sensor Models as Priors for evidential Occupancy Mapping
IROS(2020)
摘要
With the recent boost in autonomous driving, increased attention has been paid on radars as an input for occupancy mapping. Besides their many benefits, the inference of occupied space based on radar detections is notoriously difficult because of the data sparsity and the environment dependent noise (e.g. multipath reflections). Recently, deep learning-based inverse sensor models, from here on called deep ISMs, have been shown to improve over their geometric counterparts in retrieving occupancy information. Nevertheless, these methods perform a data-driven interpolation which has to be verified later on in the presence of measurements. In this work, we describe a novel approach to integrate deep ISMs together with geometric ISMs into the evidential occupancy mapping framework. Our method leverages both the capabilities of the data-driven approach to initialize cells not yet observable for the geometric model effectively enhancing the perception field and convergence speed, while at the same time use the precision of the geometric ISM to converge to sharp boundaries. We further define a lower limit on the deep ISM estimate's certainty together with analytical proofs of convergence which we use to distinguish cells that are solely allocated by the deep ISM from cells already verified using the geometric approach.
更多查看译文
关键词
deep inverse sensor models,autonomous driving,occupied space,radar detections,data sparsity,environment dependent noise,deep learning-based inverse sensor models,data-driven interpolation,geometric ISM,data-driven approach,geometric model,deep ISM,evidential occupancy mapping,multipath reflections,perception field,convergence speed
AI 理解论文
溯源树
样例
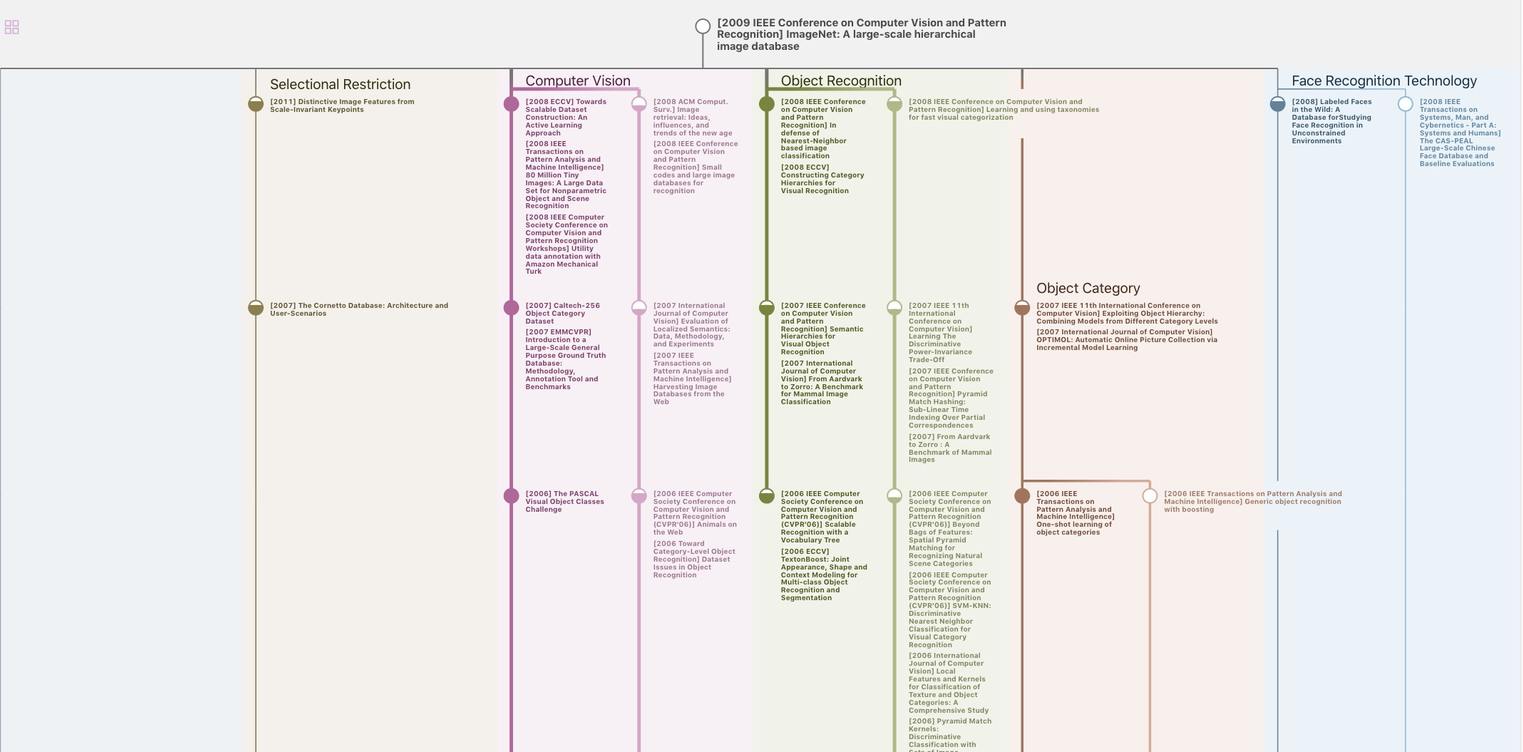
生成溯源树,研究论文发展脉络
Chat Paper
正在生成论文摘要