Scan2Cap: Context-aware Dense Captioning in RGB-D Scans
2021 IEEE/CVF CONFERENCE ON COMPUTER VISION AND PATTERN RECOGNITION, CVPR 2021(2021)
摘要
We introduce the task of dense captioning in 3D scans from commodity RGB-D sensors. As input, we assume a point cloud of a 3D scene; the expected output is the bounding boxes along with the descriptions for the underlying objects. To address the 3D object detection and description problems, we propose Scan2Cap, an end-to-end trained method, to detect objects in the input scene and describe them in natural language. We use an attention mechanism that generates descriptive tokens while referring to the related components in the local context. To reflect object relations (i.e. relative spatial relations) in the generated captions, we use a message passing graph module to facilitate learning object relation features. Our method can effectively localize and describe 3D objects in scenes from the ScanRefer dataset, outperforming 2D baseline methods by a significant margin (27.61% CiDEr@0.5IoU improvement).
更多查看译文
关键词
Scan2Cap,context-aware dense captioning,RGB-D scans,commodity RGB-D sensors,point cloud,expected output,bounding boxes,underlying objects,description problems,end-to-end trained method,input scene,descriptive tokens,related components,local context,object relations,relative spatial relations,generated captions,relation features,2D baseline methods
AI 理解论文
溯源树
样例
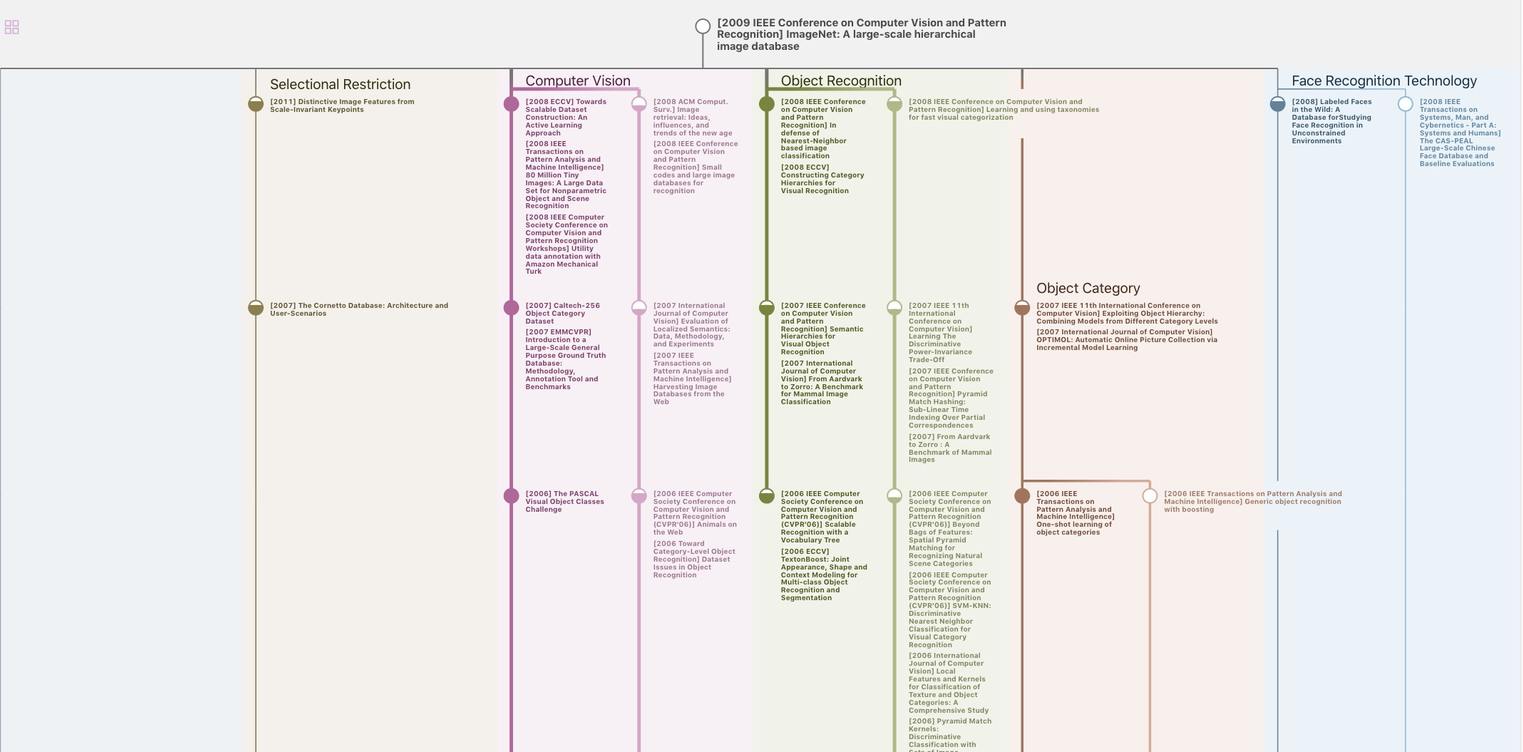
生成溯源树,研究论文发展脉络
Chat Paper
正在生成论文摘要