Penalised t-walk MCMC
Journal of Statistical Planning and Inference(2022)
摘要
Handling multimodality that commonly arises from complicated statistical models remains a challenge. Current Markov chain Monte Carlo (MCMC) methodology tackling this subject is based on an ensemble of chains targeting a product of power-tempered distributions. Despite the theoretical validity of such methods, practical implementations typically suffer from bad mixing and slow convergence due to the high-computation cost involved. In this work we study novel extensions of the t-walk algorithm, an existing MCMC method that is inexpensive and invariant to affine transformations of the state space, for dealing with multimodal distributions. We acknowledge that the effectiveness of the new method will be problem dependent and might struggle in complex scenarios; for such cases we propose a post-processing technique based on pseudo-marginal theory for combining isolated samples.
更多查看译文
关键词
Bayesian inference,Multimodality,Monte Carlo,MCMC,t-walk,Pseudo-marginal
AI 理解论文
溯源树
样例
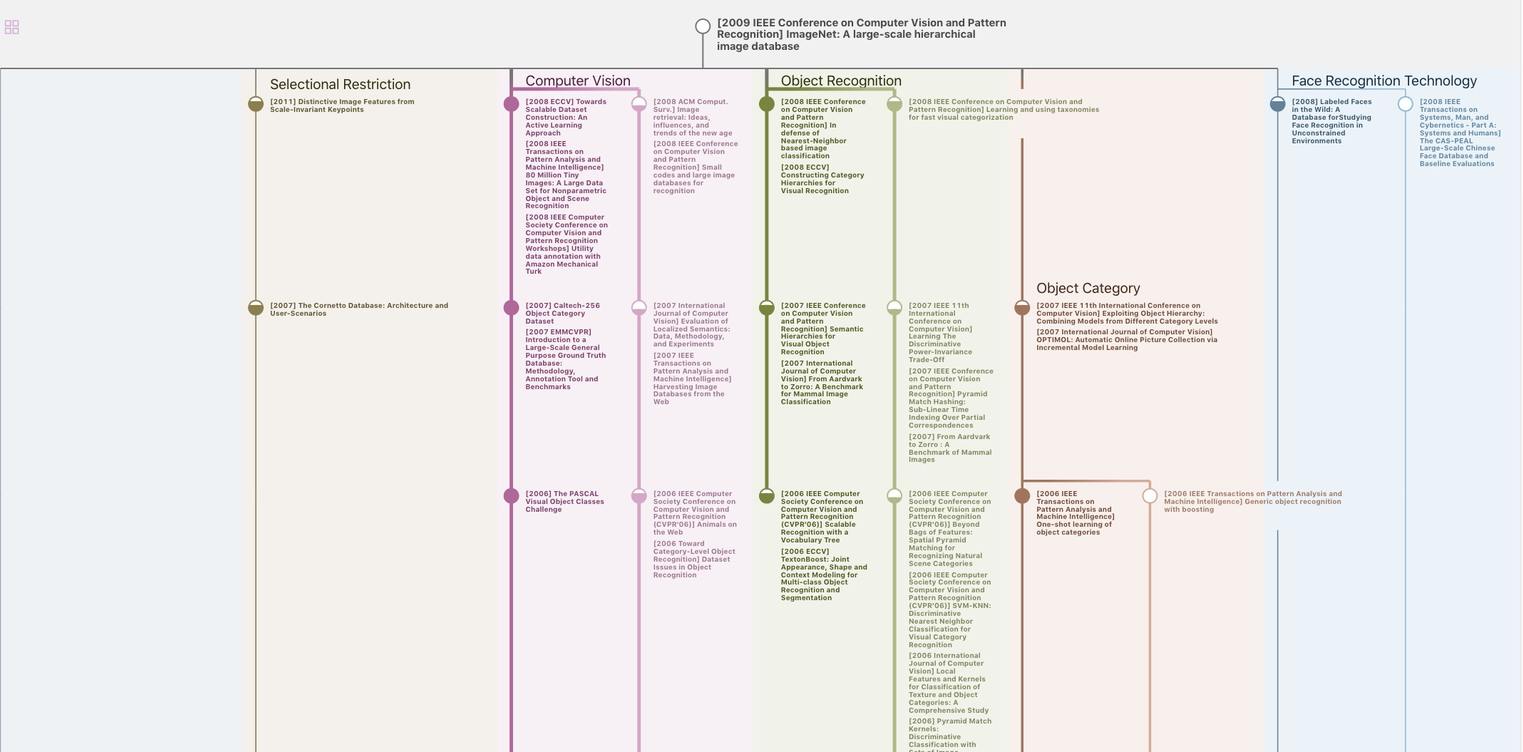
生成溯源树,研究论文发展脉络
Chat Paper
正在生成论文摘要