RLOC: Terrain-Aware Legged Locomotion Using Reinforcement Learning and Optimal Control
IEEE transactions on robotics(2022)
摘要
We present a unified model-based and data-driven approach for quadrupedal planning and control to achieve dynamic locomotion over uneven terrain. We utilize on-board proprioceptive and exteroceptive feedback to map sensory information and desired base velocity commands into footstep plans using a reinforcement learning (RL) policy. This RL policy is trained in simulation over a wide range of procedurally generated terrains. When run online, the system tracks the generated footstep plans using a model-based motion controller. We evaluate the robustness of our method over a wide variety of complex terrains. It exhibits behaviors that prioritize stability over aggressive locomotion. Additionally, we introduce two ancillary RL policies for corrective whole-body motion tracking and recovery control. These policies account for changes in physical parameters and external perturbations. We train and evaluate our framework on a complex quadrupedal system, ANYmal version B, and demonstrate transferability to a larger and heavier robot, ANYmal C, without requiring retraining.
更多查看译文
关键词
Robots,Quadrupedal robots,Planning,Training,Computational modeling,Legged locomotion,Tracking,AI-based methods,deep learning in robotics and automation,legged robots,robust,adaptive control of robotic systems
AI 理解论文
溯源树
样例
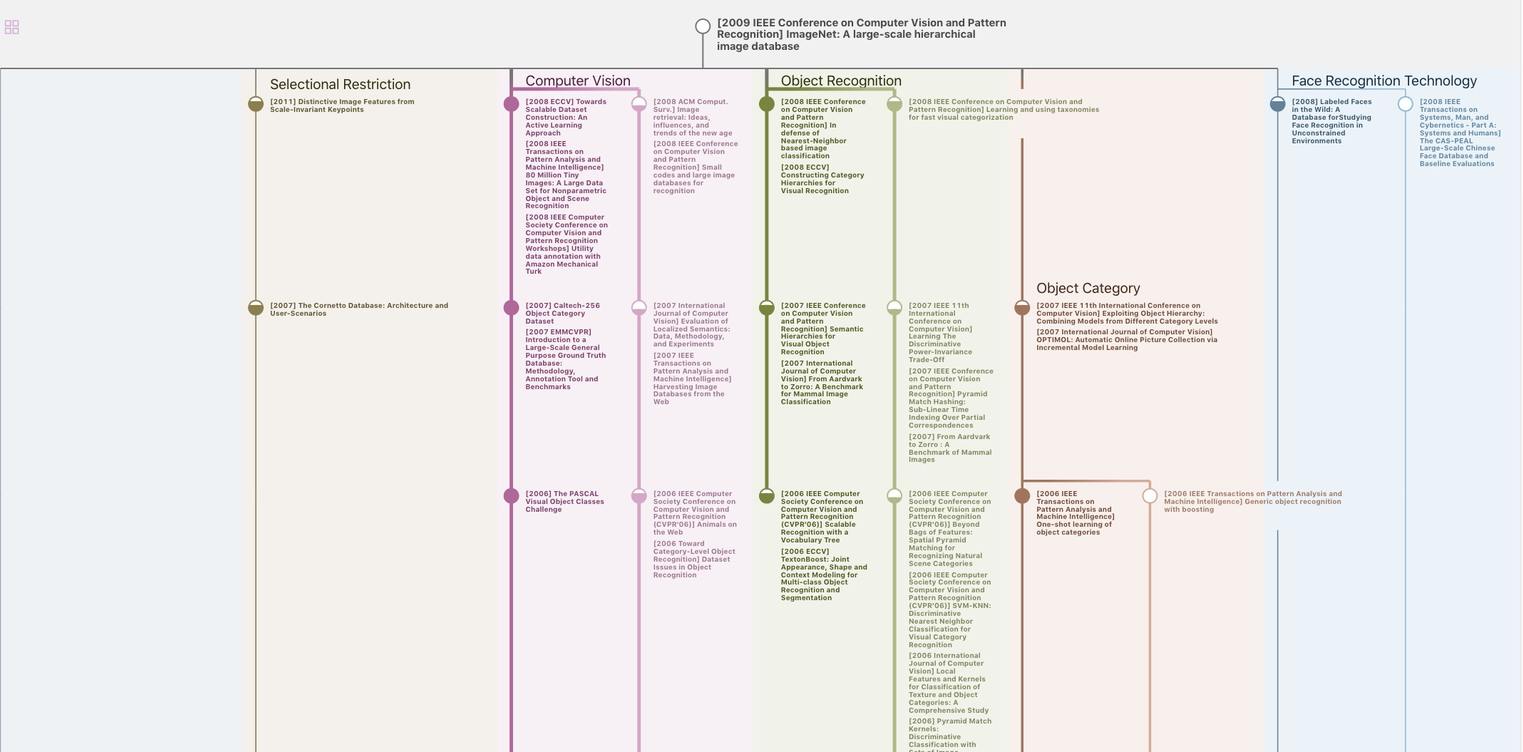
生成溯源树,研究论文发展脉络
Chat Paper
正在生成论文摘要