Seeking New Physics in Cosmology with Bayesian Neural Networks: Dark Energy and Modified Gravity
PHYSICAL REVIEW D(2022)
摘要
We study the potential of Bayesian neural networks (BNNs) to detect new physics in the dark matter power spectrum, concentrating here on evolving dark energy and modifications to general relativity. After introducing a new technique to quantify classification uncertainty in BNNs, we train two BNNs on mock matter power spectra produced using the publicly available code ReACT in the k range (0.01 - 2.5) hMpc(-1) and redshift bins (0.1, 0.478, 0.783, 1.5) with Euclid-like noise. The first network classifies spectra into five labels including Lambda CDM, f(R), wCDM, Dvali-Gabadadze-Porrati gravity and a "random" class, whereas the second is trained to distinguish Lambda CDM from non-Lambda CDM. Both networks achieve a comparable training, validation and test accuracy of similar to 95%. Each network is also capable of correctly classifying spectra with deviations from Lambda CDM that were not included in the training set, demonstrated with spectra generated using the growth index gamma. To obtain an indication of the BNNs classification capability, we compute the smallest deviation from Lambda CDM such that the noise-averaged non-Lambda CDM classification probability is at least 95% according to our estimated error quantification, finding these bounds to be f(R0) less than or similar to 10(-7), Omega(rc) less than or similar to 10(-2), -1.05 less than or similar to w(0) less than or similar to 0.95, -0.2 less than or similar to w(a) less than or similar to 0.2, and 0.52 less than or similar to gamma less than or similar to 0.59. The bounds on f(R) can be improved by training a specialist network to distinguish solely between Lambda CDM and f(R) power spectra which can detect a nonzero f(R0) at O(10(-8)). We expect that further developments, such as the inclusion of smaller length scales or additional extensions to Lambda CDM, will only improve the potential of BNNs to indicate the presence of new physics in cosmological datasets, regardless of the underlying theory.
更多查看译文
关键词
Deep Learning,Nonparametric Methods
AI 理解论文
溯源树
样例
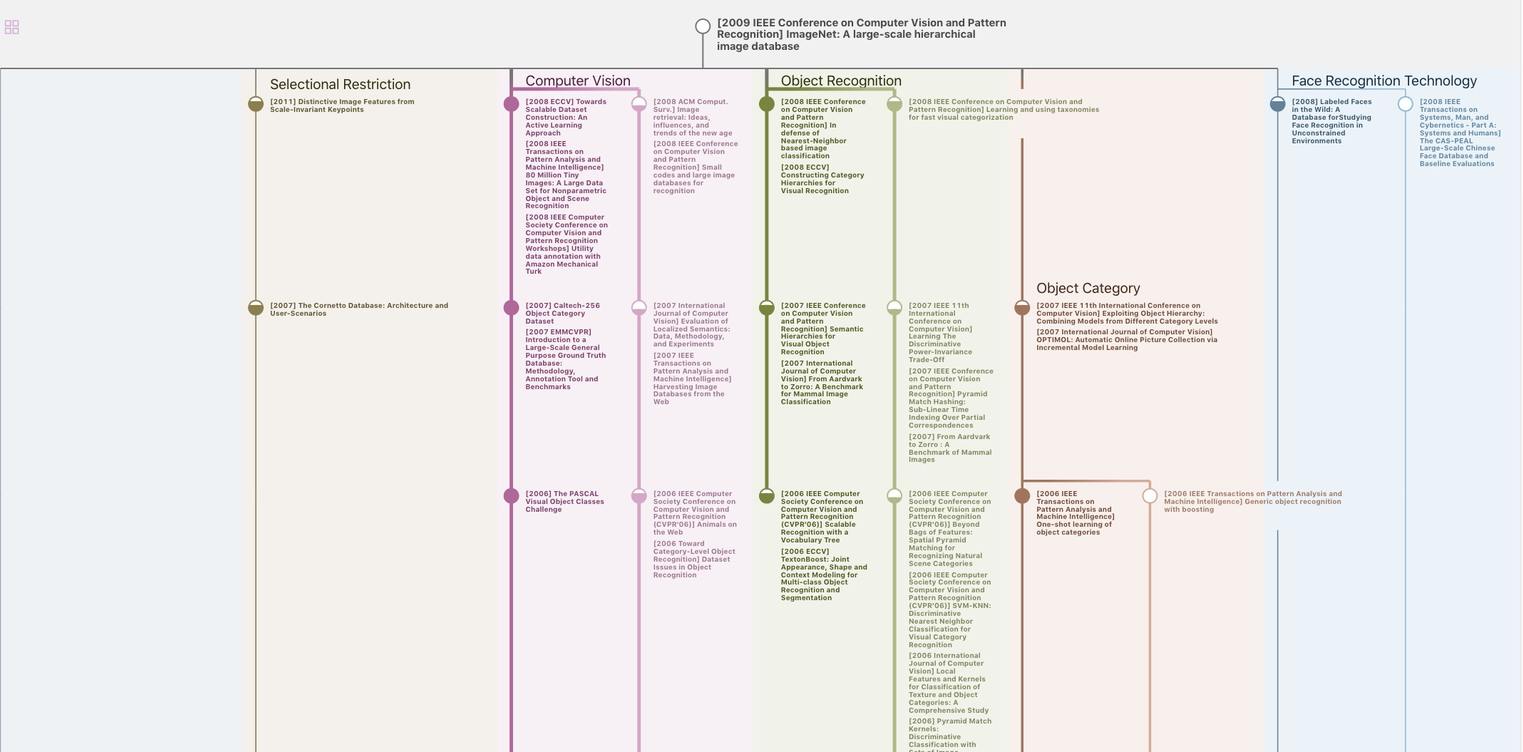
生成溯源树,研究论文发展脉络
Chat Paper
正在生成论文摘要