ANAPT: Additive Noise Analysis for Persistence Thresholding
arxiv(2022)
摘要
We introduce a novel method for Additive Noise Analysis for Persistence Thresholding (ANAPT) which separates significant features in the sublevel set persistence diagram of a time series based on a statistics analysis of the persistence of a noise distribution. Specifically, we consider an additive noise model and leverage the statistical analysis to provide a noise cutoff or confidence interval in the persistence diagram for the observed time series. This analysis is done for several common noise models including Gaussian, uniform, exponential and Rayleigh distributions. ANAPT is computationally efficient, does not require any signal pre-filtering, is widely applicable, and has open-source software available. We demonstrate the functionality ANAPT with both numerically simulated examples and an experimental data set. Additionally, we provide an efficient $\Theta(n\log(n))$ algorithm for calculating the zero-dimensional sublevel set persistence homology.
更多查看译文
关键词
Topological Signal Processing, Statistics, Topological Data Analysis, Sublevel Set Persistence, Sublevel Sets, Confidence Intervals, Persistent Homology, Signal Processing, Extrema Detection, Time Series Analysis, Cutoff
AI 理解论文
溯源树
样例
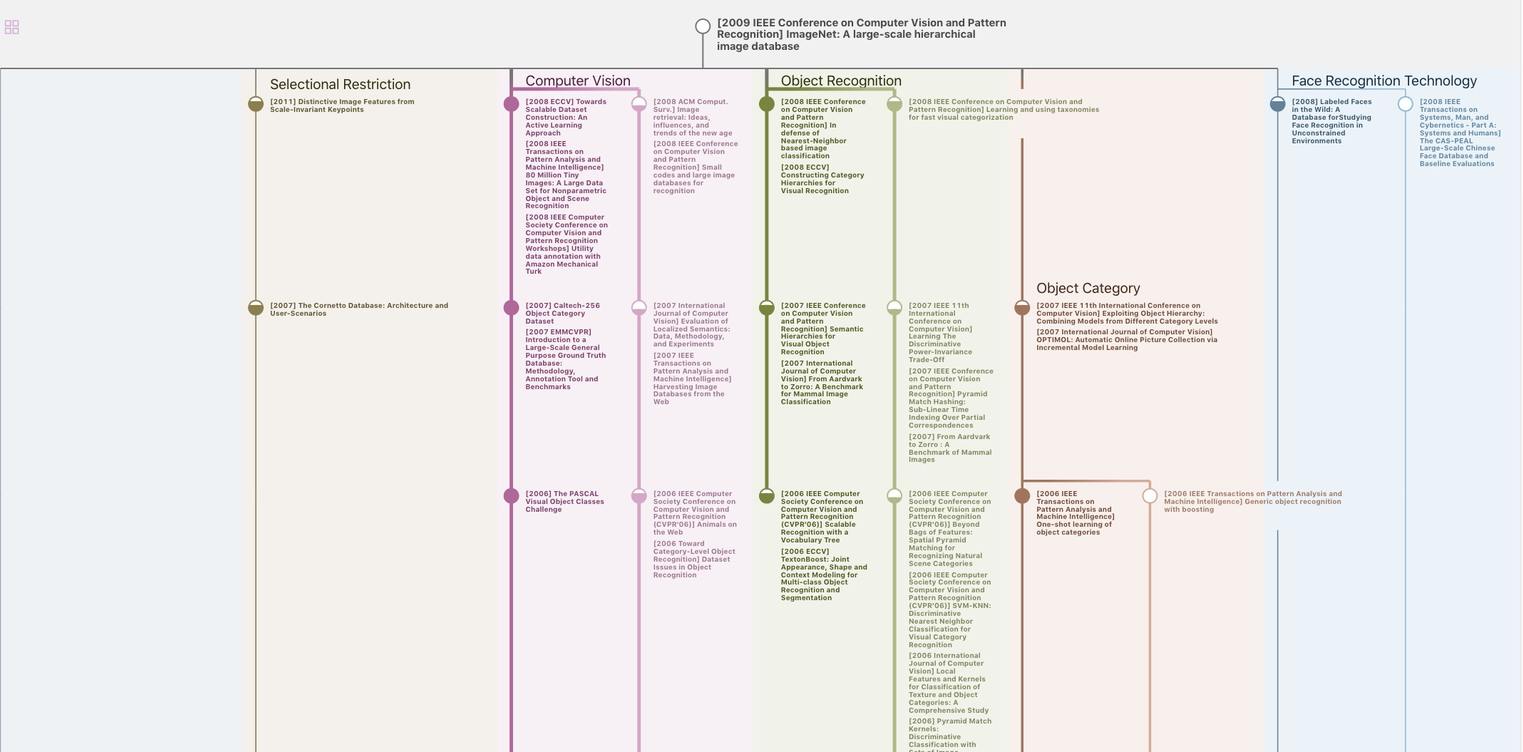
生成溯源树,研究论文发展脉络
Chat Paper
正在生成论文摘要