Rotation-Invariant Point Convolution With Multiple Equivariant Alignments.
2020 International Conference on 3D Vision (3DV)(2020)
摘要
Recent attempts at introducing rotation invariance or equivariance in 3D deep learning approaches have shown promising results, but these methods still struggle to reach the performances of standard 3D neural networks. In this work we study the relation between equivariance and invariance in 3D point convolutions. We show that using rotation-equivariant alignments, it is possible to make any convolutional layer rotation-invariant. Furthermore, we improve this simple alignment procedure by using the alignment themselves as features in the convolution, and by combining multiple alignments together. With this core layer, we design rotation-invariant architectures which improve state-of-the-art results in both object classification and semantic segmentation and reduces the gap between rotation-invariant and standard 3D deep learning approaches.
更多查看译文
关键词
Deep Learning,Point Clouds,Rotation invaraince,Rotation equivariance,Convolution
AI 理解论文
溯源树
样例
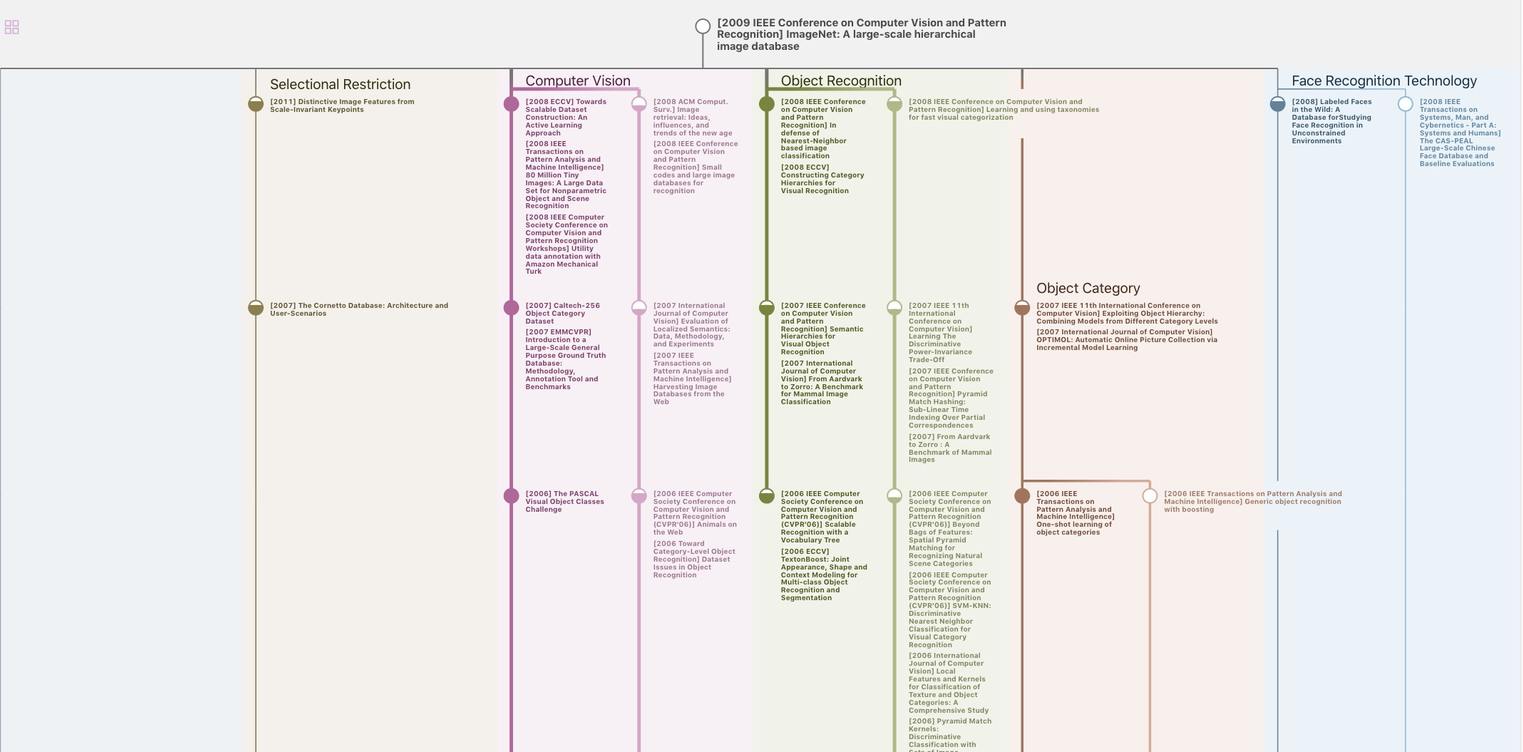
生成溯源树,研究论文发展脉络
Chat Paper
正在生成论文摘要