Concept Drift and Covariate Shift Detection Ensemble with Lagged Labels.
IEEE BigData(2021)
摘要
In model serving, having one fixed model during the entire often life-long inference process is usually detrimental to model performance, as data distribution evolves over time, resulting in lack of reliability of the model trained on historical data. It is important to detect changes and retrain the model in time. The existing methods generally have three weaknesses: 1) using only classification error rate as signal, 2) assuming ground truth labels are immediately available after features from samples are received and 3) unable to decide what data to use to retrain the model when change occurs. We address the first problem by utilizing six different signals to capture a wide range of characteristics of data, and we address the second problem by allowing lag of labels, where labels of corresponding features are received after a lag in time. For the third problem, our proposed method automatically decides what data to use to retrain based on the signals. Extensive experiments on structured and unstructured data for different type of data changes establish that our method consistently outperforms the state-of-the-art methods by a large margin.
更多查看译文
关键词
Drift Detection,Model Serving,Lagged Labels
AI 理解论文
溯源树
样例
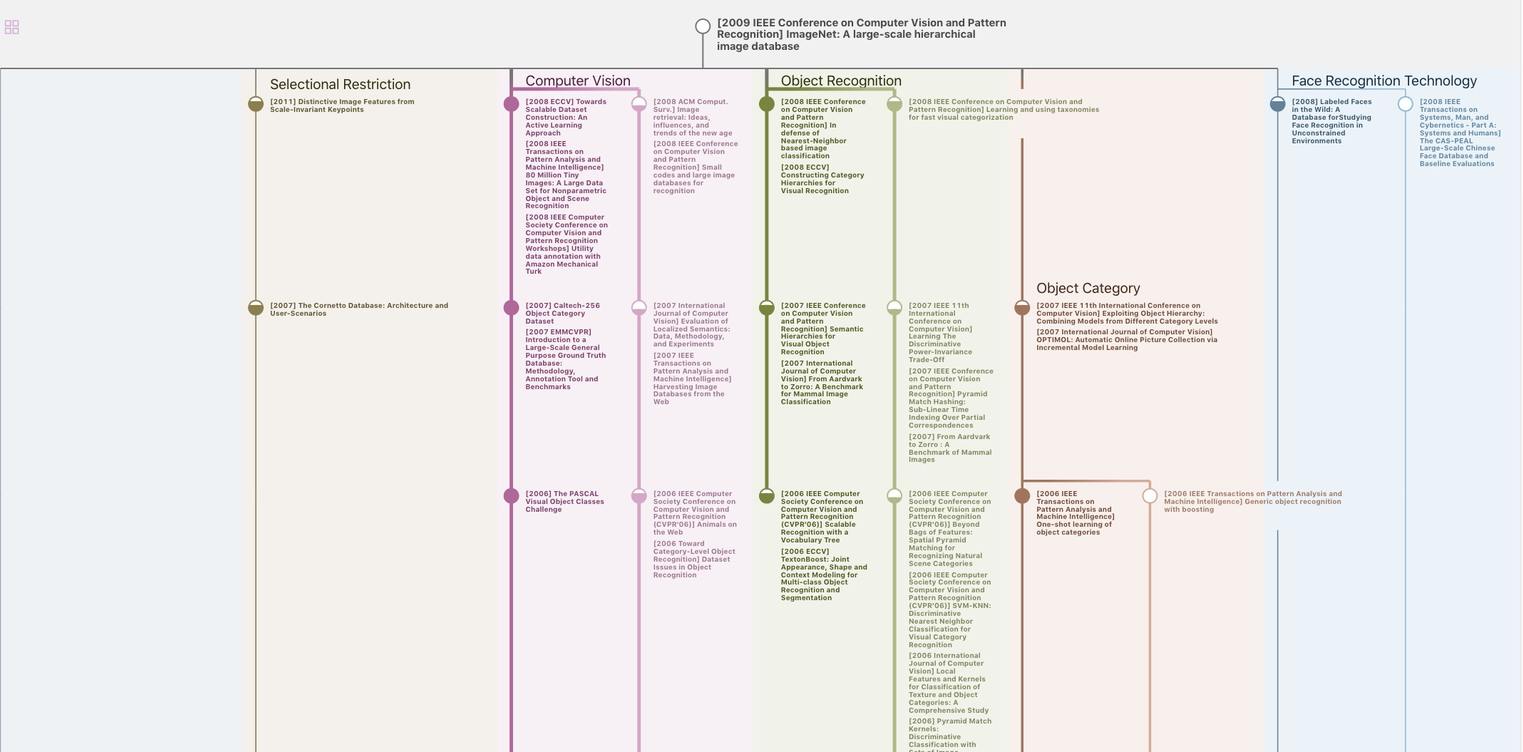
生成溯源树,研究论文发展脉络
Chat Paper
正在生成论文摘要