A Consensus-Based Global Optimization Method with Adaptive Momentum Estimation
COMMUNICATIONS IN COMPUTATIONAL PHYSICS(2022)
摘要
Objective functions in large-scale machine-learning and artificial intelligence applications often live in high dimensions with strong non-convexity and massive local minima. Gradient-based methods, such as the stochastic gradient method and Adam [15], and gradient-free methods, such as the consensus-based optimization (CBO) method, can be employed to find minima. In this work, based on the CBO method and Adam, we propose a consensus-based global optimization method with adaptive momentum estimation (Adam-CBO). Advantages of the Adam-CBO method include: It is capable of finding global minima of non-convex objective functions with high success rates and low costs. This is verified by finding the global minimizer of the 1000 dimensional Rastrigin function with 100% success rate at a cost only growing linearly with respect to the dimensionality. It can handle non-differentiable activation functions and thus approximate low-regularity functions with better accuracy. This is confirmed by solving a machine learning task for partial differential equations with low-regularity solutions where the Adam-CBO method provides better results than Adam. It is robust in the sense that its convergence is insensitive to the learning rate by a linear stability analysis. This is confirmed by finding the minimizer of a quadratic function.
更多查看译文
关键词
Consensus-based optimization, global optimization, machine learning, curse of dimensionality
AI 理解论文
溯源树
样例
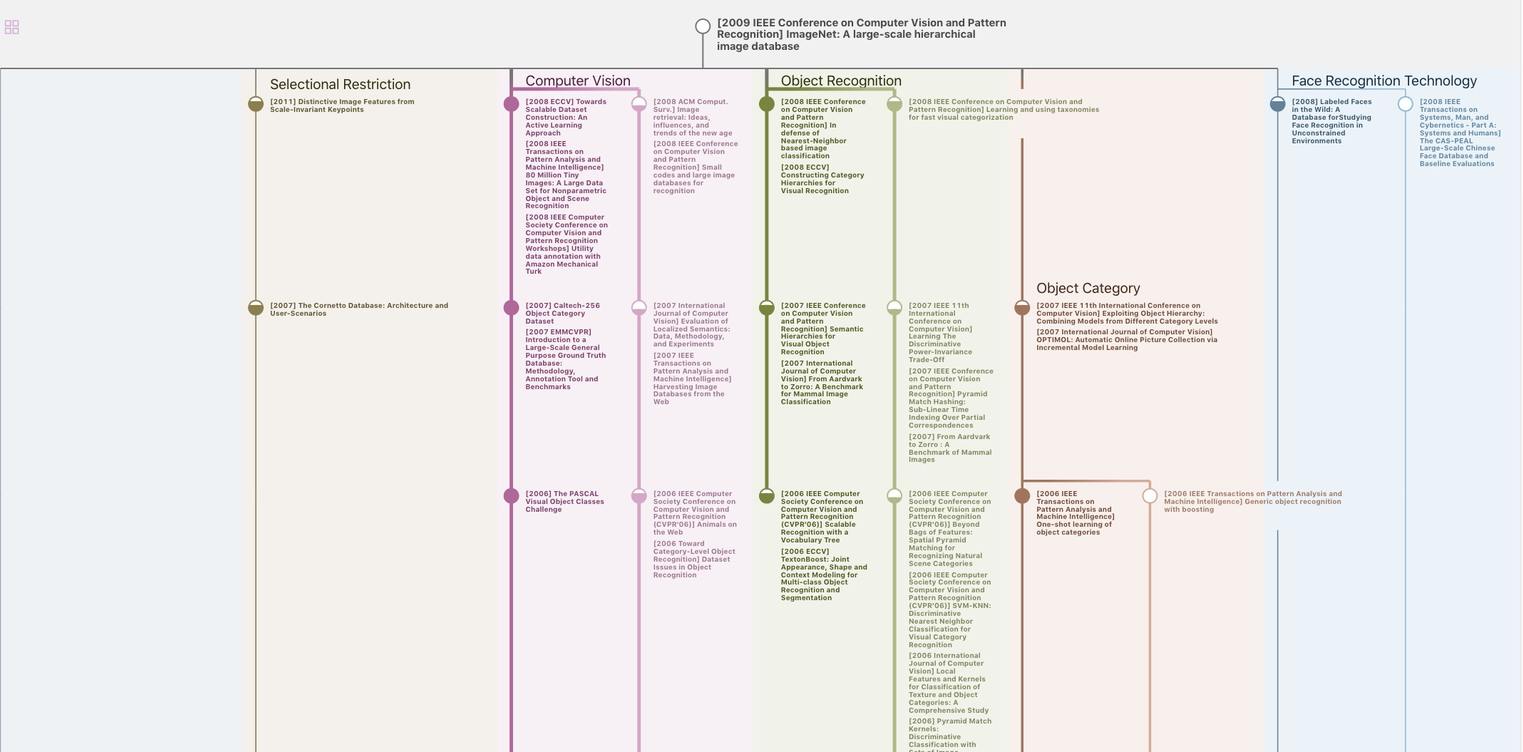
生成溯源树,研究论文发展脉络
Chat Paper
正在生成论文摘要