Scalable Neural Tangent Kernel of Recurrent Architectures
arxiv(2021)
摘要
Kernels derived from deep neural networks (DNNs) in the infinite-width provide not only high performance in a range of machine learning tasks but also new theoretical insights into DNN training dynamics and generalization. In this paper, we extend the family of kernels associated with recurrent neural networks (RNNs), which were previously derived only for simple RNNs, to more complex architectures that are bidirectional RNNs and RNNs with average pooling. We also develop a fast GPU implementation to exploit its full practical potential. While RNNs are typically only applied to time-series data, we demonstrate that classifiers using RNN-based kernels outperform a range of baseline methods on 90 non-time-series datasets from the UCI data repository.
更多查看译文
关键词
scalable neural tangent kernel,recurrent architectures
AI 理解论文
溯源树
样例
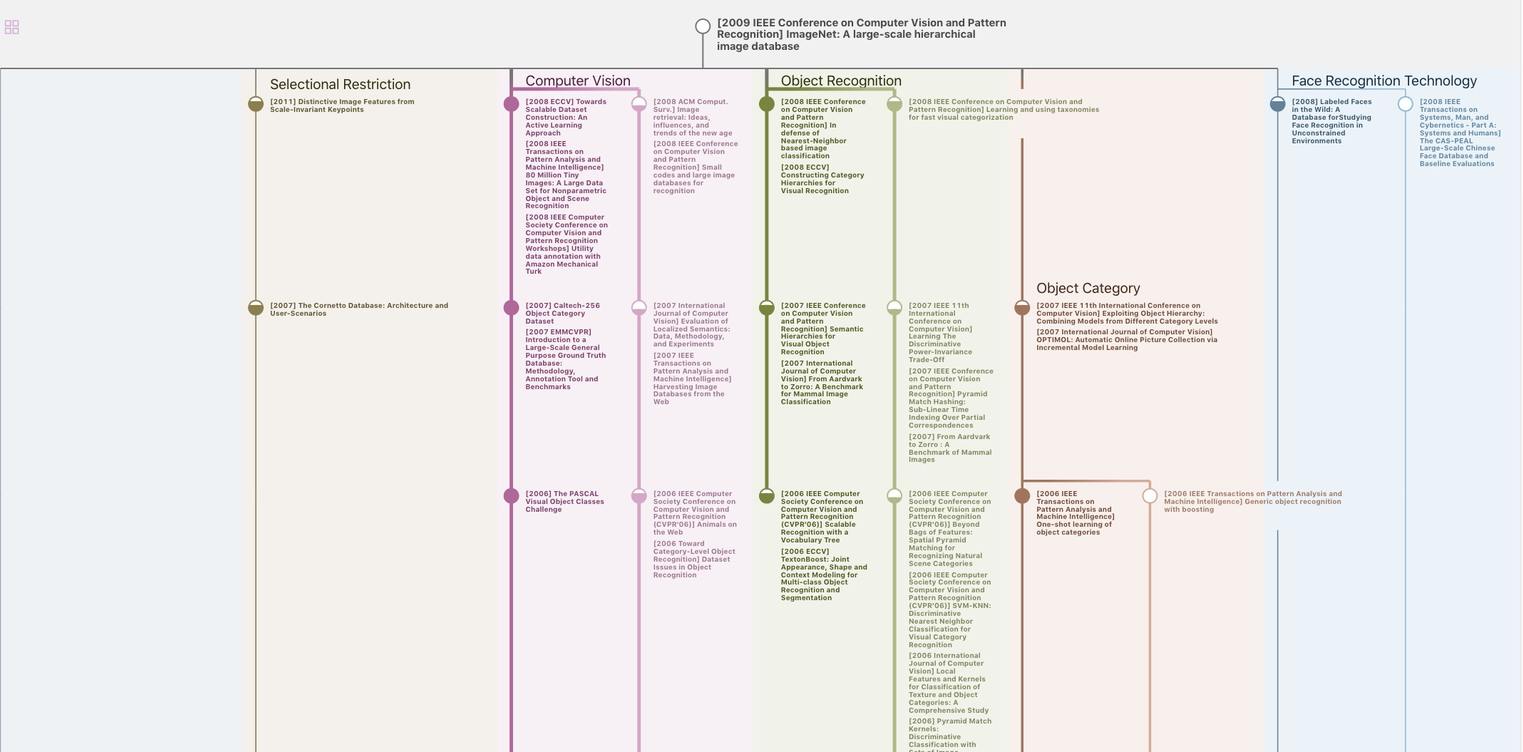
生成溯源树,研究论文发展脉络
Chat Paper
正在生成论文摘要