TMP-SSurface: A Deep Learning-Based Predictor for Surface Accessibility of Transmembrane Protein Residues
CRYSTALS(2019)
摘要
Transmembrane proteins (TMPs) play vital and diverse roles in many biological processes, such as molecular transportation and immune response. Like other proteins, many major interactions with other molecules happen in TMPs' surface area, which is important for function annotation and drug discovery. Under the condition that the structure of TMP is hard to derive from experiment and prediction, it is a practical way to predict the TMP residues' surface area, measured by the relative accessible surface area (rASA), based on computational methods. In this study, we presented a novel deep learning-based predictor TMP-SSurface for both alpha-helical and beta-barrel transmembrane proteins (alpha-TMP and beta-TMP), where convolutional neural network (CNN), inception blocks, and CapsuleNet were combined to construct a network framework, simply accepting one-hot code and position-specific score matrix (PSSM) of protein fragment as inputs. TMP-SSurface was tested against an independent dataset achieving appreciable performance with 0.584 Pearson correlation coefficients (CC) value. As the first TMP's rASA predictor utilizing the deep neural network, our method provided a referenceable sample for the community, as well as a practical step to discover the interaction sites of TMPs based on their sequence.
更多查看译文
关键词
transmembrane protein,surface accessibility,deep learning
AI 理解论文
溯源树
样例
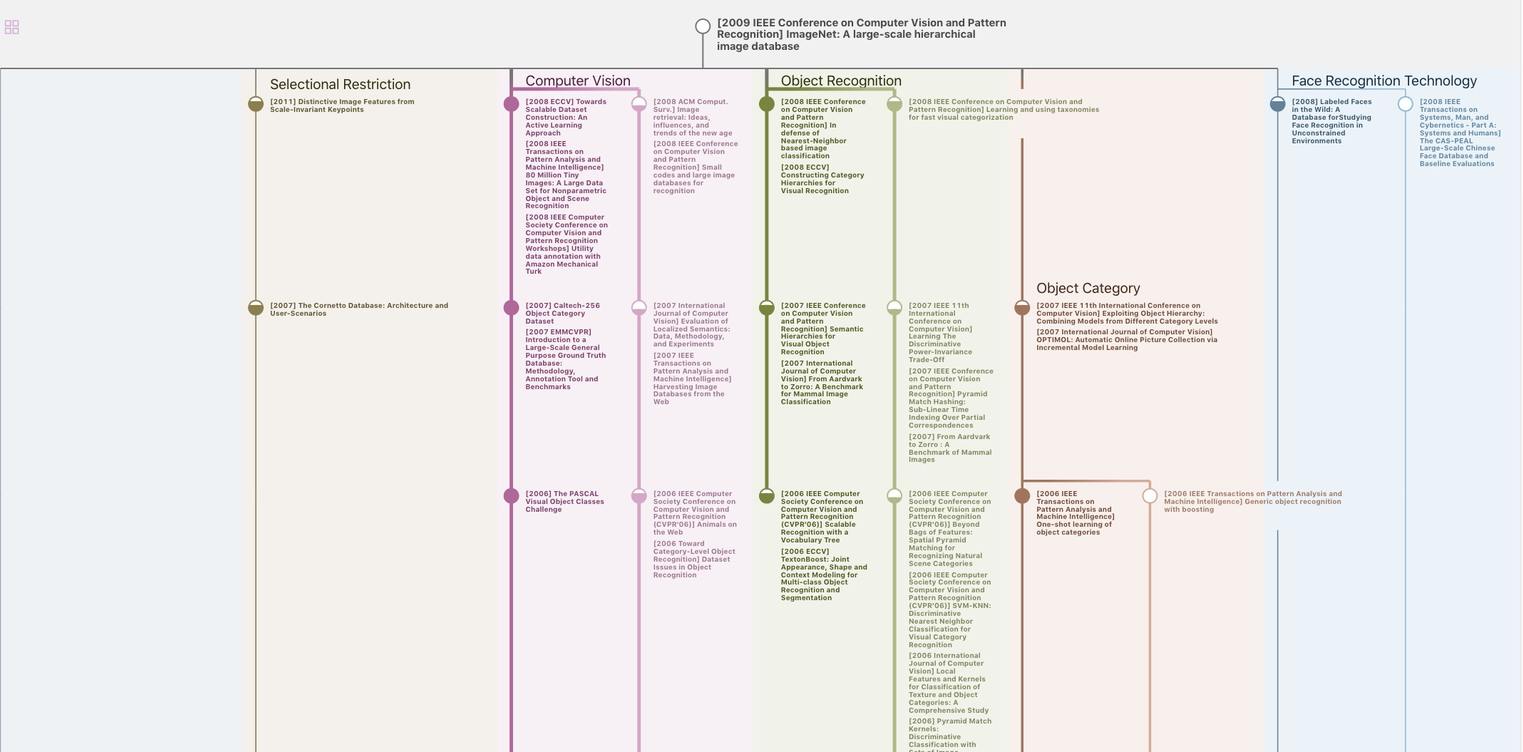
生成溯源树,研究论文发展脉络
Chat Paper
正在生成论文摘要