Privacy and Robustness in Federated Learning: Attacks and Defenses.
IEEE transactions on neural networks and learning systems(2022)
摘要
As data are increasingly being stored in different silos and societies becoming more aware of data privacy issues, the traditional centralized training of artificial intelligence (AI) models is facing efficiency and privacy challenges. Recently, federated learning (FL) has emerged as an alternative solution and continues to thrive in this new reality. Existing FL protocol designs have been shown to be vulnerable to adversaries within or outside of the system, compromising data privacy and system robustness. Besides training powerful global models, it is of paramount importance to design FL systems that have privacy guarantees and are resistant to different types of adversaries. In this article, we conduct a comprehensive survey on privacy and robustness in FL over the past five years. Through a concise introduction to the concept of FL and a unique taxonomy covering: 1) threat models; 2) privacy attacks and defenses; and 3) poisoning attacks and defenses, we provide an accessible review of this important topic. We highlight the intuitions, key techniques, and fundamental assumptions adopted by various attacks and defenses. Finally, we discuss promising future research directions toward robust and privacy-preserving FL, and their interplays with the multidisciplinary goals of FL.
更多查看译文
关键词
Attacks,defenses,federated learning (FL),privacy,robustness
AI 理解论文
溯源树
样例
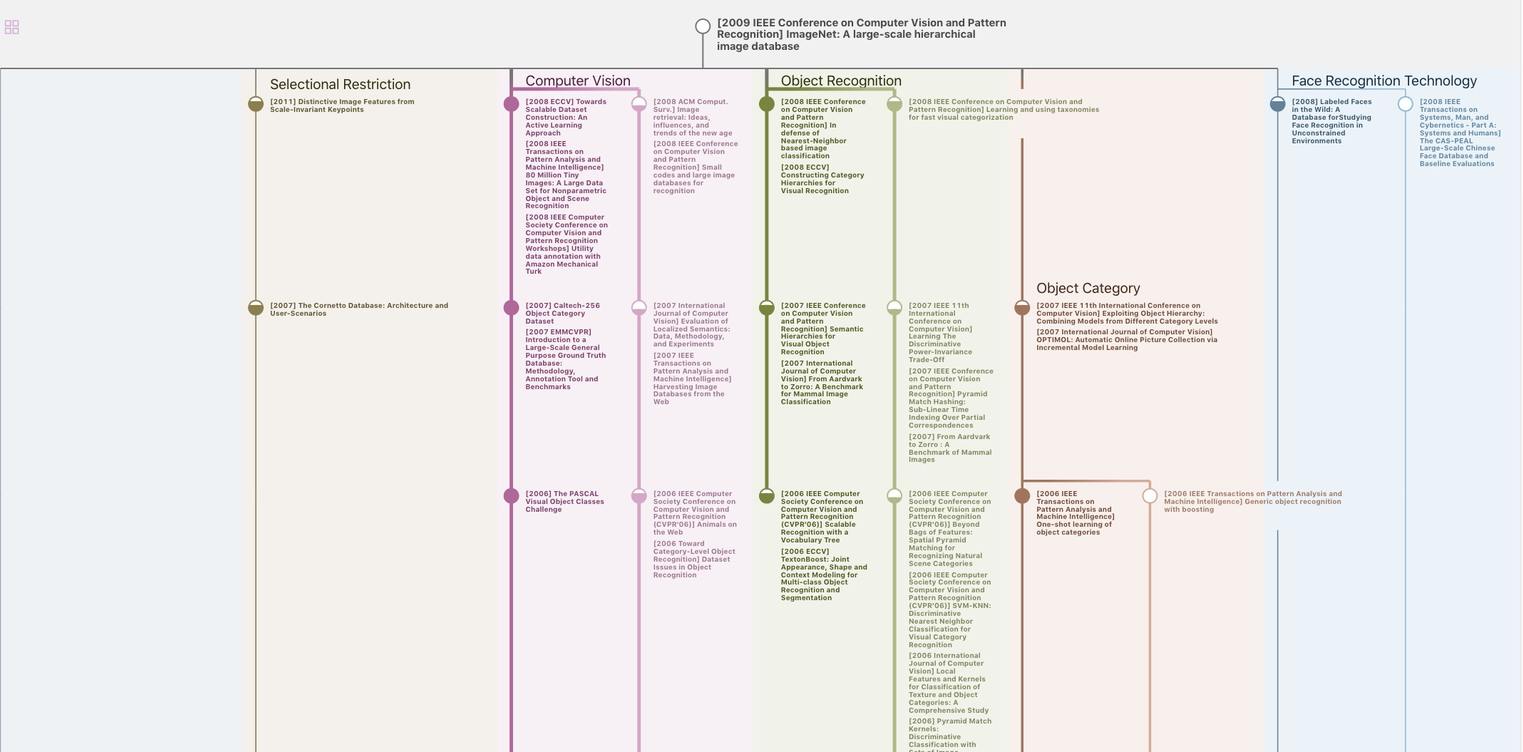
生成溯源树,研究论文发展脉络
Chat Paper
正在生成论文摘要