LayoutGMN: Neural Graph Matching for Structural Layout Similarity
2021 IEEE/CVF CONFERENCE ON COMPUTER VISION AND PATTERN RECOGNITION, CVPR 2021(2021)
摘要
We present a deep neural network to predict structural similarity between 2D layouts by leveraging Graph Matching Networks (GMN). Our network, coined LayoutGMN, learns the layout metric via neural graph matching, using an attention-based GMN designed under a triplet network setting. To train our network, we utilize weak labels obtained by pixel-wise Intersection-over-Union (IoUs) to define the triplet loss. Importantly, LayoutGMN is built with a structural bias which can effectively compensate for the lack of structure awareness in IoUs. We demonstrate this on two prominent forms of layouts, viz., floorplans and UI designs, via retrieval experiments on large-scale datasets. In particular, retrieval results by our network better match human judgement of structural layout similarity compared to both IoUs and other baselines including a state-of-theart method based on graph neural networks and image convolution. In addition, LayoutGMN is the first deep model to offer both metric learning of structural layout similarity and structural matching between layout elements.
更多查看译文
关键词
IoUs,structure awareness,match human judgement,structural layout similarity,graph neural networks,image convolution,structural matching,layout elements,neural graph matching,deep neural network,structural similarity,graph matching networks,coined LayoutGMN,layout metric,attention-based GMN,triplet network setting,pixel-wise intersection-over-union
AI 理解论文
溯源树
样例
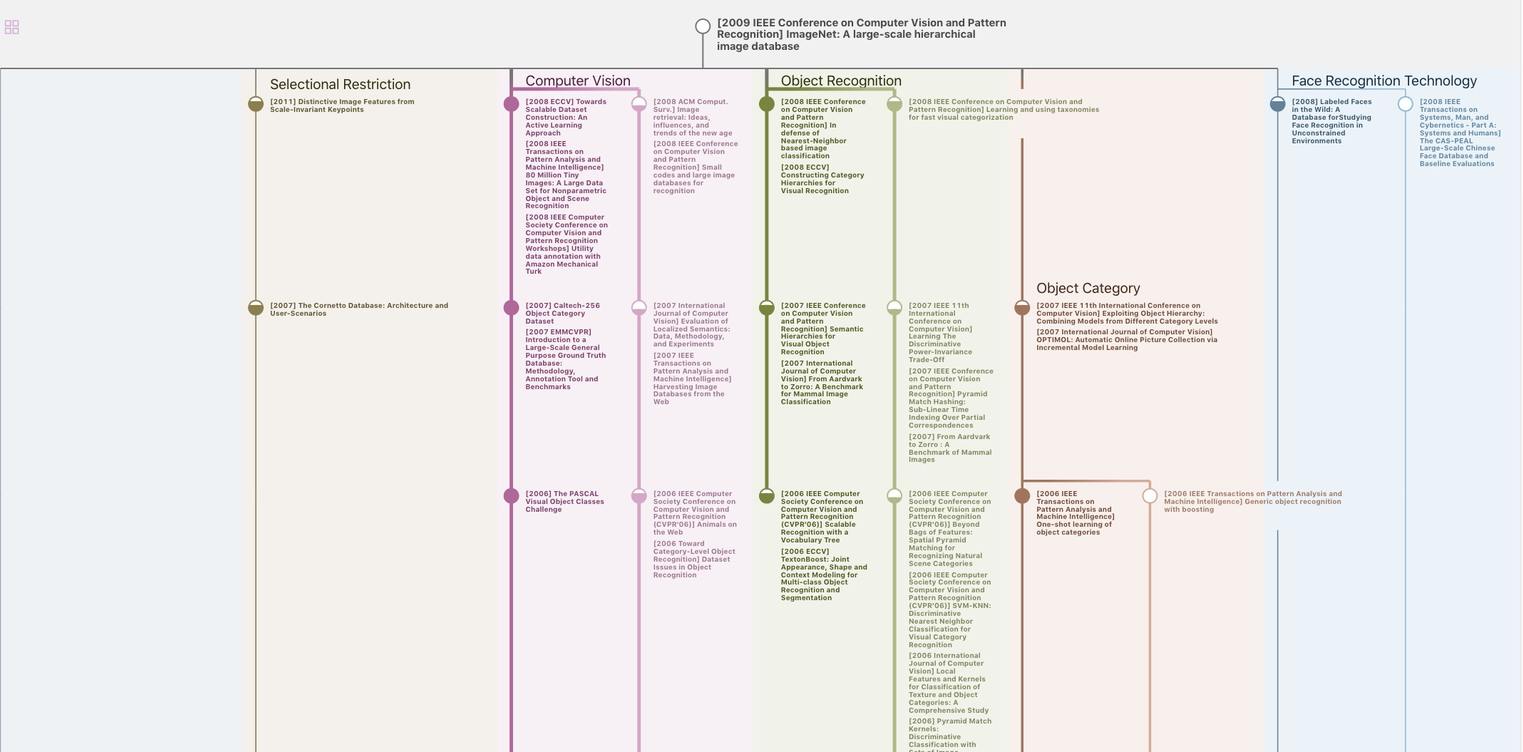
生成溯源树,研究论文发展脉络
Chat Paper
正在生成论文摘要