Delay Differential Neural Networks
PROCEEDINGS OF 2021 6TH INTERNATIONAL CONFERENCE ON MACHINE LEARNING TECHNOLOGIES (ICMLT 2021)(2021)
摘要
Neural ordinary differential equations (NODEs) treat computation of intermediate feature vectors as trajectories of ordinary differential equation parameterized by a neural network. In this paper, we propose a novel model, delay differential neural networks (DDNN), inspired by delay differential equations (DDEs). The proposed model considers the derivative of the hidden feature vector as a function of the current feature vector and past feature vectors (history) unlike only the current feature vector in the case of NODE. The function is modelled as a neural network and consequently, it leads to continuous depth alternatives to recent ResNet variants. For training DDNNs, we discuss a memory-efficient adjoint method for computing gradients and back-propagate through the network. DDNN improves the data efficiency of NODE by further reducing the number of parameters without affecting the generalization performance. Experiments conducted on real-world image classification datasets such as cifar10 and cifar100 to show the effectiveness of the proposed model.
更多查看译文
关键词
Delay differential equations, Deep learning, Adjoint method
AI 理解论文
溯源树
样例
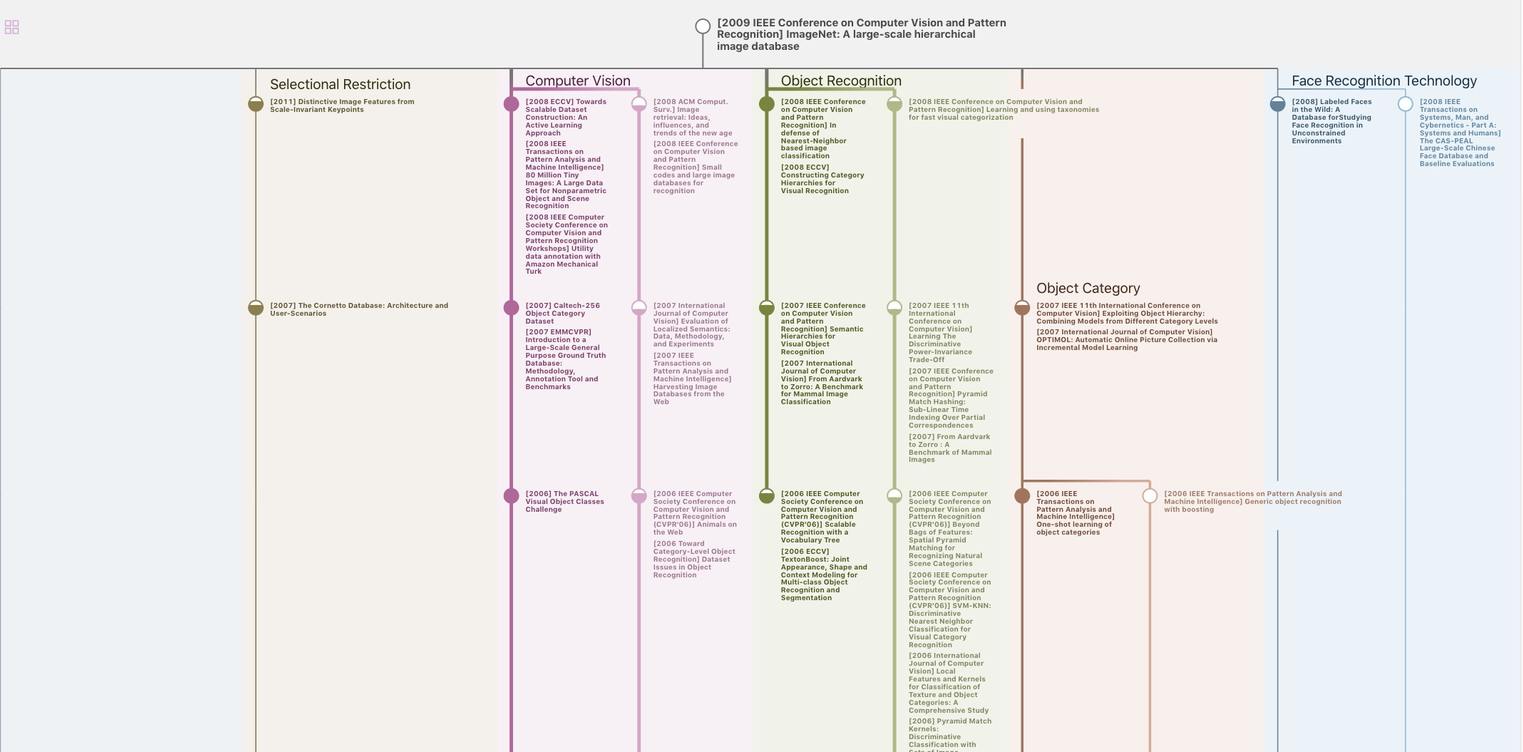
生成溯源树,研究论文发展脉络
Chat Paper
正在生成论文摘要