Bandit Learning in Decentralized Matching Markets
JOURNAL OF MACHINE LEARNING RESEARCH(2021)
摘要
We study two-sided matching markets in which one side of the market (the players) does not have a priori knowledge about its preferences for the other side (the arms) and is required to learn its preferences from experience. Also, we assume the players have no direct means of communication. This model extends the standard stochastic multi-armed bandit framework to a decentralized multiple player setting with competition. We introduce a new algorithm for this setting that, over a time horizon T, attains O(log(T)) stable regret when preferences of the arms over players are shared, and O(log(T)(2)) regret when there are no assumptions on the preferences on either side. Moreover, in the setting where a single player may deviate, we show that the algorithm is incentive compatible whenever the arms' preferences are shared, but not necessarily so when preferences are fully general.
更多查看译文
关键词
Online learning, Multi-armed bandits, Stable matching, Two-sided markets
AI 理解论文
溯源树
样例
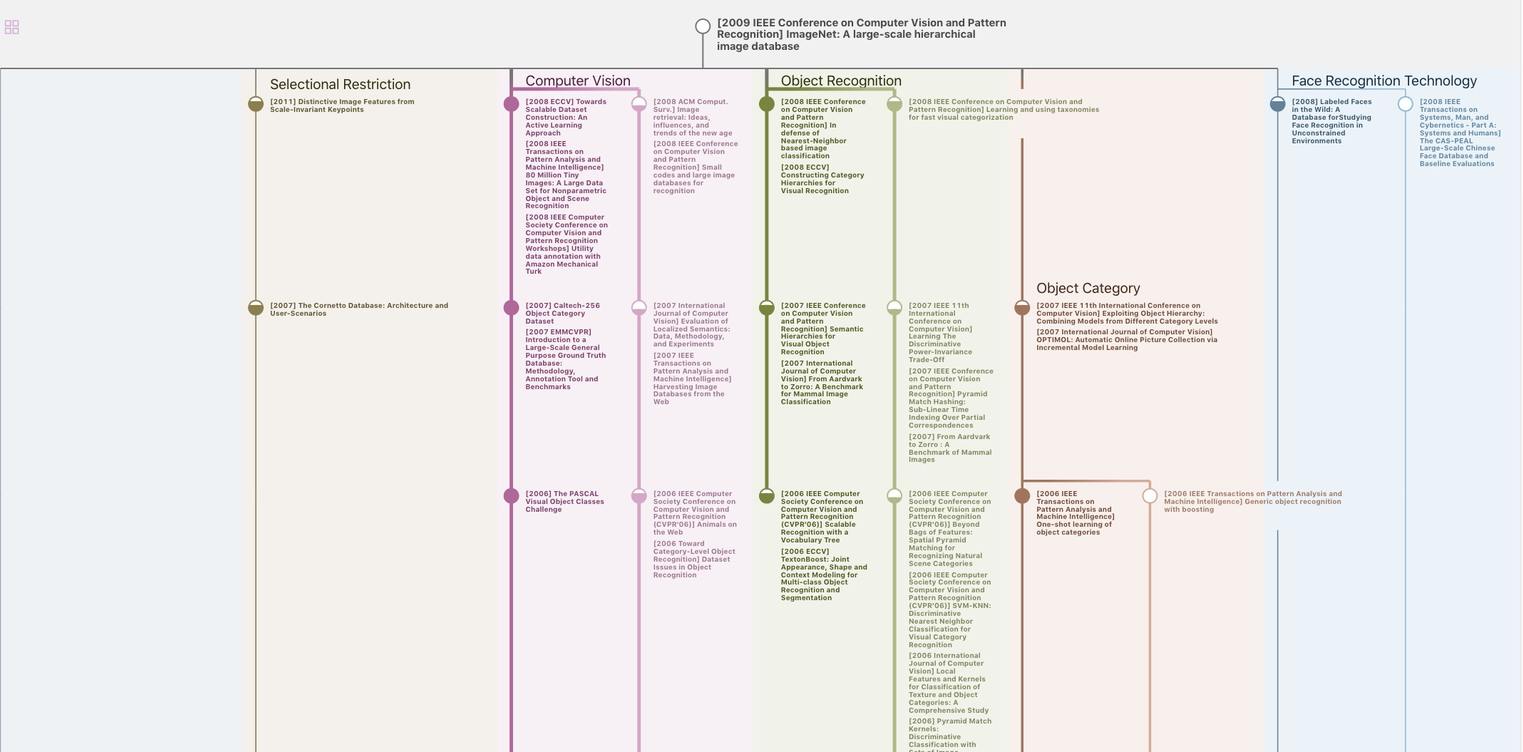
生成溯源树,研究论文发展脉络
Chat Paper
正在生成论文摘要