Improved Protein Model Quality Assessment By Integrating Sequential And Pairwise Features Using Deep Learning
BIOINFORMATICS(2021)
摘要
Motivation: Accurately estimating protein model quality in the absence of experimental structure is not only important for model evaluation and selection but also useful for model refinement. Progress has been steadily made by introducing new features and algorithms (especially deep neural networks), but the accuracy of quality assessment (QA) is still not very satisfactory, especially local QA on hard protein targets.Results: We propose a new single-model-based QA method ResNetQA for both local and global quality assessment. Our method predicts model quality by integrating sequential and pairwise features using a deep neural network composed of both 1D and 2D convolutional residual neural networks (ResNet). The 2D ResNet module extracts useful information from pairwise features such as model-derived distance maps, co-evolution information, and predicted distance potential from sequences. The 1D ResNet is used to predict local (global) model quality from sequential features and pooled pairwise information generated by 2D ResNet. Tested on the CASP12 and CASP13 datasets, our experimental results show that our method greatly outperforms existing state-of-the-art methods. Our ablation studies indicate that the 2D ResNet module and pairwise features play an important role in improving model quality assessment.
更多查看译文
关键词
protein,deep learning,pairwise features,quality
AI 理解论文
溯源树
样例
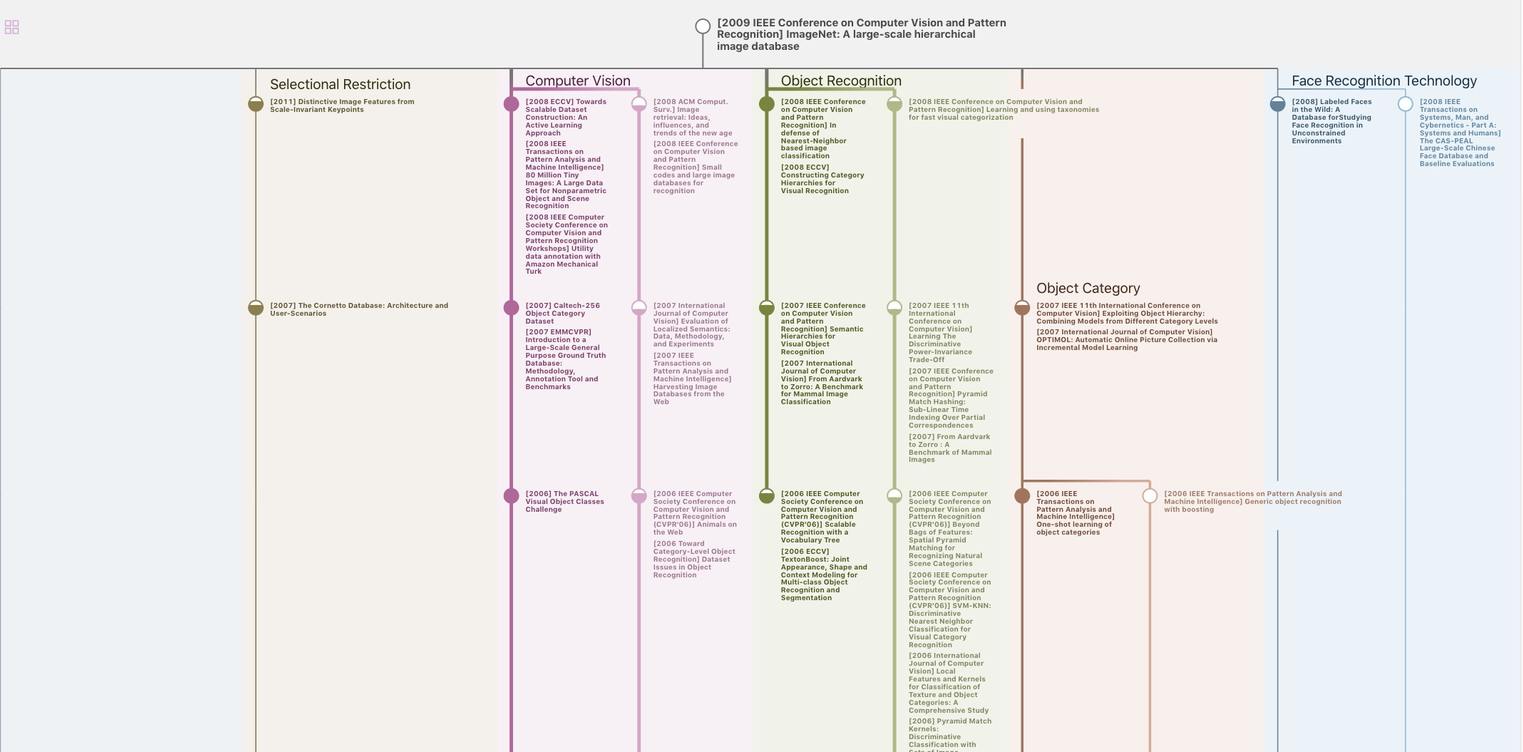
生成溯源树,研究论文发展脉络
Chat Paper
正在生成论文摘要