MiniRocket: A Very Fast (Almost) Deterministic Transform for Time Series Classification
Knowledge Discovery and Data Mining(2021)
摘要
ABSTRACTRocket achieves state-of-the-art accuracy for time series classification with a fraction of the computational expense of most existing methods by transforming input time series using random convolutional kernels, and using the transformed features to train a linear classifier. We reformulate Rocket into a new method, MiniRocket. MiniRocket is up to 75 times faster than Rocket on larger datasets, and almost deterministic (and optionally, fully deterministic), while maintaining essentially the same accuracy. Using this method, it is possible to train and test a classifier on all of 109 datasets from the UCR archive to state-of-the-art accuracy in under 10 minutes. MiniRocket is significantly faster than any other method of comparable accuracy (including Rocket), and significantly more accurate than any other method of remotely similar computational expense.
更多查看译文
关键词
scalable, time series classification, convolution, transform
AI 理解论文
溯源树
样例
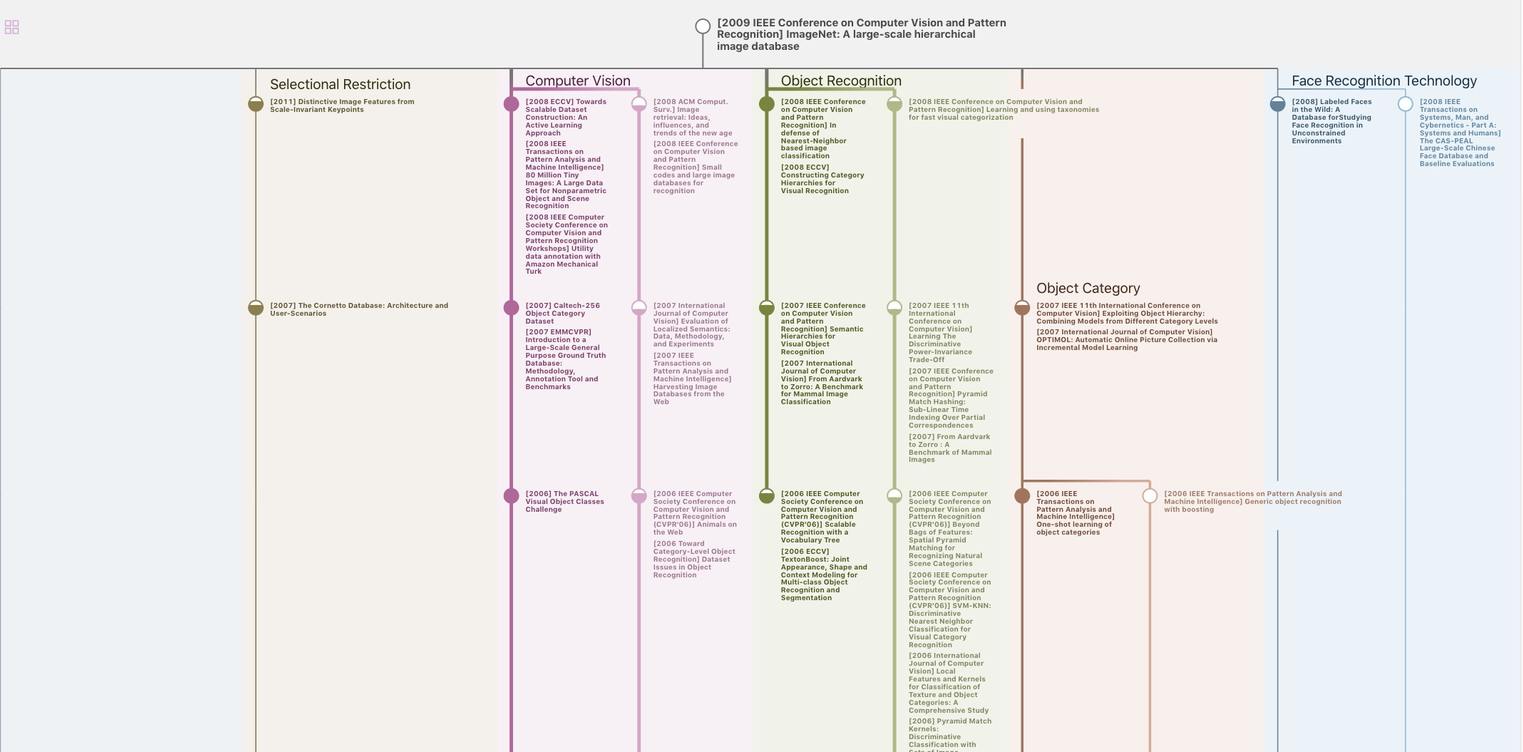
生成溯源树,研究论文发展脉络
Chat Paper
正在生成论文摘要