Spatial Context-Aware Self-Attention Model For Multi-Organ Segmentation
2021 IEEE WINTER CONFERENCE ON APPLICATIONS OF COMPUTER VISION (WACV 2021)(2021)
摘要
Multi-organ segmentation is one of most successful applications of deep learning in medical image analysis. Deep convolutional neural nets (CNNs) have shown great promise in achieving clinically applicable image segmentation performance on CT or MRI images. State-of-the-art CNN segmentation models apply either 2D or 3D convolutions on input images, with pros and cons associated with each method: 2D convolution is fast, less memory-intensive but inadequate for extracting 3D contextual information from volumetric images, while the opposite is true for 3D convolution. To fit a 3D CNN model on CT or MRI images on commodity GPUs, one usually has to either down-sample input images or use cropped local regions as inputs, which limits the utility of 3D models for multi-organ segmentation. In this work, we propose a new framework for combining 3D and 2D models, in which the segmentation is realized through high-resolution 2D convolutions, but guided by spatial contextual information extracted from a low-resolution 3D model. We implement a self-attention mechanism to control which 3D features should be used to guide 2D segmentation. Our model is light on memory usage but fully equipped to take 3D contextual information into account. Experiments on multiple organ segmentation datasets demonstrate that by taking advantage of both 2D and 3D models, our method is consistently outperforms existing 2D and 3D models in organ segmentation accuracy, while being able to directly take raw whole-volume image data as inputs.
更多查看译文
关键词
convolutional neural nets,clinically applicable image segmentation performance,state-of-the-art CNN segmentation models,extracting 3D contextual information,volumetric images,3D CNN model,downsample input images,multiorgan segmentation,high-resolution 2D convolutions,spatial contextual information,low-resolution 3D,multiple organ segmentation datasets,organ segmentation accuracy,raw whole-volume image data,spatial context-aware self,medical image analysis
AI 理解论文
溯源树
样例
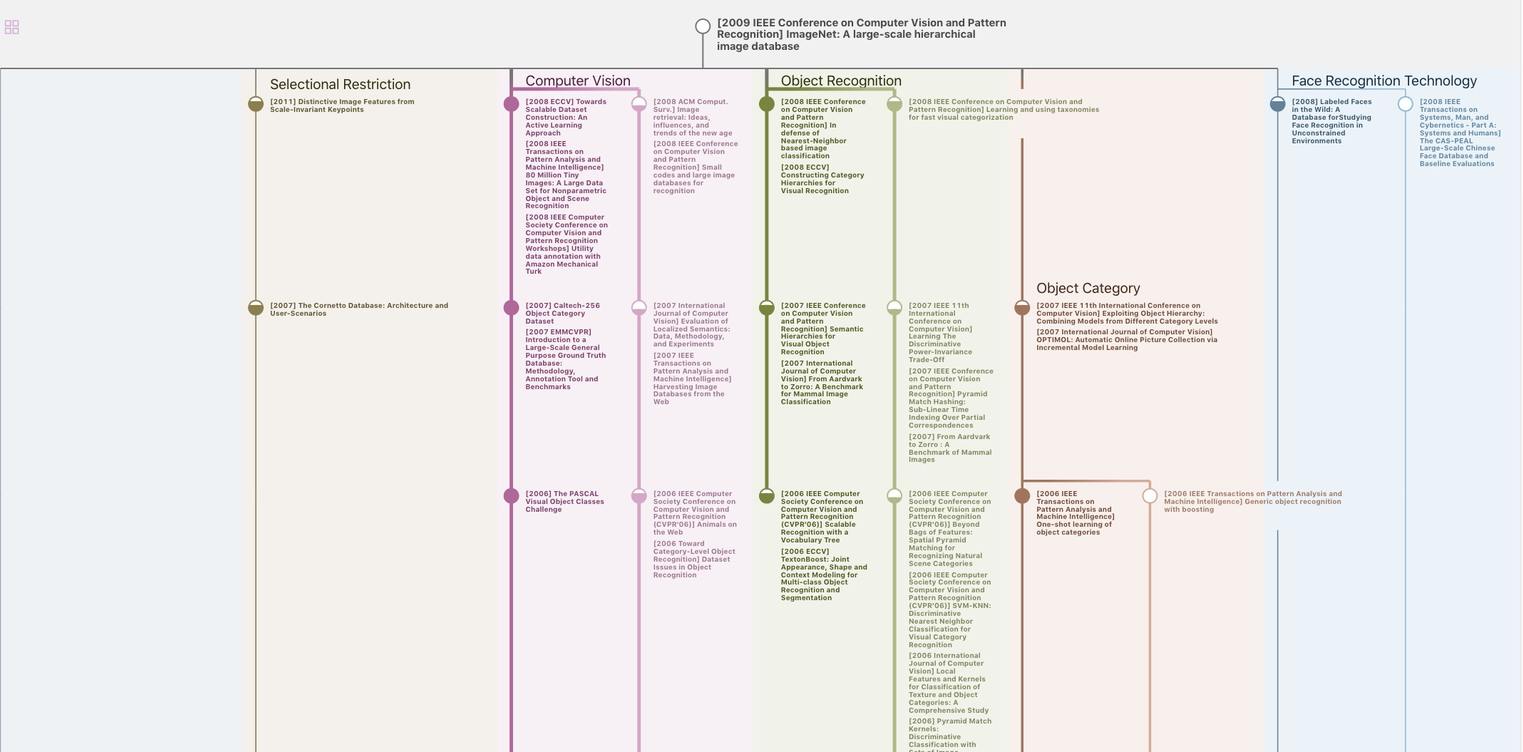
生成溯源树,研究论文发展脉络
Chat Paper
正在生成论文摘要