A Data-Driven Approach for the Health Prognosis of High-Speed Train Wheels
PROCEEDINGS OF THE INSTITUTION OF MECHANICAL ENGINEERS PART O-JOURNAL OF RISK AND RELIABILITY(2020)
摘要
Polygonal wear is one of the most critical failure modes of high-speed train wheels that would significantly compromise the safety and reliability of high-speed train operation. However, the mechanism underpinning wheel polygon is complex and still not fully understood, which makes it challenging to track its evolution of the polygonal wheel. The large amount of data gathered through regular inspection and maintenance of Chinese high-speed trains provides a promising way to tackle this challenge with data-driven methods. This article proposes a data-driven approach to predict the degree of the polygonal wear, assess the reliability of individual wheels and the health index of all wheels of a high-speed train for maintenance priority ranking. The synthetic minority over-sampling technique-nominal continuous is adopted to augment the maintenance dataset of imbalanced and mixed features. The autoencoder is used to learn abstract features to represent the original datasets, which are then fed into a support vector machine classifier. The approach is coherently optimized by tuning the model hyper-parameters based on Bayesian optimization. The effectiveness of our proposed approach is demonstrated by the wheel maintenance data obtained from the year 2016 to 2017. The results can also be used to support practical maintenance priority allocation.
更多查看译文
关键词
Polygonal wear,high-speed train,data-driven,synthetic minority over-sampling technique-nominal continuous,autoencoder,Bayesian optimization
AI 理解论文
溯源树
样例
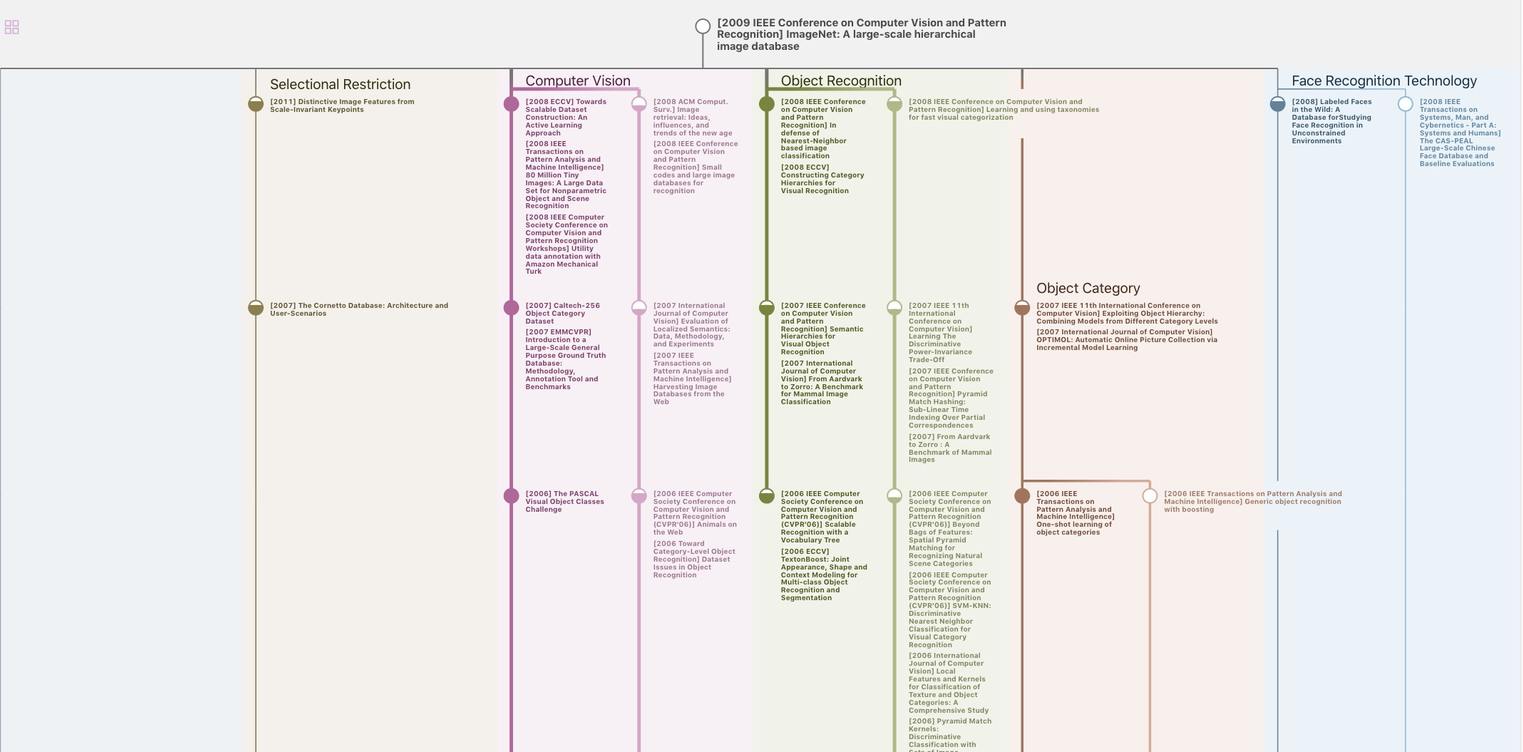
生成溯源树,研究论文发展脉络
Chat Paper
正在生成论文摘要