Automatic Liver Tumor Segmentation on Multiphase Computed Tomography Volume Using SegNet Deep Neural Network and K-means Clustering.
International journal of imaging systems and technology(2022)
摘要
Liver and liver tumor segmentations are essential in computer-aided systems for diagnosing liver tumors. These systems must operate on multiphase computed tomography (CT) images instead of a single phase for accurate diagnosis for clinical applications. We have proposed a framework that can perform segmentation from quadriphasic CT data. The liver was segmented using a fine-tuned SegNet model and the liver tumor was segmented using the K-means clustering method coupled with a power-law transformation-based image enhancement technique. The best values for liver segmentation achieved were: Dice Coefficient = 96.46 +/- 0.48%, Jaccard Index = 93.16 +/- 0.89%, volumetric overlap error = 6.84 +/- 0.89% and average symmetric surface distance = 0.59 +/- 0.3 mm and the results for liver tumor delineation were Dice Coefficient = 85.07 +/- 4.5%, Jaccard Index = 74.29 +/- 6.8%, volumetric overlap error = 25.71 +/- 6.8% and average symmetric surface distance = 1.14 +/- 1.3 mm. The proposed liver segmentation method based on deep learning is fully automatic, robust, and effective for all phases. The image enhancement technique has shown promising results and aided in better liver tumor segmentation. The liver tumors were segmented satisfactorily; however, improvements concerning false positive reduction can further increase the accuracy.
更多查看译文
关键词
computed tomography,contrast enhancement,K-means clustering,liver tumor segmentation,power-law transformation,SegNet,semantic segmentation
AI 理解论文
溯源树
样例
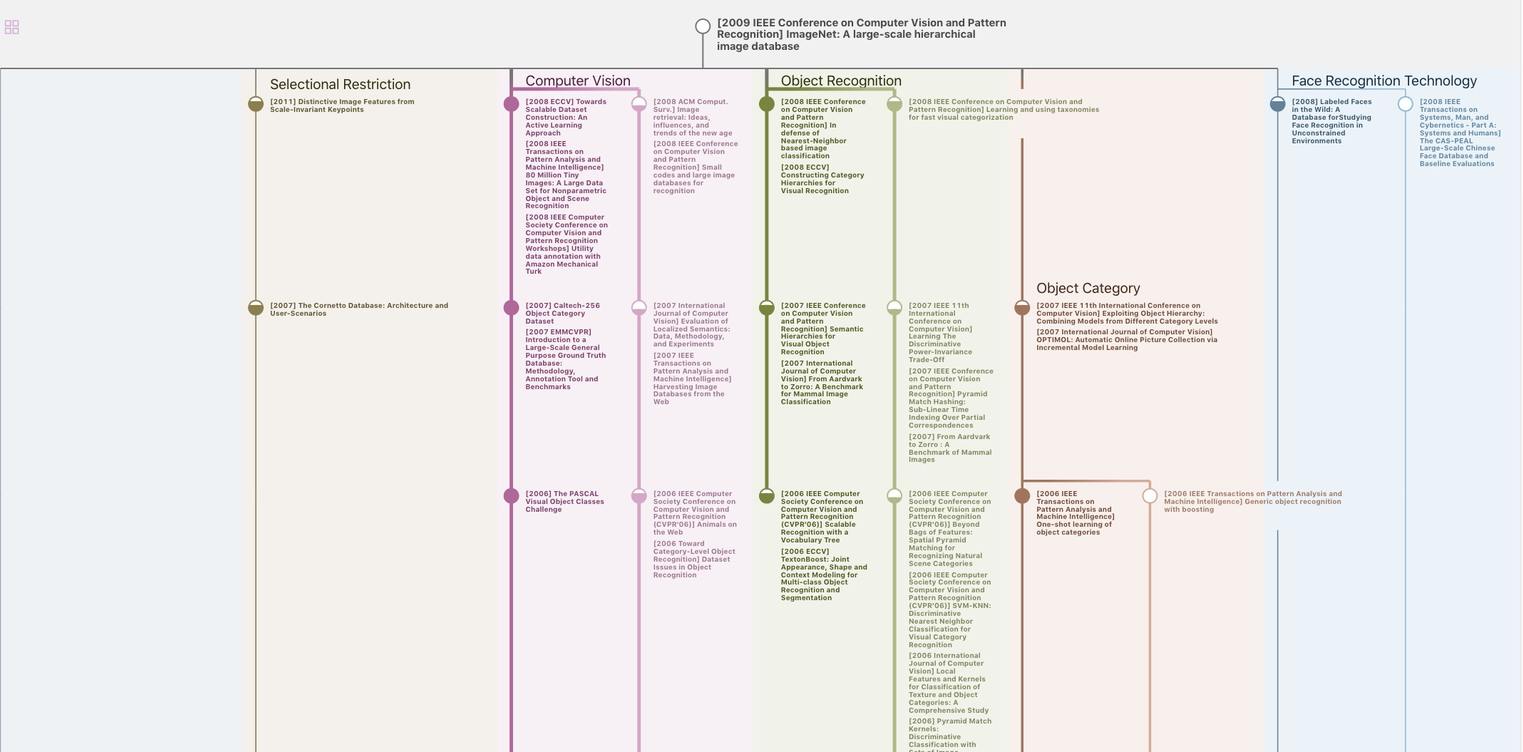
生成溯源树,研究论文发展脉络
Chat Paper
正在生成论文摘要