Improving clinical disease subtyping and future events prediction through a chest CT-based deep learning approach.
Medical physics(2021)
摘要
PURPOSE:To develop and evaluate a deep learning (DL) approach to extract rich information from high-resolution computed tomography (HRCT) of patients with chronic obstructive pulmonary disease (COPD).
METHODS:We develop a DL-based model to learn a compact representation of a subject, which is predictive of COPD physiologic severity and other outcomes. Our DL model learned: (a) to extract informative regional image features from HRCT; (b) to adaptively weight these features and form an aggregate patient representation; and finally, (c) to predict several COPD outcomes. The adaptive weights correspond to the regional lung contribution to the disease. We evaluate the model on 10 300 participants from the COPDGene cohort.
RESULTS:Our model was strongly predictive of spirometric obstruction ( r 2 = 0.67) and grouped 65.4% of subjects correctly and 89.1% within one stage of their GOLD severity stage. Our model achieved an accuracy of 41.7% and 52.8% in stratifying the population-based on centrilobular (5-grade) and paraseptal (3-grade) emphysema severity score, respectively. For predicting future exacerbation, combining subjects' representations from our model with their past exacerbation histories achieved an accuracy of 80.8% (area under the ROC curve of 0.73). For all-cause mortality, in Cox regression analysis, we outperformed the BODE index improving the concordance metric (ours: 0.61 vs BODE: 0.56).
CONCLUSIONS:Our model independently predicted spirometric obstruction, emphysema severity, exacerbation risk, and mortality from CT imaging alone. This method has potential applicability in both research and clinical practice.
更多查看译文
AI 理解论文
溯源树
样例
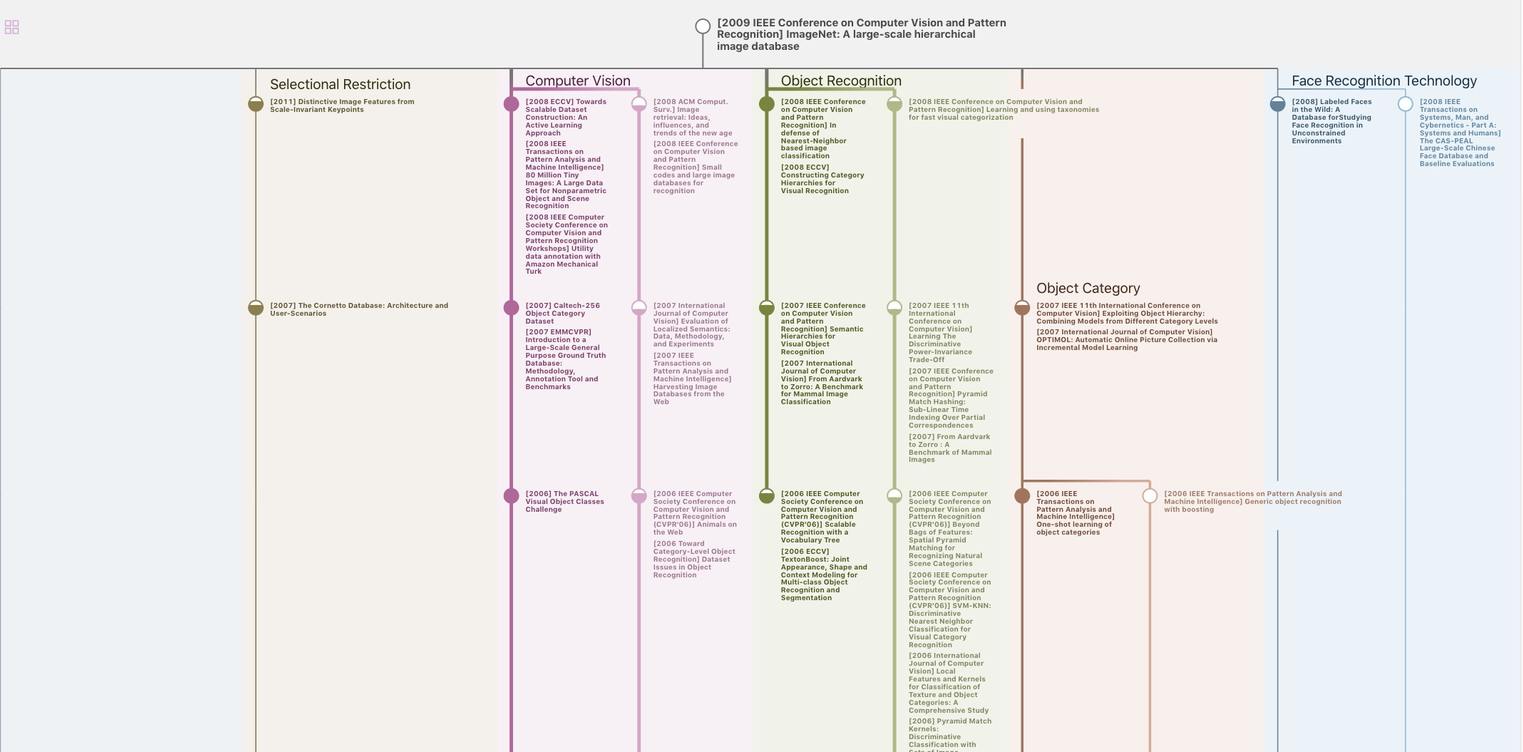
生成溯源树,研究论文发展脉络
Chat Paper
正在生成论文摘要