Information-Preserving Contrastive Learning for Self-Supervised Representations
arxiv(2021)
摘要
Contrastive learning is very effective at learning useful representations without supervision. Yet contrastive learning has its limitations. It can learn a shortcut that is irrelevant to the downstream task, and discard relevant information. Past work has addressed this limitation via custom data augmentations that eliminate the shortcut. This solution however does not work for data modalities that are not interpretable by humans, e.g., radio signals. For such modalities, it is hard for a human to guess which shortcuts may exist in the signal, or how to alter the radio signals to eliminate the shortcuts. Even for visual data, sometimes eliminating the shortcut may be undesirable. The shortcut may be irrelevant to one downstream task but important to another. In this case, it is desirable to learn a representation that captures both the shortcut information and the information relevant to the other downstream task. This paper presents information-preserving contrastive learning (IPCL), a new framework for unsupervised representation learning that preserves relevant information even in the presence of shortcuts. We empirically show that IPCL addresses the above problems and outperforms contrastive learning on radio signals and learning RGB data representation with different features that support different downstream tasks.
更多查看译文
关键词
feature suppression,representations,visual
AI 理解论文
溯源树
样例
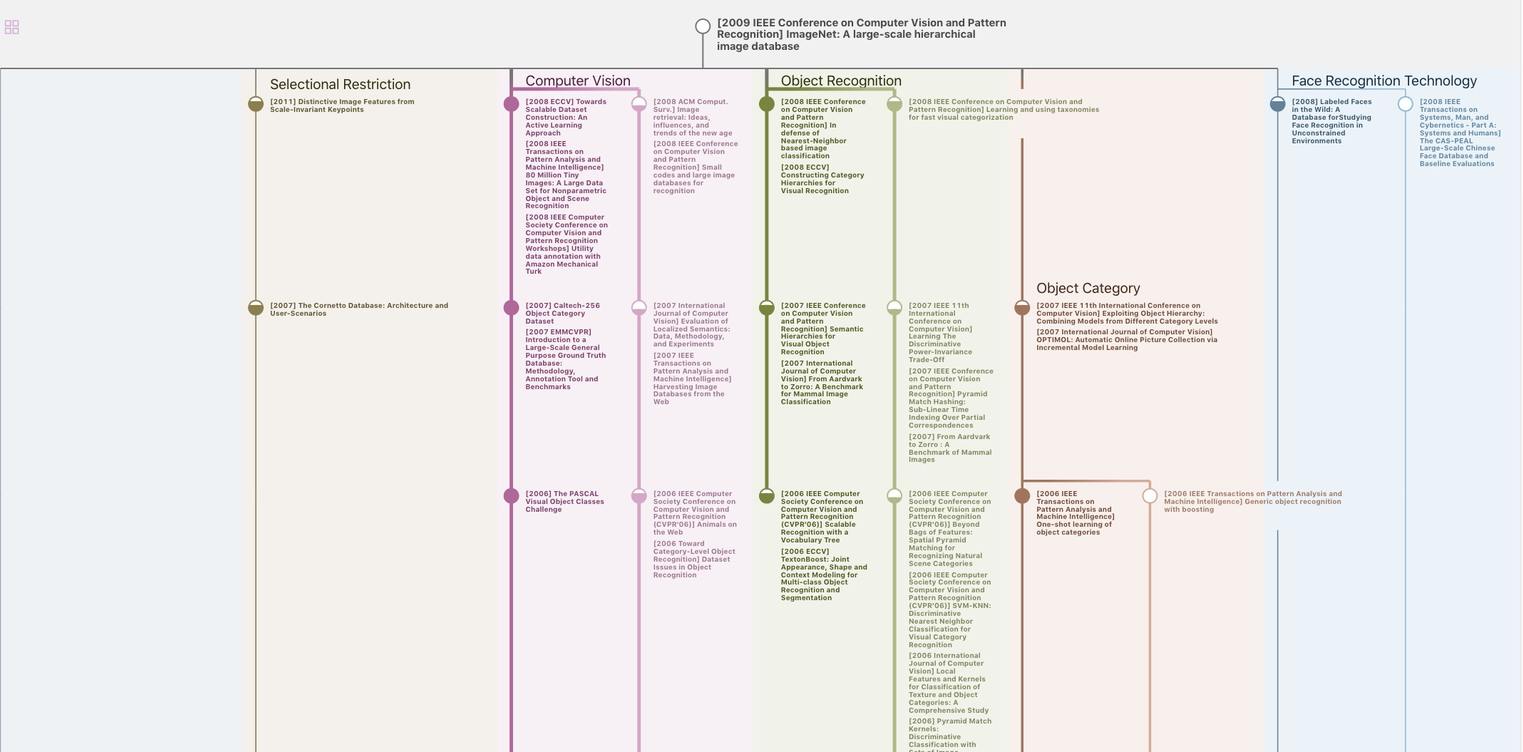
生成溯源树,研究论文发展脉络
Chat Paper
正在生成论文摘要