Regularized estimation in high-dimensional vector auto-regressive models using spatio-temporal information
STATISTICA SINICA(2023)
摘要
The vector auto-regressive (VAR) model is commonly used to model multivariate time series, and there are many penalized methods to handle high dimensionality. However for spatio-temporal data, most of these methods do not consider the spatial and temporal structure of the data, which may lead to unreliable network detection and inaccurate forecasts. This paper proposes a data-driven weighted l1 regularized approach for spatio-temporal VAR models. Extensive simulation studies compare the proposed method with five existing methods for high-dimensional VAR models, demonstrating advantages of our method over others in terms of parameter estimation, network detection, and out-of-sample forecasts. We also apply our method to a traffic data set to evaluate its performance in a real application. In addition, we explore the theoretical properties of the l1 regularized estimation of the VAR model under a weakly sparse scenario, in which exact sparsity can be viewed as a special case. To the best of our knowledge, this is the first study to do so. For a general stationary VAR process, we derive the nonasymptotic upper bounds on the l1 regularized estimation errors, provide the conditions for estimation consistency, and further simplify these conditions for a special VAR(1) case.
更多查看译文
关键词
l1 regularization,spatio-temporal structure,vector auto-regressive model,weak sparsity
AI 理解论文
溯源树
样例
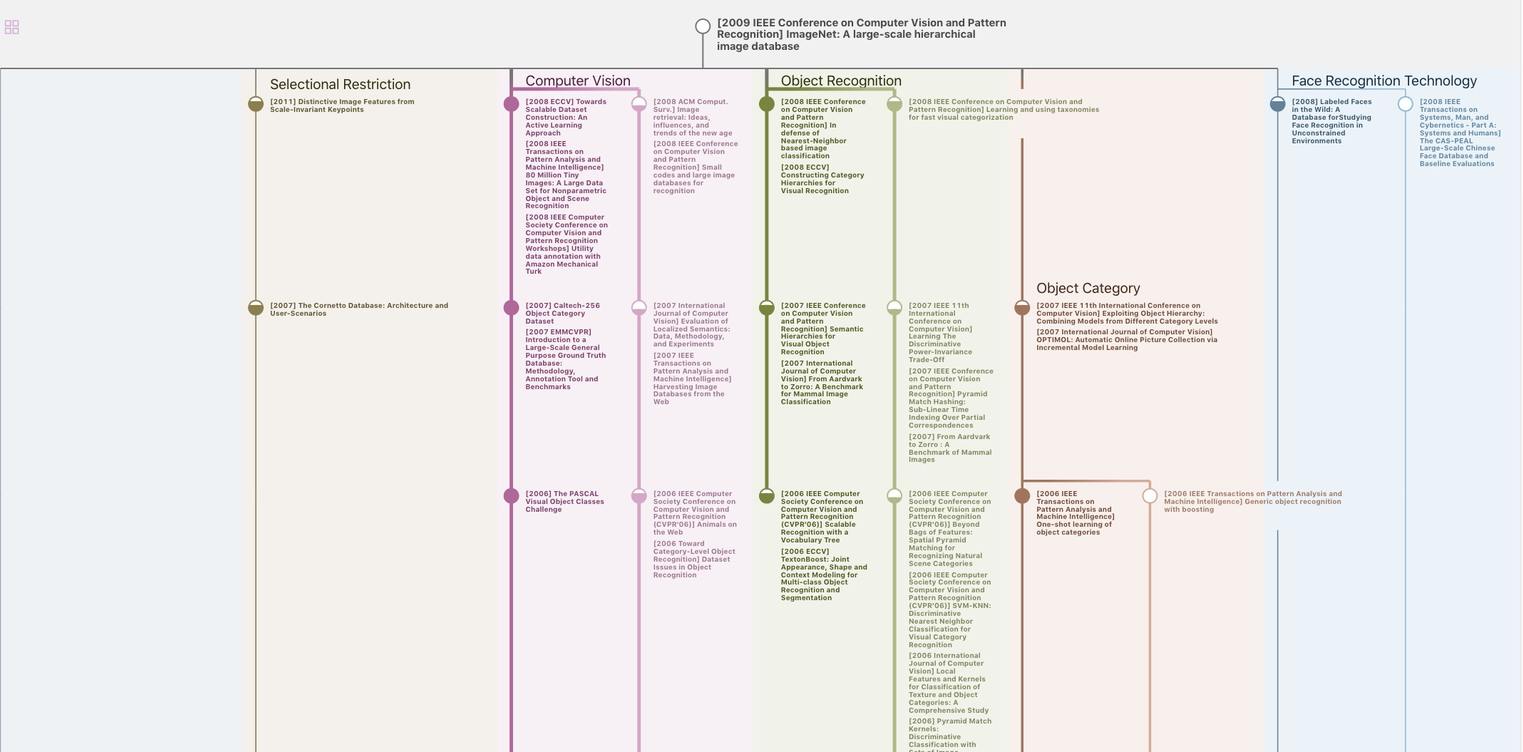
生成溯源树,研究论文发展脉络
Chat Paper
正在生成论文摘要