Stable Implementation of Probabilistic ODE Solvers
arxiv(2020)
摘要
Probabilistic solvers for ordinary differential equations (ODEs) provide efficient quantification of numerical uncertainty associated with simulation of dynamical systems. Their convergence rates have been established by a growing body of theoretical analysis. However, these algorithms suffer from numerical instability when run at high order or with small step-sizes -- that is, exactly in the regime in which they achieve the highest accuracy. The present work proposes and examines a solution to this problem. It involves three components: accurate initialisation, a coordinate change preconditioner that makes numerical stability concerns step-size-independent, and square-root implementation. Using all three techniques enables numerical computation of probabilistic solutions of ODEs with algorithms of order up to 11, as demonstrated on a set of challenging test problems. The resulting rapid convergence is shown to be competitive to high-order, state-of-the-art, classical methods. As a consequence, a barrier between analysing probabilistic ODE solvers and applying them to interesting machine learning problems is effectively removed.
更多查看译文
关键词
probabilistic ode solvers,stable implementation
AI 理解论文
溯源树
样例
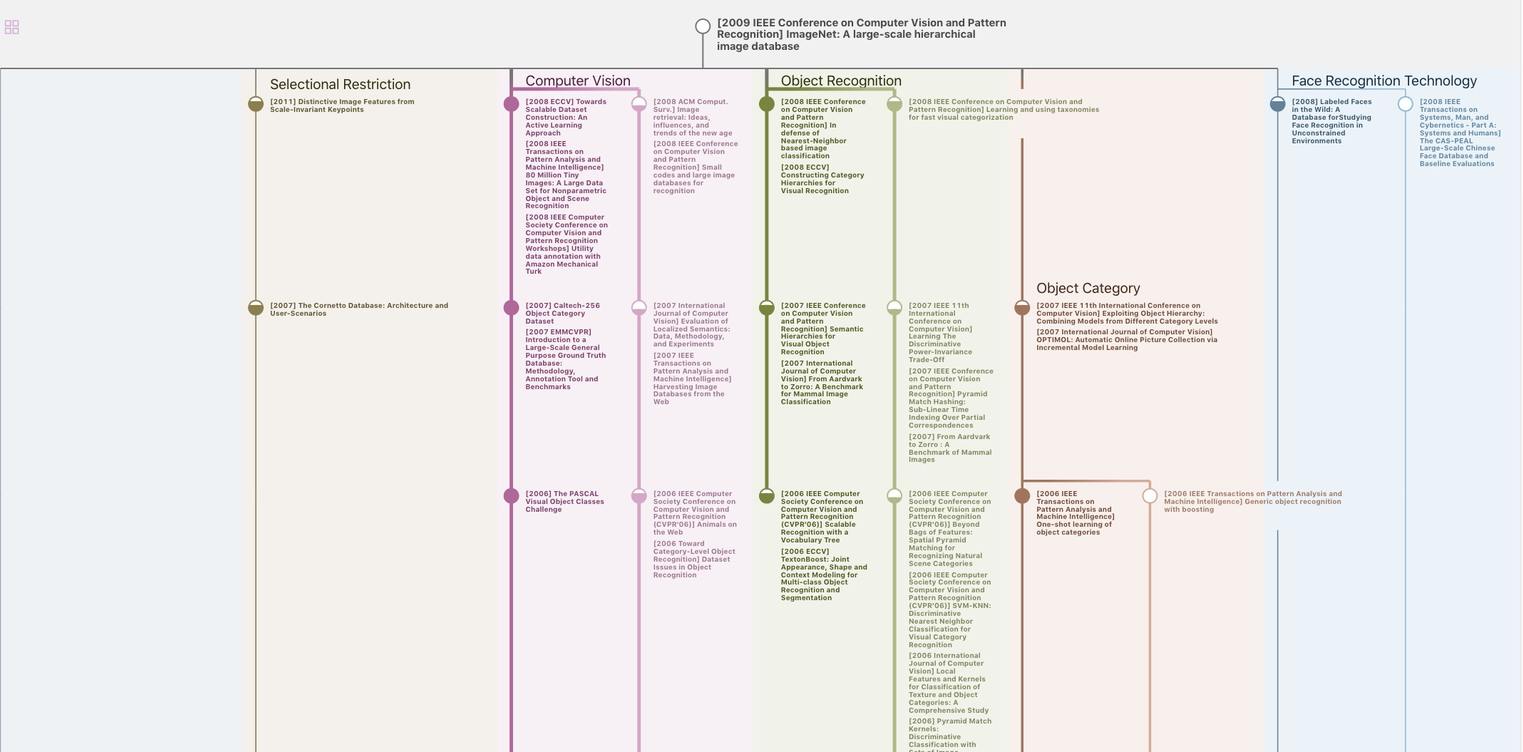
生成溯源树,研究论文发展脉络
Chat Paper
正在生成论文摘要