Finding Sparse Structures For Domain Specific Neural Machine Translation
THIRTY-FIFTH AAAI CONFERENCE ON ARTIFICIAL INTELLIGENCE, THIRTY-THIRD CONFERENCE ON INNOVATIVE APPLICATIONS OF ARTIFICIAL INTELLIGENCE AND THE ELEVENTH SYMPOSIUM ON EDUCATIONAL ADVANCES IN ARTIFICIAL INTELLIGENCE(2021)
摘要
Neural machine translation often adopts the fine-tuning approach to adapt to specific domains. However, nonrestricted fine-tuning can easily degrade on the general domain and over-fit to the target domain. To mitigate the issue, we propose PRUNE-TUNE, a novel domain adaptation method via gradual pruning. It learns tiny domain-specific sub-networks during fine-tuning on new domains. PRUNE-TUNE alleviates the over-fitting and the degradation problem without model modification. Furthermore, PRUNE-TUNE is able to sequentially learn a single network with multiple disjoint domain-specific sub-networks for multiple domains. Empirical experiment results show that PRUNE-TUNE outperforms several strong competitors in the target domain test set without sacrificing the quality on the general domain in both single and multi-domain settings. The source code and data are available at https://github.com/ohlionel/Prune-Tune.
更多查看译文
关键词
sparse structures,translation
AI 理解论文
溯源树
样例
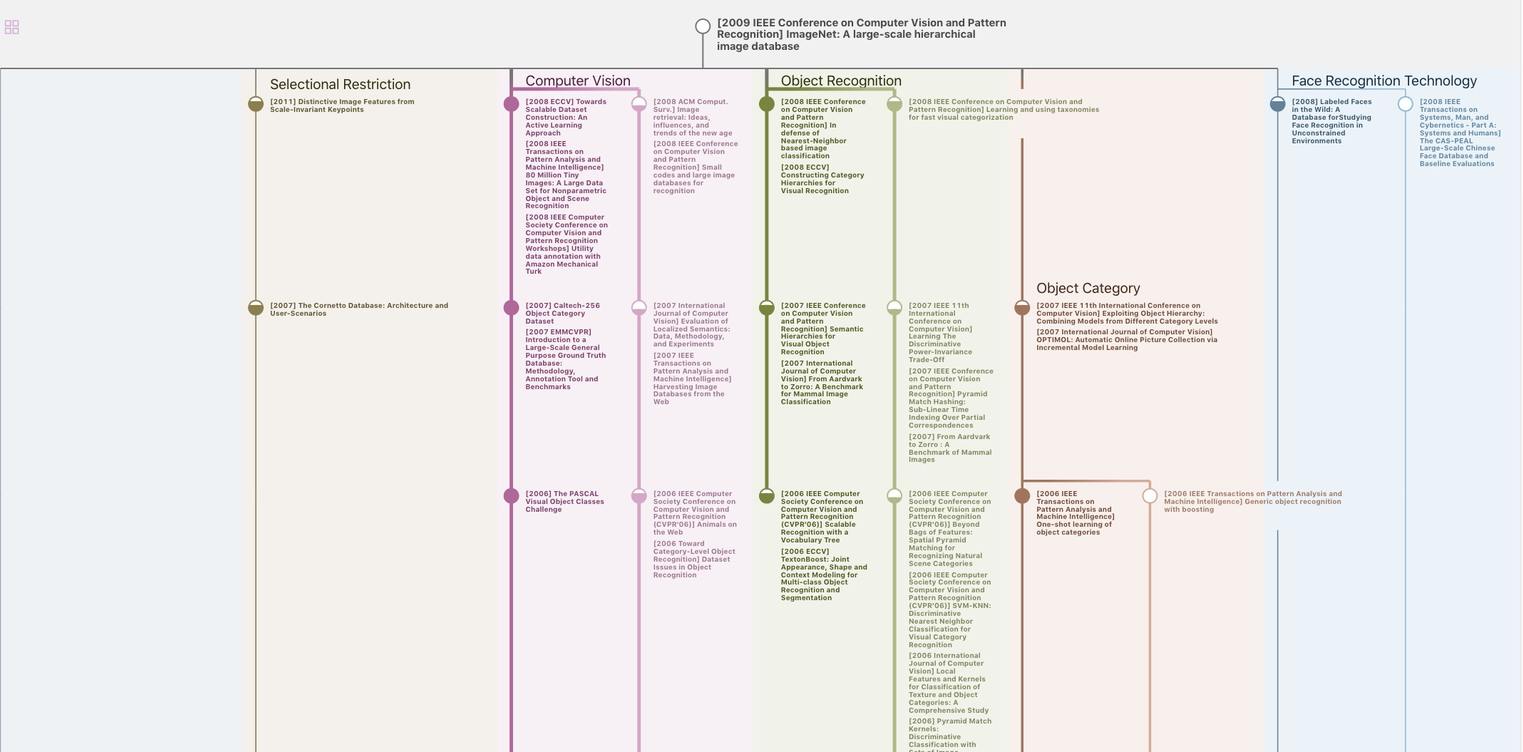
生成溯源树,研究论文发展脉络
Chat Paper
正在生成论文摘要