Covariance matrix estimation under data-based loss
Statistics & Probability Letters(2020)
摘要
In this paper, we consider the problem of estimating the $p\times p$ scale matrix $\Sigma$ of a multivariate linear regression model $Y=X\,\beta + \mathcal{E}\,$ when the distribution of the observed matrix $Y$ belongs to a large class of elliptically symmetric distributions. After deriving the canonical form $(Z^T U^T)^T$ of this model, any estimator $\hat{ \Sigma}$ of $\Sigma$ is assessed through the data-based loss tr$(S^{+}\Sigma\, (\Sigma^{-1}\hat{\Sigma} - I_p)^2 )\,$ where $S=U^T U$ is the sample covariance matrix and $S^{+}$ is its Moore-Penrose inverse. We provide alternative estimators to the usual estimators $a\,S$, where $a$ is a positive constant, which present smaller associated risk. Compared to the usual quadratic loss tr$(\Sigma^{-1}\hat{\Sigma} - I_p)^2$, we obtain a larger class of estimators and a wider class of elliptical distributions for which such an improvement occurs. A numerical study illustrates the theory.
更多查看译文
关键词
Data-based loss, Elliptically symmetric distributions, High-dimensional statistics, Orthogonally invariant estimators, Stein-Haff type identities
AI 理解论文
溯源树
样例
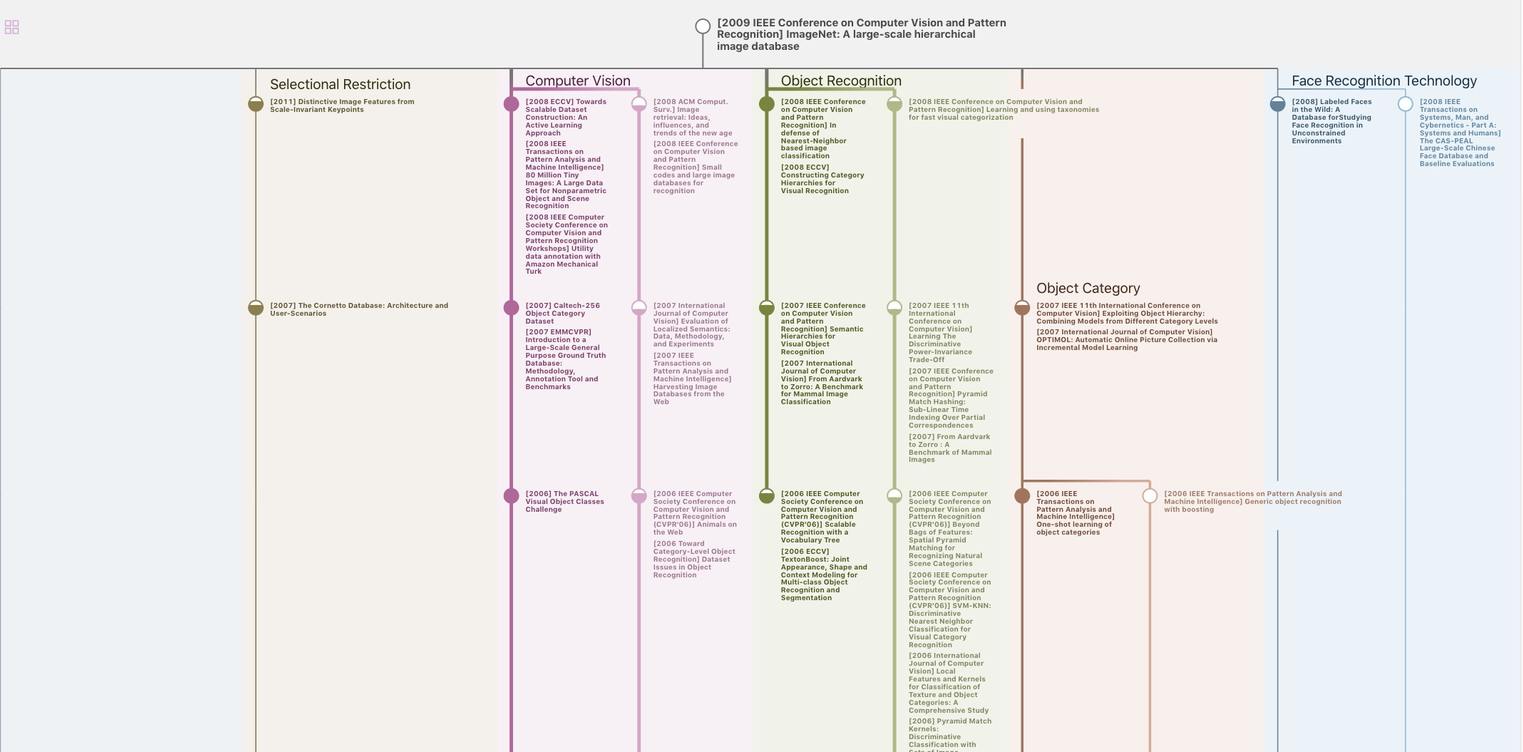
生成溯源树,研究论文发展脉络
Chat Paper
正在生成论文摘要