YolactEdge: Real-time Instance Segmentation on the Edge
2021 IEEE INTERNATIONAL CONFERENCE ON ROBOTICS AND AUTOMATION (ICRA 2021)(2021)
摘要
We propose YolactEdge, the first competitive instance segmentation approach that runs on small edge devices at real-time speeds. Specifically, YolactEdge runs at up to 30.8 FPS on a Jetson AGX Xavier (and 172.7 FPS on an RTX 2080 Ti) with a ResNet-101 backbone on 550x550 resolution images. To achieve this, we make two improvements to the state-of-the-art image-based real-time method YOLACT [1]: (1) applying TensorRT optimization while carefully trading off speed and accuracy, and (2) a novel feature warping module to exploit temporal redundancy in videos. Experiments on the YouTube VIS and MS COCO datasets demonstrate that YolactEdge produces a 3-5x speed up over existing real-time methods while producing competitive mask and box detection accuracy. We also conduct ablation studies to dissect our design choices and modules. Code and models are available at https://github.com/haotian-liu/yolact_edge.
更多查看译文
关键词
jetson agx xavier,yolactedge,rtx,yolactedge,real-time
AI 理解论文
溯源树
样例
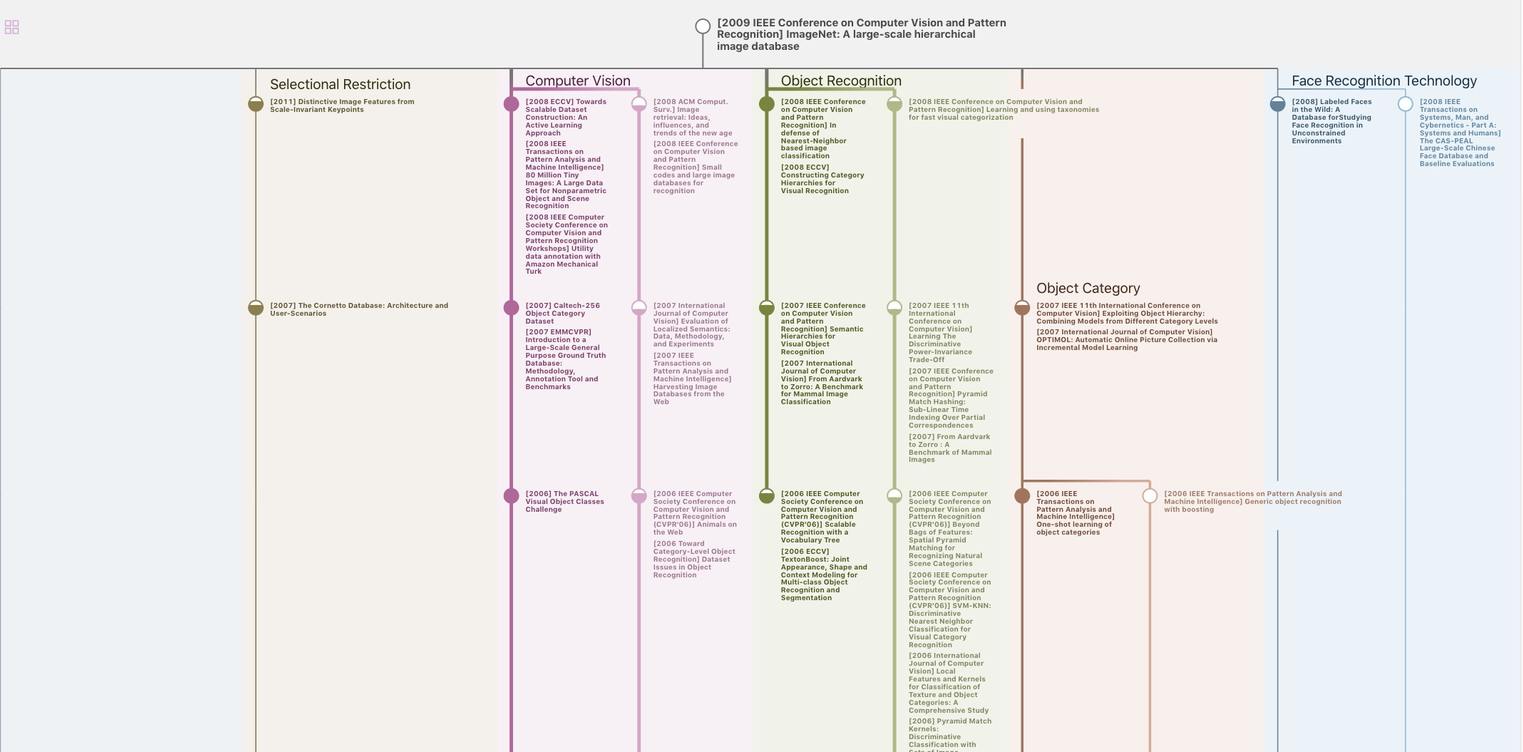
生成溯源树,研究论文发展脉络
Chat Paper
正在生成论文摘要