Effect of Shot Peening Time on Δ/γ Residual Stress Profiles of AISI 304 Weld
Journal of Materials Processing Technology(2020)
Key words
Shot peening,delta Ferrite,Residual stress,FWHM,Corrosion current intensity
AI Read Science
Must-Reading Tree
Example
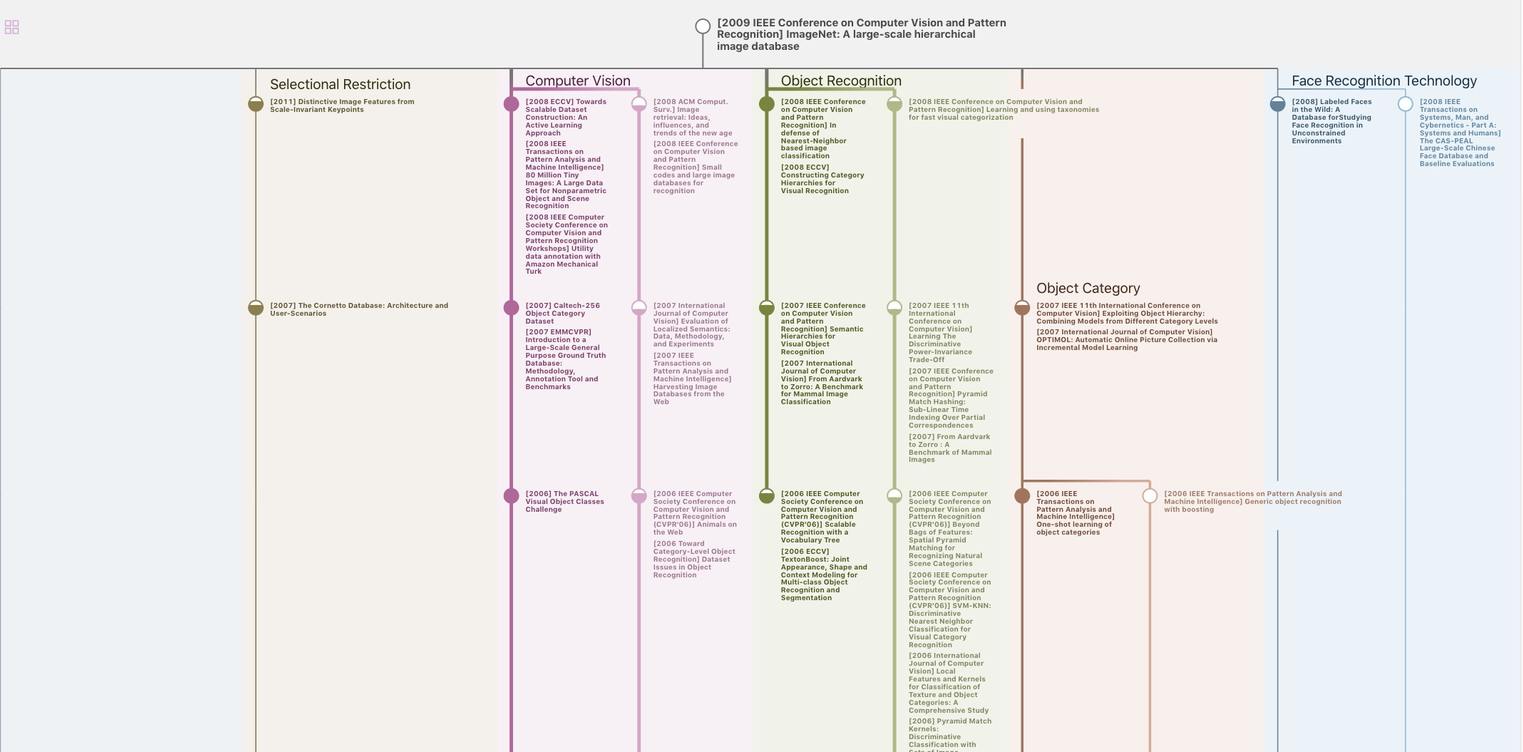
Generate MRT to find the research sequence of this paper
Chat Paper
Summary is being generated by the instructions you defined