Bayesian learning of adatom interactions from atomically-resolved imaging data
arxiv(2020)
摘要
Atomic structures and adatom geometries of surfaces encode information about the thermodynamics and kinetics of the processes that lead to their formation, and which can be captured by a generative physical model. Here we develop a workflow based on a machine learning-based analysis of scanning tunneling microscopy images to reconstruct the atomic and adatom positions, and a Bayesian optimization procedure to minimize statistical distance between the chosen physical models and experimental observations. We optimize the parameters of a 2- and 3-parameter Ising model describing surface ordering and use the derived generative model to make predictions across the parameter space. For concentration dependence, we compare the predicted morphologies at different adatom concentrations with the dissimilar regions on the sample surfaces that serendipitously had different adatom concentrations. The proposed workflow is universal and can be used to reconstruct the thermodynamic models and associated uncertainties from the experimental observations of materials microstructures. The code used in the manuscript is available at https://github.com/saimani5/Adatom_interactions.
更多查看译文
关键词
Kagome-lattice Weyl semimetal, Bayesian optimization, Ising model, Kawasaki dynamics, Monte Carlo simulations, Gaussian processes
AI 理解论文
溯源树
样例
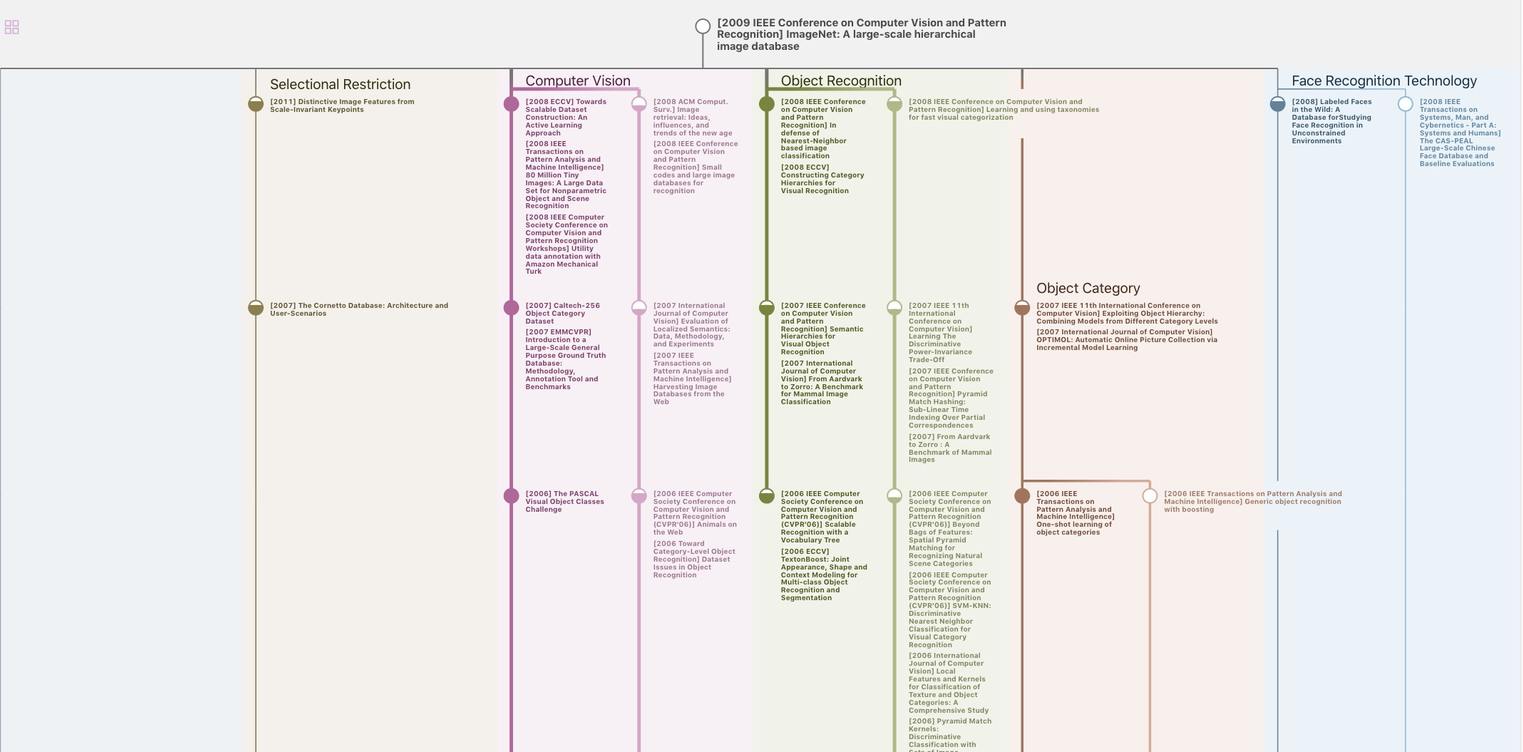
生成溯源树,研究论文发展脉络
Chat Paper
正在生成论文摘要