Warping of Radar Data Into Camera Image for Cross-Modal Supervision in Automotive Applications
IEEE Transactions on Vehicular Technology(2022)
摘要
We present an approach to automatically generate semantic labels for real recordings of automotive range-Doppler (RD) radar spectra. Such labels are required when training a neural network for object recognition from radar data. The automatic labeling approach rests on the simultaneous recording of camera and lidar data in addition to the radar spectrum. By warping radar spectra into the camera image, state-of-the-art object recognition algorithms can be applied to label relevant objects, such as cars, in the camera image. The warping operation is designed to be fully differentiable, which allows backpropagating the gradient computed on the camera image through the warping operation to the neural network operating on the radar data. As the warping operation relies on accurate scene flow estimation, we further propose a novel scene flow estimation algorithm which exploits information from camera, lidar and radar sensors. The proposed scene flow estimation approach is compared against a state-of-the-art scene flow algorithm, and it outperforms it by approximately 30% w.r.t. mean average error. The feasibility of the overall framework for automatic label generation for RD spectra is verified by evaluating the performance of neural networks trained with the proposed framework for Direction-of-Arrival estimation.
更多查看译文
关键词
Automotive radar,neural network,lidar,virtual testing,direction-of-arrival,cross-modal supervision
AI 理解论文
溯源树
样例
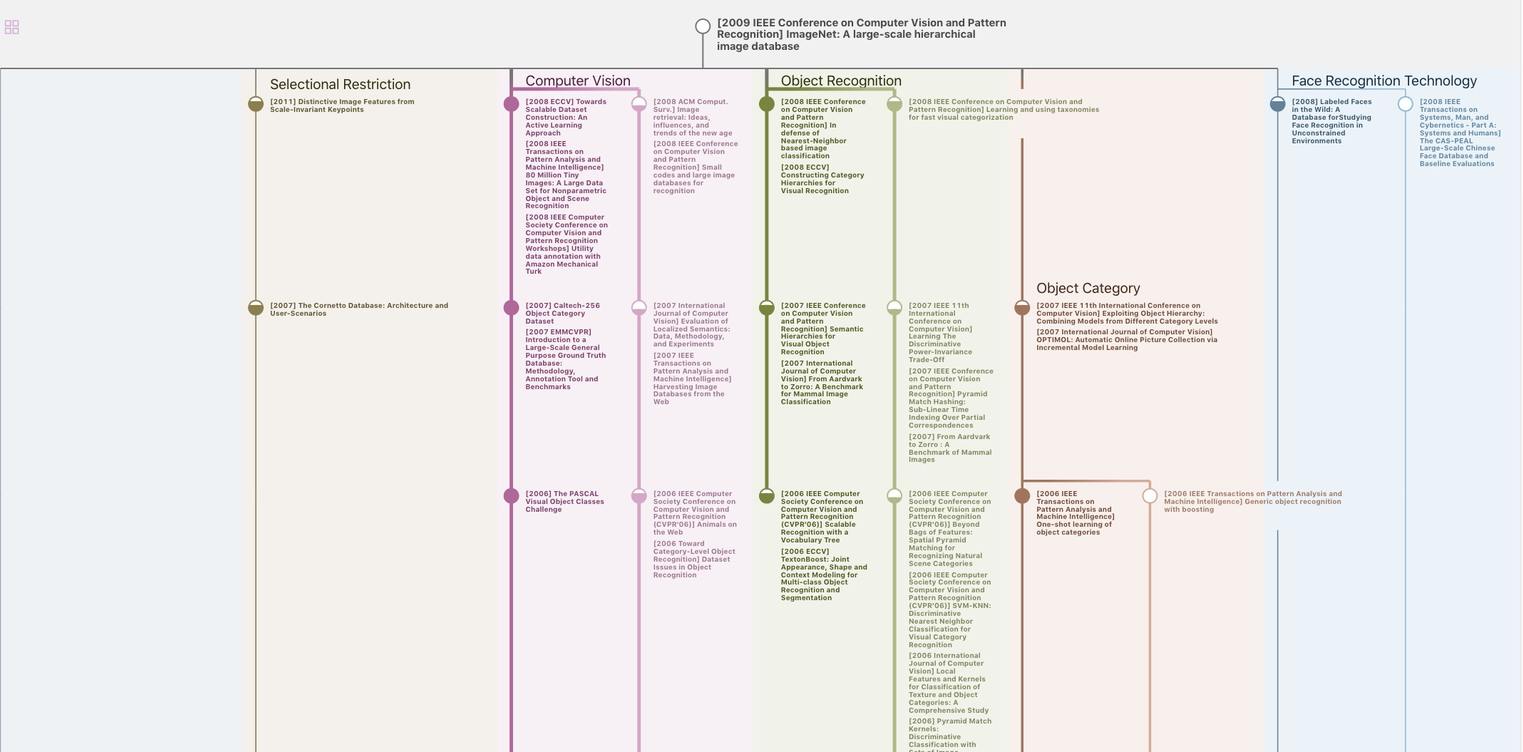
生成溯源树,研究论文发展脉络
Chat Paper
正在生成论文摘要