AsymptoticNG: A regularized natural gradient optimization algorithm with look-ahead strategy
arxiv(2020)
摘要
Optimizers that further adjust the scale of gradient, such as Adam, Natural Gradient (NG), etc., despite widely concerned and used by the community, are often found poor generalization performance, compared with Stochastic Gradient Descent (SGD). They tend to converge excellently at the beginning of training but are weak at the end. An immediate idea is to complement the strengths of these algorithms with SGD. However, a truncated replacement of optimizer often leads to a crash of the update pattern, and new algorithms often require many iterations to stabilize their search direction. Driven by this idea and to address this problem, we design and present a regularized natural gradient optimization algorithm with look-ahead strategy, named asymptotic natural gradient (ANG). According to the total iteration step, ANG dynamic assembles NG and Euclidean gradient, and updates parameters along the new direction using the intensity of NG. Validation experiments on CIFAR10 and CIFAR100 data sets show that ANG can update smoothly and stably at the second-order speed, and achieve better generalization performance.
更多查看译文
关键词
natural gradient optimization algorithm,look-ahead
AI 理解论文
溯源树
样例
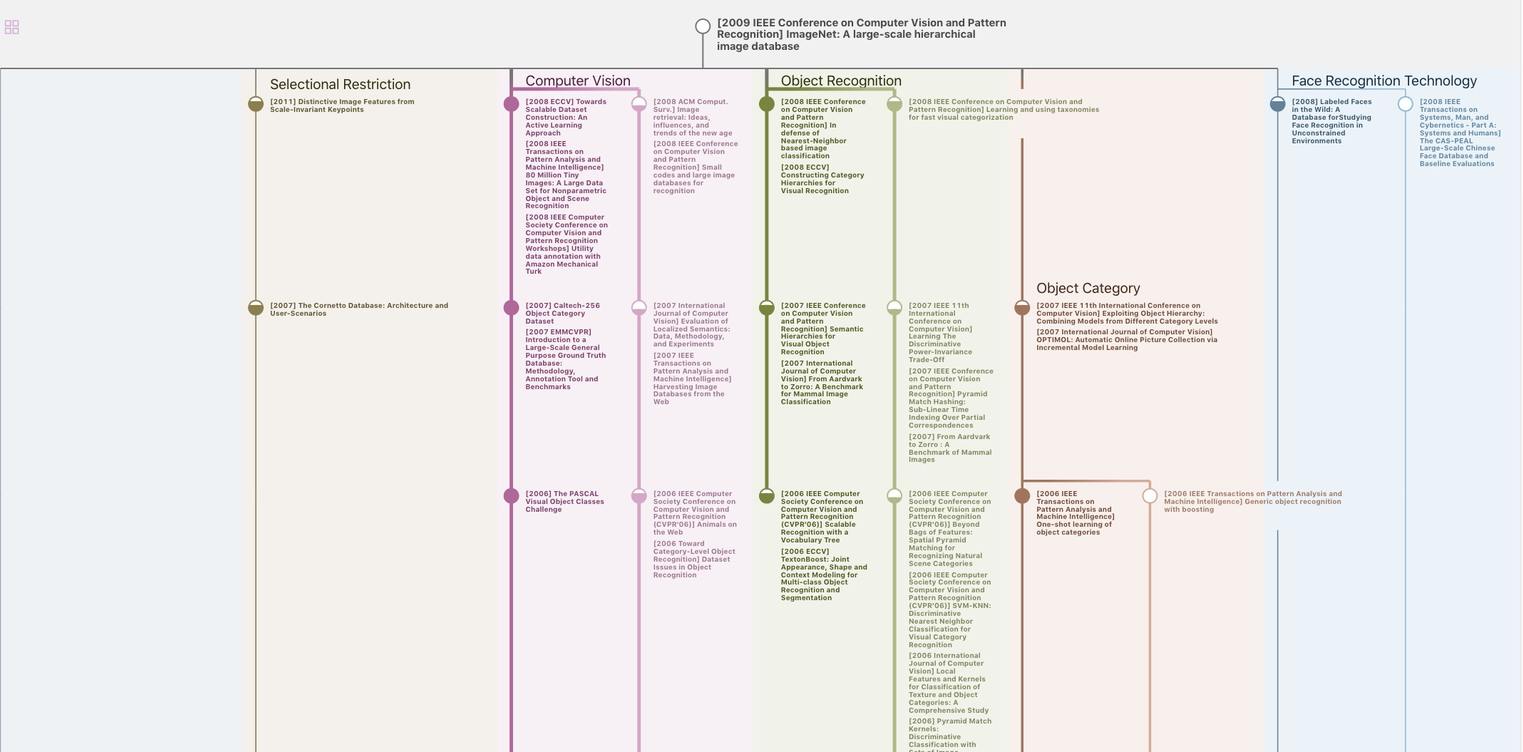
生成溯源树,研究论文发展脉络
Chat Paper
正在生成论文摘要