Towards Coordinated Robot Motions: End-to-End Learning of Motion Policies on Transform Trees
2021 IEEE/RSJ INTERNATIONAL CONFERENCE ON INTELLIGENT ROBOTS AND SYSTEMS (IROS)(2021)
摘要
Generating robot motion that fulfills multiple tasks simultaneously is challenging due to the geometric constraints imposed on the robot. In this paper, we propose to solve multi-task problems through learning structured policies from human demonstrations. Our structured policy is inspired by RMPflow, a framework for combining subtask policies on different spaces. The policy structure provides the user an interface to 1) specifying the spaces that are directly relevant to the completion of the tasks, and 2) designing policies for certain tasks that do not need to be learned. We derive an end-to-end learning objective that is suitable for the multi-task problem, emphasizing the distance between generated motions and demonstrations measured on task spaces. Furthermore, the motion generated from the learned policy class is guaranteed to be stable. We validate the effectiveness of our proposed learning framework through qualitative and quantitative evaluations on three robotic tasks on a 7-DOF Rethink Sawyer robot.
更多查看译文
关键词
coordinated robot motions,transform trees,end-to-end
AI 理解论文
溯源树
样例
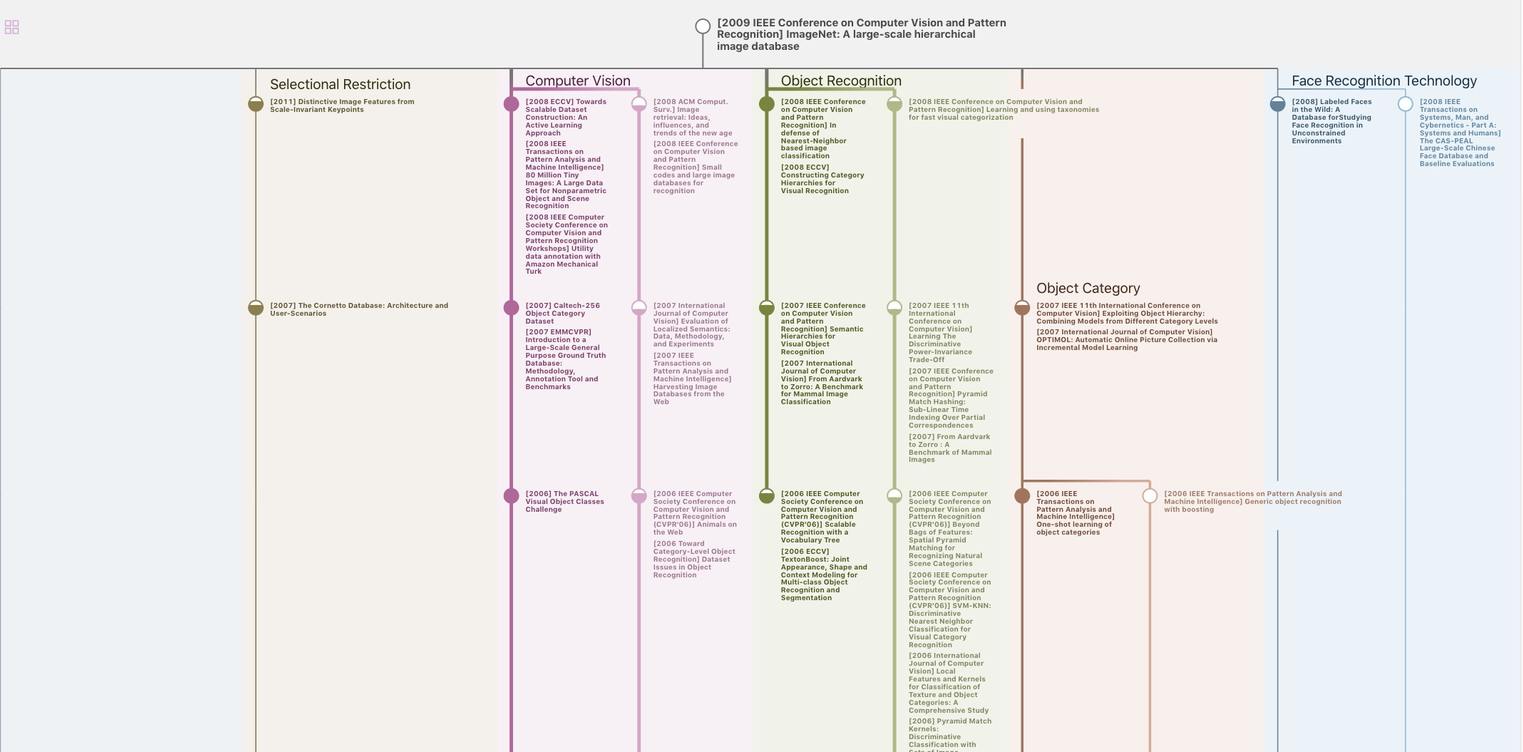
生成溯源树,研究论文发展脉络
Chat Paper
正在生成论文摘要