ON SELF-SUPERVISED MULTIMODAL REPRESENTATION LEARNING: AN APPLICATION TO ALZHEIMER'S DISEASE
2021 IEEE 18TH INTERNATIONAL SYMPOSIUM ON BIOMEDICAL IMAGING (ISBI)(2021)
摘要
Introspection of deep supervised predictive models trained on functional and structural brain imaging may uncover novel markers of Alzheimer's disease (AD). However, supervised training is prone to learning from spurious features (shortcut learning), impairing its value in the discovery process. Deep unsupervised and, recently, contrastive self-supervised approaches, not biased to classification, arc better candidates for the task. Their multimodal options specifically offer additional regularization via modality interactions. This paper introduces a way to exhaustively consider multimodal architectures for a contrastive self-supervised fusion of fMRI and MRI of AD patients and controls. We show that this multimodal fusion results in representations that improve the downstream classification results for both modalities. We investigate the fused self-supervised features projected into the brain space and introduce a numerically stable way to do so.
更多查看译文
关键词
Multimodal data fusion, Neuroimaging, Mutual Information, Deep Learning
AI 理解论文
溯源树
样例
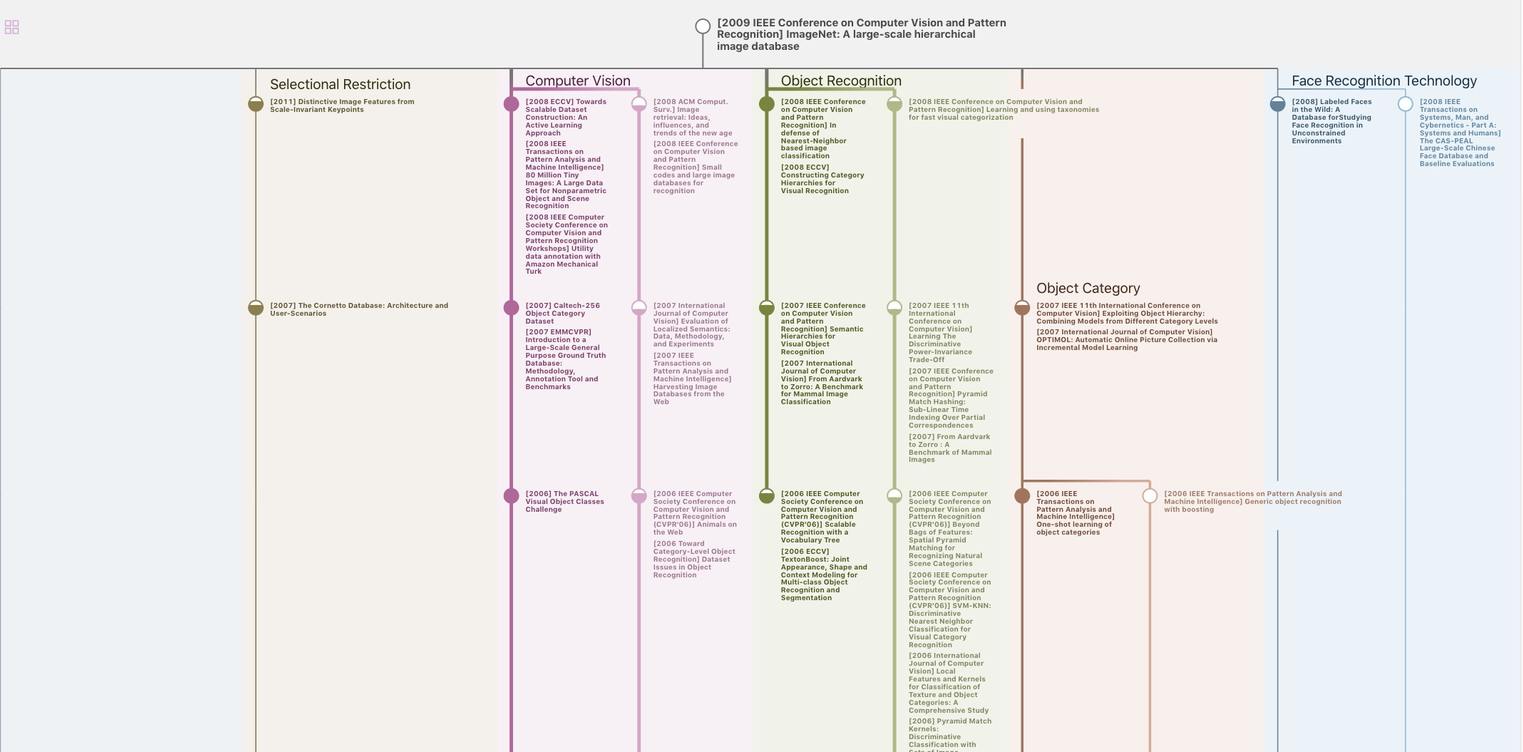
生成溯源树,研究论文发展脉络
Chat Paper
正在生成论文摘要