Dual-Refinement: Joint Label And Feature Refinement For Unsupervised Domain Adaptive Person Re-Identification
IEEE TRANSACTIONS ON IMAGE PROCESSING(2021)
摘要
Unsupervised domain adaptive (UDA) person re-identification (re-ID) is a challenging task due to the missing of labels for the target domain data. To handle this problem, some recent works adopt clustering algorithms to off-line generate pseudo labels, which can then be used as the supervision signal for on-line feature learning in the target domain. However, the off-line generated labels often contain lots of noise that significantly hinders the discriminability of the on-line learned features, and thus limits the final UDA re-ID performance. To this end, we propose a novel approach, called Dual-Refinement, that jointly refines pseudo labels at the off-line clustering phase and features at the on-line training phase, to alternatively boost the label purity and feature discriminability in the target domain for more reliable re-ID. Specifically, at the off-line phase, a new hierarchical clustering scheme is proposed, which selects representative prototypes for every coarse cluster. Thus, labels can be effectively refined by using the inherent hierarchical information of person images. Besides, at the on-line phase, we propose an instant memory spread-out (IM-spread-out) regularization, that takes advantage of the proposed instant memory bank to store sample features of the entire dataset and enable spread-out feature learning over the entire training data instantly. Our Dual-Refinement method reduces the influence of noisy labels and refines the learned features within the alternative training process. Experiments demonstrate that our method outperforms the state-of-the-art methods by a large margin.
更多查看译文
关键词
Noise measurement, Training, Prototypes, Reliability, Training data, Adaptation models, Refining, Person re-ID, unsupervised domain adaption, pseudo label noise
AI 理解论文
溯源树
样例
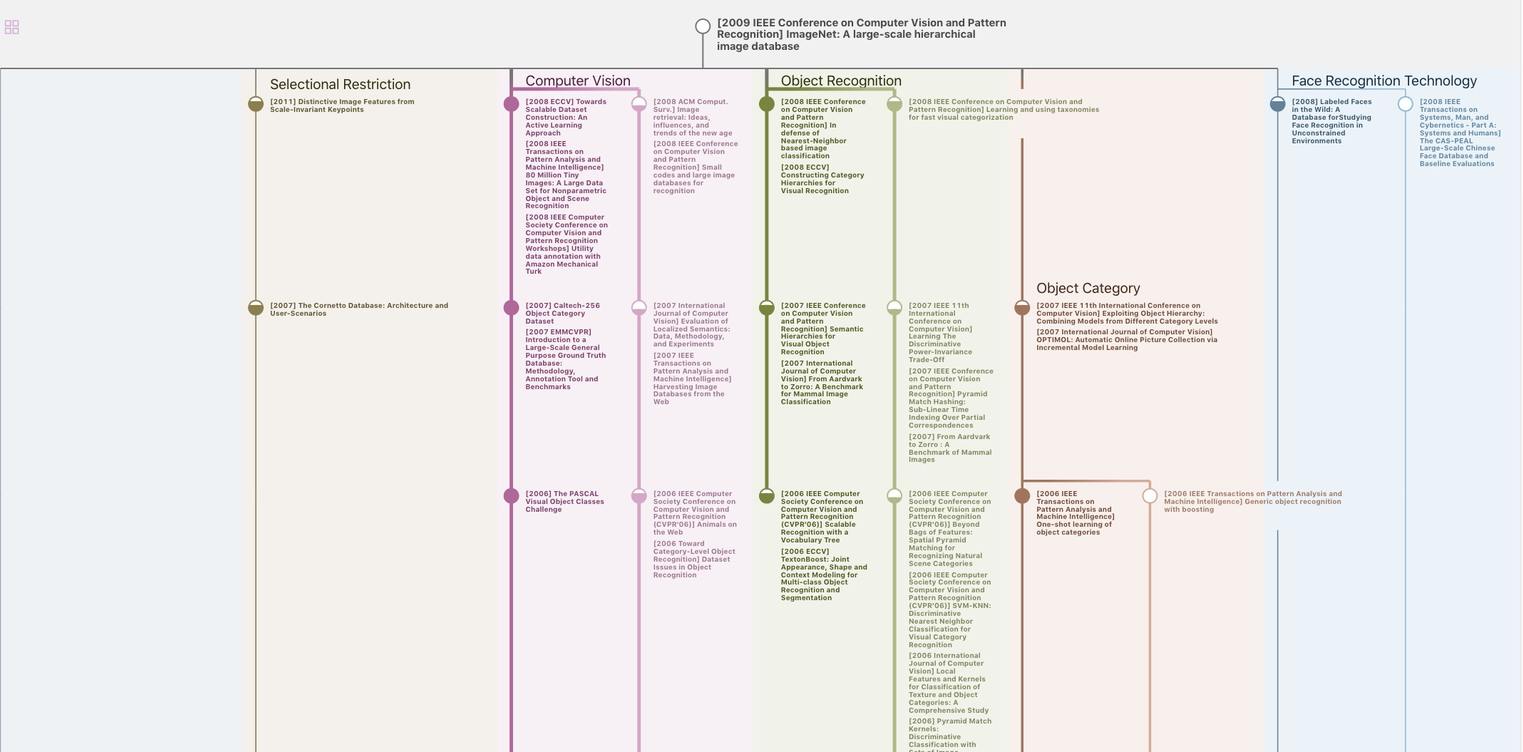
生成溯源树,研究论文发展脉络
Chat Paper
正在生成论文摘要