Jacobian-based learning for inverse kinematics of soft robots
arXiv (Cornell University)(2020)
Abstract
This paper presents a new method to solve the inverse kinematic (IK) problem in real-time on soft robots with highly non-linear deformation. The major challenge of efficiently computing IK for such robots is caused by the lack of analytical formulation for either forward or inverse kinematics. To tackle this challenge, we employ neural-networks to learn both the mapping function of forward kinematics and also the Jacobian of this function. As a result, Jacobian-based iteration can be applied to solve the IK problem. A sim-to-real training transfer strategy is conducted to make this approach more practical. We first generate large amount of samples in a simulation environment for learning both the kinematic and the Jacobian networks of a soft robot design. After that, a sim-to-real layer of differentiable neurons is employed to map the results of simulation to the physical hardware, where this sim-to-real layer can be learned from very limited number of training samples generated on the hardware. The effectiveness of our approach has been verified on several pneumatic-driven soft robots in the tasks of trajectory following and interactive positioning.
MoreTranslated text
Key words
Inverse kinematics (IKs),Jacobian,learning,sim-to-real,soft robots
AI Read Science
Must-Reading Tree
Example
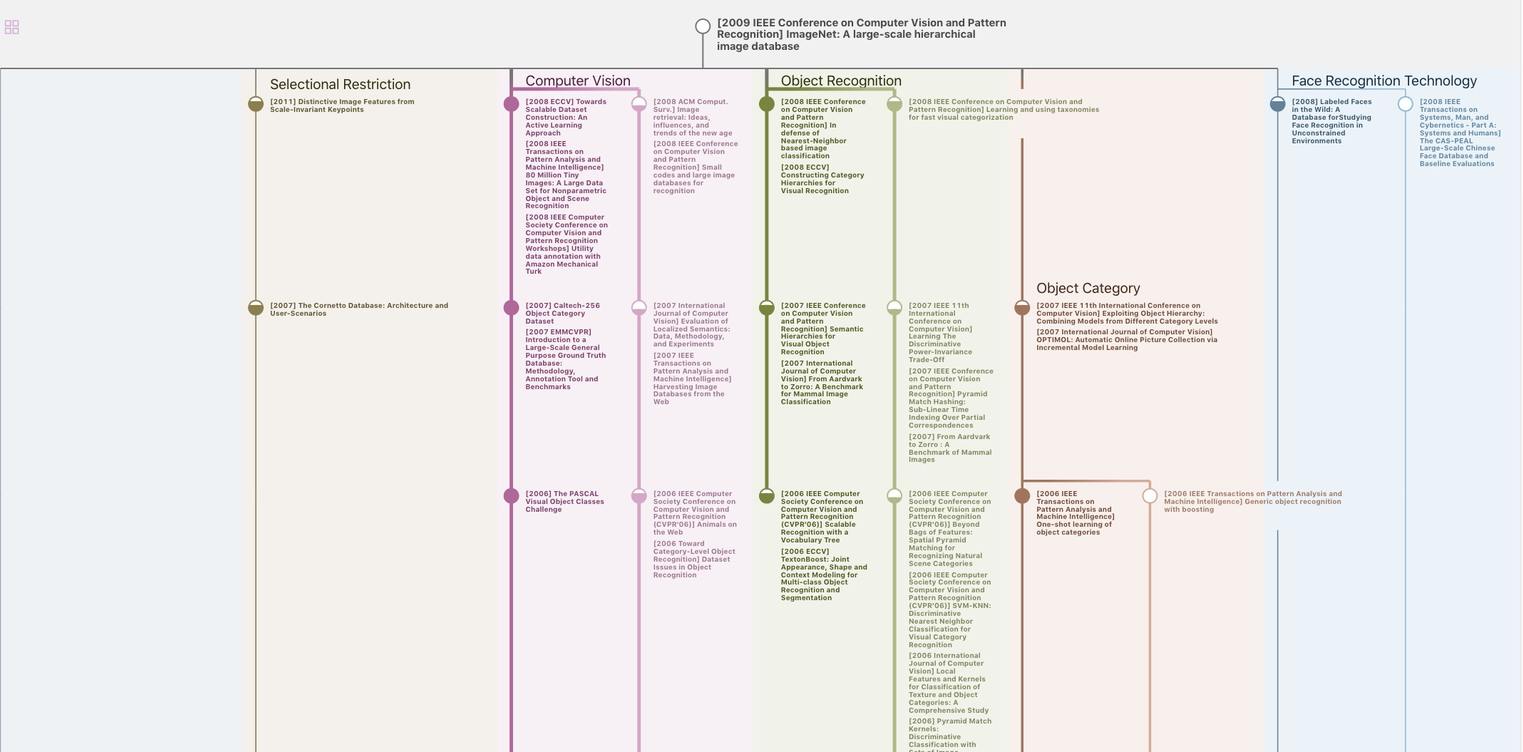
Generate MRT to find the research sequence of this paper
Chat Paper
Summary is being generated by the instructions you defined