Latent space models for multiplex networks with shared structure
BIOMETRIKA(2022)
摘要
Latent space models are frequently used for modelling single-layer networks and include many popular special cases, such as the stochastic block model and the random dot product graph. However, they are not well developed for more complex network structures, which are becoming increasingly common in practice. In this article we propose a new latent space model for multiplex networks, i.e., multiple heterogeneous networks observed on a shared node set. Multiplex networks can represent a network sample with shared node labels, a network evolving over time, or a network with multiple types of edges. The key feature of the proposed model is that it learns from data how much of the network structure is shared between layers and pools information across layers as appropriate. We establish identifiability, develop a fitting procedure using convex optimization in combination with a nuclear-norm penalty, and prove a guarantee of recovery for the latent positions provided there is sufficient separation between the shared and the individual latent subspaces. We compare the model with competing methods in the literature on simulated networks and on a multiplex network describing the worldwide trade of agricultural products.
更多查看译文
关键词
Latent space model, Multilayer nework, Multiplex network
AI 理解论文
溯源树
样例
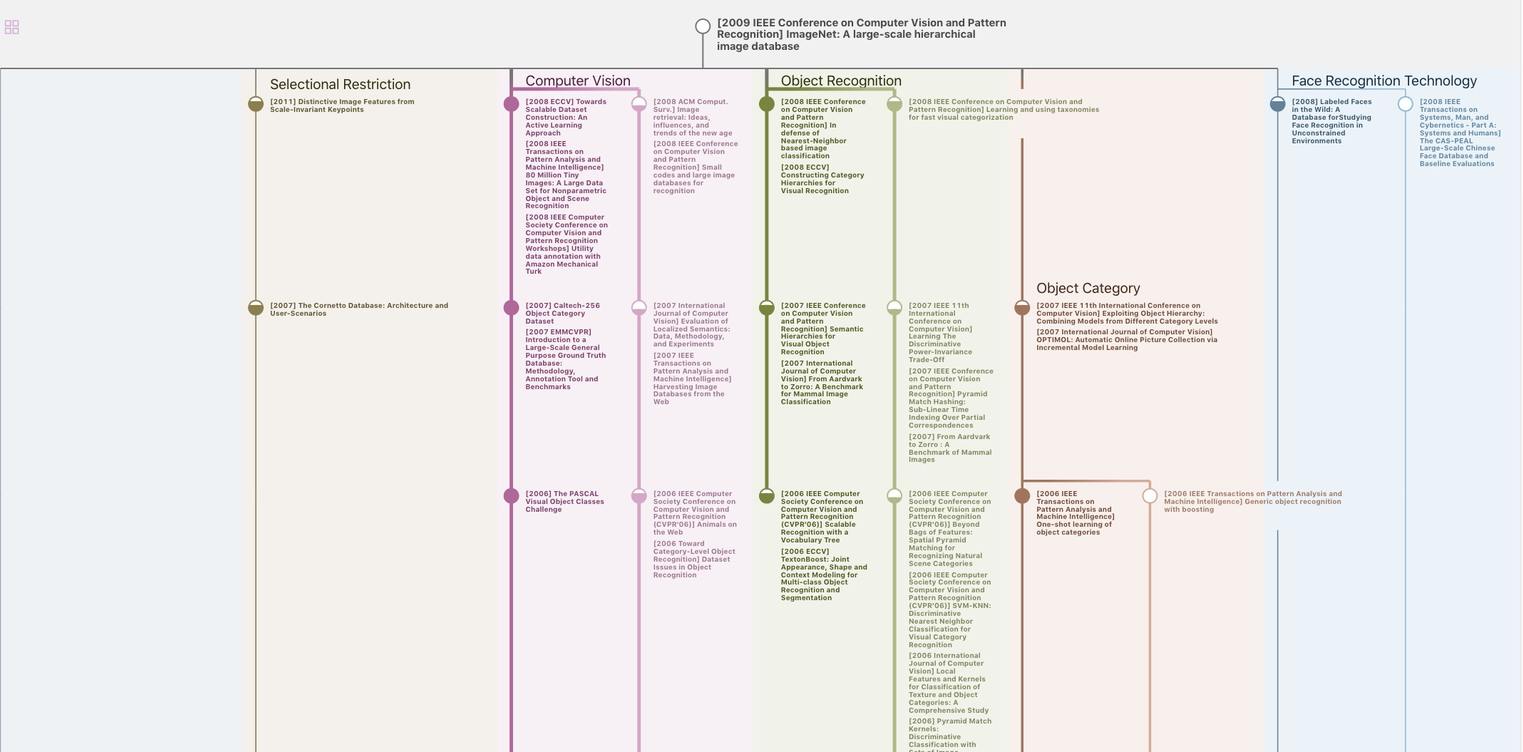
生成溯源树,研究论文发展脉络
Chat Paper
正在生成论文摘要