Sparse deep dictionary learning identifies differences of time-varying functional connectivity in brain neuro-developmental study
Neural Networks(2021)
摘要
Recently, the focus of functional connectivity analysis of human brain has shifted from merely revealing the inter-regional functional correlation over the entire scan duration to capturing the time-varying information of brain networks and characterizing time-resolved reoccurring patterns of connectivity. Much effort has been invested into developing approaches that can track changes in re-occurring patterns of functional connectivity over time. In this paper, we propose a sparse deep dictionary learning method to characterize the essential differences of reoccurring patterns of time-varying functional connectivity between different age groups. The proposed method combines both the interpretability of sparse dictionary learning and the capability of extracting sparse nonlinear higher-level features in the latent space of sparse deep autoencoder. In other words, it learns a sparse dictionary of the original data by considering the nonlinear representation of the data in the encoder layer based on a sparse deep autoencoder. In this way, the nonlinear structure and higher-level features of the data can be captured by deep dictionary learning. The proposed method is applied to the analysis of the Philadelphia Neurodevelopmental Cohort. It shows that there exist essential differences in the reoccurrence patterns of function connectivity between child and young adult groups. Specially, children have more diffusive functional connectivity patterns while young adults possess more focused functional connectivity patterns, and the brain function transits from undifferentiated systems to specialized neural networks with the growth.
更多查看译文
关键词
Deep dictionary learning,Deep autoencoder,Sparsity,Dynamic functional connectivity,Reoccurring pattern,Brain development
AI 理解论文
溯源树
样例
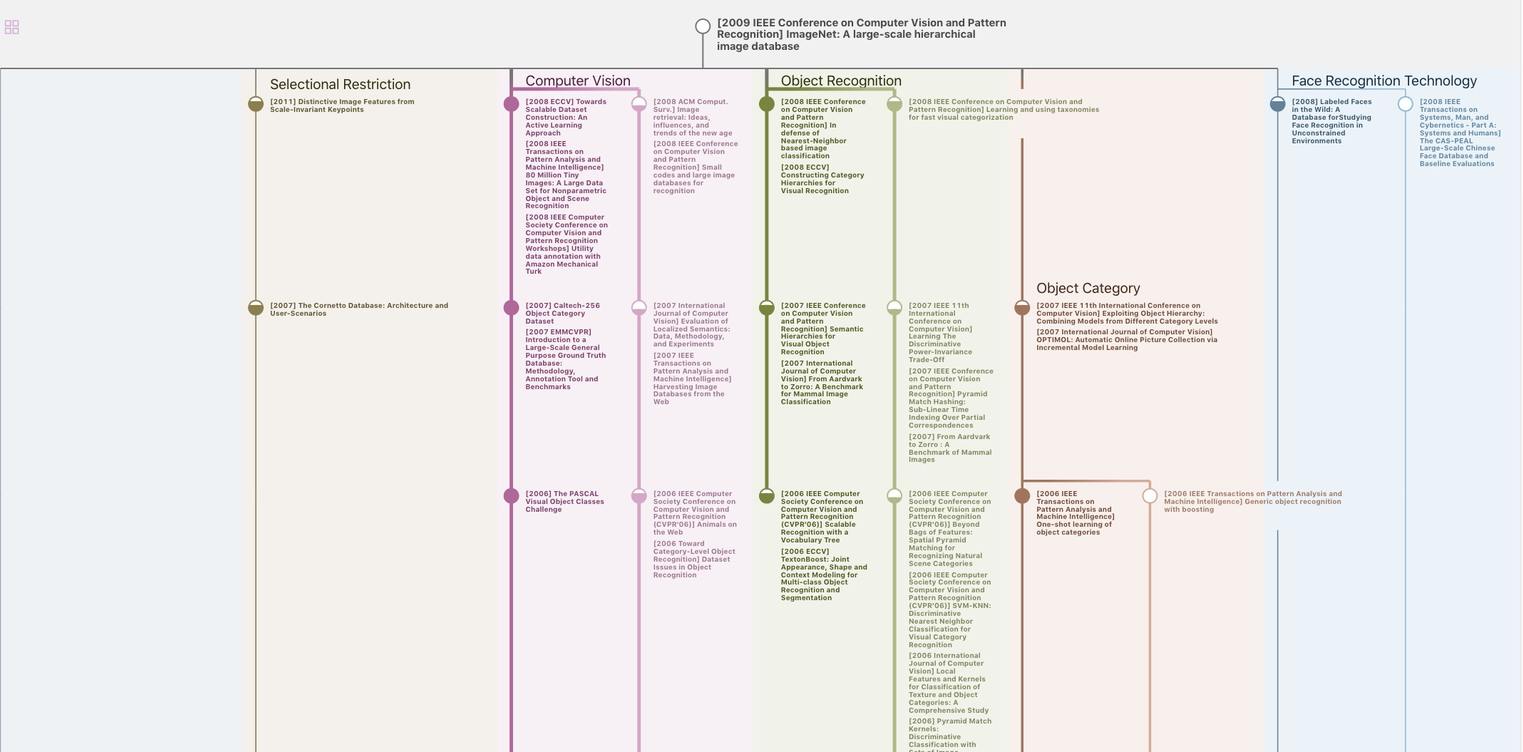
生成溯源树,研究论文发展脉络
Chat Paper
正在生成论文摘要