Deep Learning Detection Of Sea Fan Neovascularization From Ultra-Widefield Color Fundus Photographs Of Patients With Sickle Cell Hemoglobinopathy
JAMA OPHTHALMOLOGY(2021)
摘要
Importance Adherence to screening for vision-threatening proliferative sickle cell retinopathy is limited among patients with sickle cell hemoglobinopathy despite guidelines recommending dilated fundus examinations beginning in childhood. An automated algorithm for detecting sea fan neovascularization from ultra-widefield color fundus photographs could expand access to rapid retinal evaluations to identify patients at risk of vision loss from proliferative sickle cell retinopathy. Objective To develop a deep learning system for detecting sea fan neovascularization from ultra-widefield color fundus photographs from patients with sickle cell hemoglobinopathy. Design, Setting, and Participants In a cross-sectional study conducted at a single-institution, tertiary academic referral center, deidentified, retrospectively collected, ultra-widefield color fundus photographs from 190 adults with sickle cell hemoglobinopathy were independently graded by 2 masked retinal specialists for presence or absence of sea fan neovascularization. A third masked retinal specialist regraded images with discordant or indeterminate grades. Consensus retinal specialist reference standard grades were used to train a convolutional neural network to classify images for presence or absence of sea fan neovascularization. Participants included nondiabetic adults with sickle cell hemoglobinopathy receiving care from a Wilmer Eye Institute retinal specialist; the patients had received no previous laser or surgical treatment for sickle cell retinopathy and underwent imaging with ultra-widefield color fundus photographs between January 1, 2012, and January 30, 2019. Interventions Deidentified ultra-widefield color fundus photographs were retrospectively collected. Main Outcomes and Measures Sensitivity, specificity, and area under the receiver operating characteristic curve of the convolutional neural network for sea fan detection. Results A total of 1182 images from 190 patients were included. Of the 190 patients, 101 were women (53.2%), and the mean (SD) age at baseline was 36.2 (12.3) years; 119 patients (62.6%) had hemoglobin SS disease and 46 (24.2%) had hemoglobin SC disease. One hundred seventy-nine patients (94.2%) were of Black or African descent. Images with sea fan neovascularization were obtained in 57 patients (30.0%). The convolutional neural network had an area under the curve of 0.988 (95% CI, 0.969-0.999), with sensitivity of 97.4% (95% CI, 86.5%-99.9%) and specificity of 97.0% (95% CI, 93.5%-98.9%) for detecting sea fan neovascularization from ultra-widefield color fundus photographs. Conclusions and Relevance This study reports an automated system with high sensitivity and specificity for detecting sea fan neovascularization from ultra-widefield color fundus photographs from patients with sickle cell hemoglobinopathy, with potential applications for improving screening for vision-threatening proliferative sickle cell retinopathy.This cross-sectional study evaluates the development of a deep learning method to detect sea fan neovascularization in patients with sickle cell hemoglobinopathy.Question Can deep learning automate detection of sea fan neovascularization from ultra-widefield color fundus photographs? Findings In a cross-sectional study using 1182 ultra-widefield color fundus photographs from 190 patients with sickle cell hemoglobinopathy, a convolutional neural network was trained to classify images for presence or absence of sea fan neovascularization. The sensitivity was 97.4% and specificity was 97.0% for detecting sea fans. Meaning Automated detection of sea fan neovascularization from ultra-widefield color fundus photographs may facilitate screening for vision-threatening proliferative sickle cell retinopathy.
更多查看译文
AI 理解论文
溯源树
样例
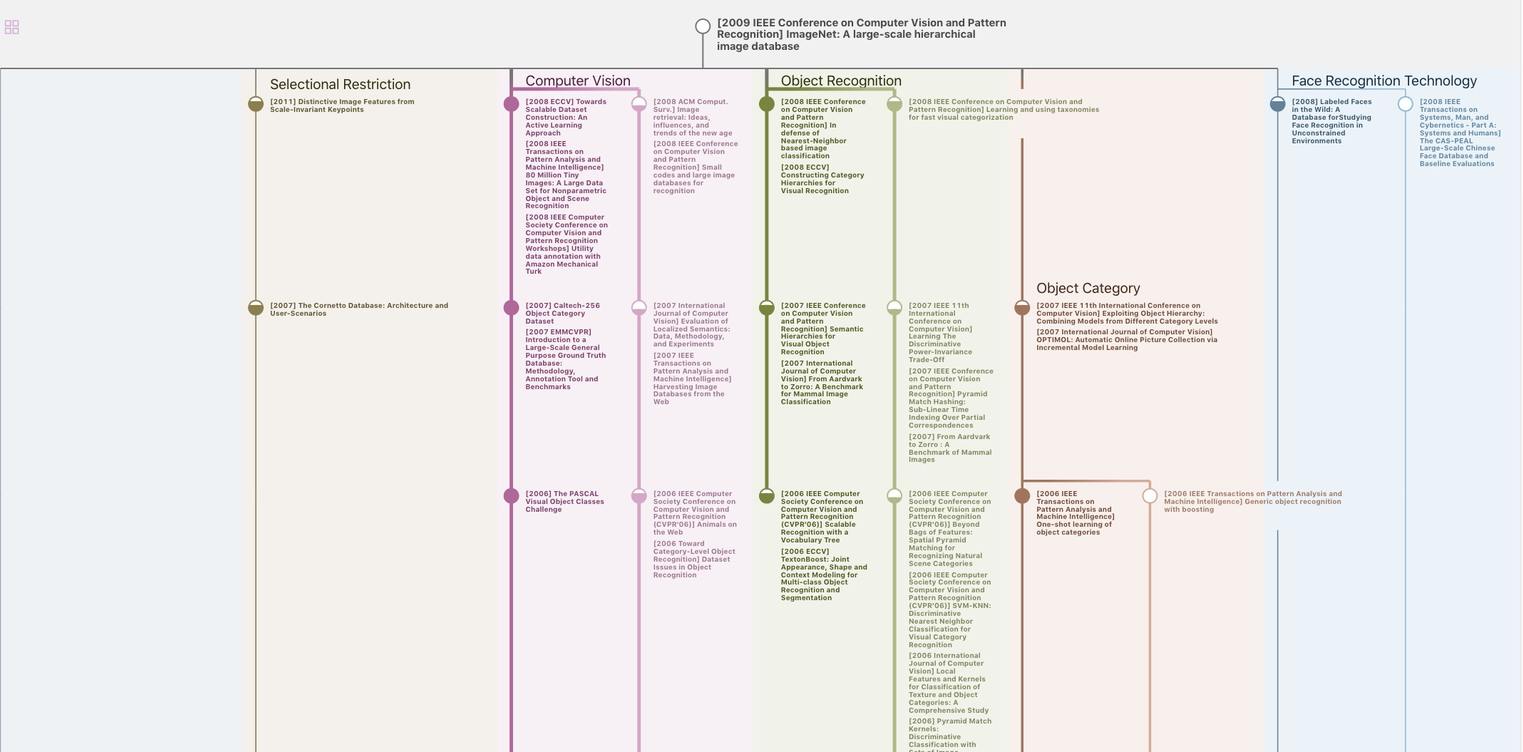
生成溯源树,研究论文发展脉络
Chat Paper
正在生成论文摘要