How Much Deep Learning Is Enough For Automatic Identification To Be Reliable? A Cephalometric Example
ANGLE ORTHODONTIST(2020)
摘要
Objectives: To determine the optimal quantity of learning data needed to develop artificial intelligence (AI) that can automatically identify cephalometric landmarks.Materials and Methods: A total of 2400 cephalograms were collected, and 80 landmarks were manually identified by a human examiner. Of these, 2200 images were chosen as the learning data to train AI. The remaining 200 images were used as the test data. A total of 24 combinations of the quantity of learning data (50, 100, 200, 400, 800, 1600, and 2000) were selected by the random sampling method without replacement, and the number of detecting targets per image (19, 40, and 80) were used in the AI training procedures. The training procedures were repeated four times. A total of 96 different AIs were produced. The accuracy of each AI was evaluated in terms of radial error.Results: The accuracy of AI increased linearly with the increasing number of learning data sets on a logarithmic scale. It decreased with increasing numbers of detection targets. To estimate the optimal quantity of learning data, a prediction model was built. At least 2300 sets of learning data appeared to be necessary to develop AI as accurate as human examiners.Conclusions: A considerably large quantity of learning data was necessary to develop accurate AI. The present study might provide a basis to determine how much learning data would be necessary in developing AI.
更多查看译文
关键词
Artificial intelligence, Deep learning, Data quantity, Logarithmic transformation
AI 理解论文
溯源树
样例
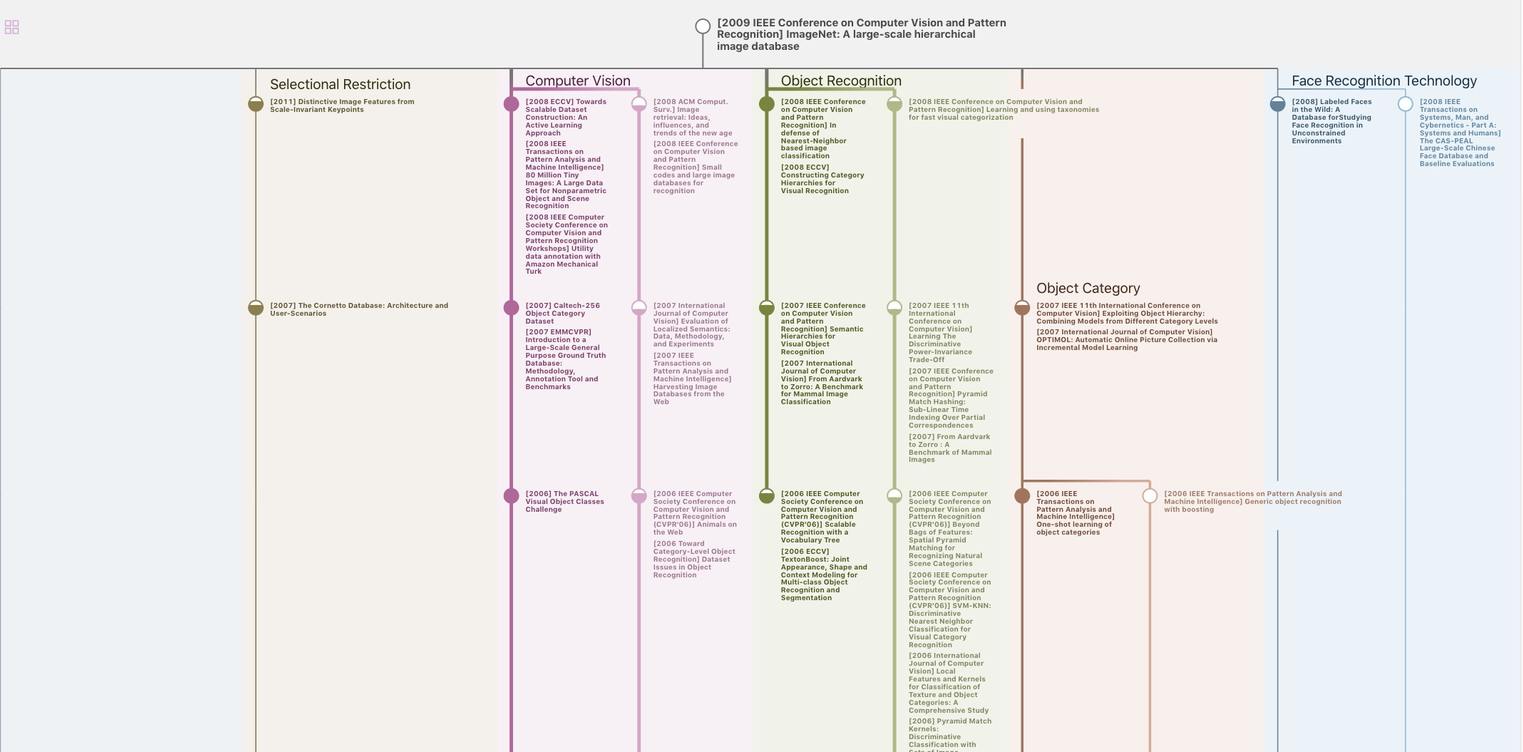
生成溯源树,研究论文发展脉络
Chat Paper
正在生成论文摘要