Control Barriers in Bayesian Learning of System Dynamics
IEEE Transactions on Automatic Control(2023)
摘要
This article focuses on learning a model of system dynamics online, while satisfying safety constraints. Our objective is to avoid offline system identification or hand-specified models and allow a system to safely and autonomously estimate and adapt its own model during operation. Given streaming observations of the system state, we use Bayesian learning to obtain a distribution over the system dynamics. Specifically, we propose a new matrix variate Gaussian process (MVGP) regression approach with an efficient covariance factorization to learn the drift and input gain terms of a nonlinear control-affine system. The MVGP distribution is then used to optimize the system behavior and ensure safety with high probability, by specifying control Lyapunov function (CLF) and control barrier function (CBF) chance constraints. We show that a safe control policy can be synthesized for systems with arbitrary relative degree and probabilistic CLF-CBF constraints by solving a second-order cone program. Finally, we extend our design to a self-triggering formulation, adaptively determining the time at which a new control input needs to be applied in order to guarantee safety.
更多查看译文
关键词
Control barrier function (CBF),Gaussian process,high relative-degree system safety,learning for dynamics and control,self-triggered safe control
AI 理解论文
溯源树
样例
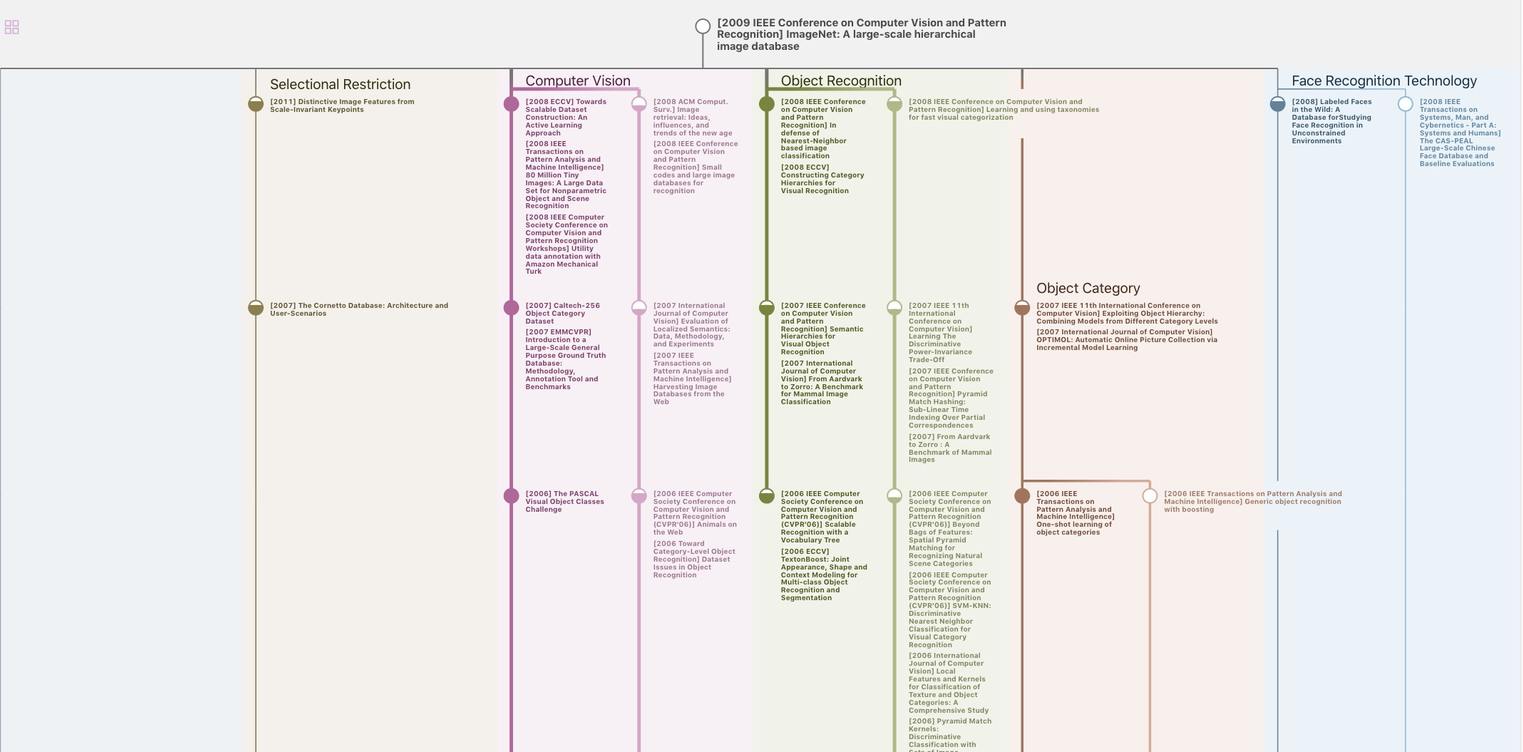
生成溯源树,研究论文发展脉络
Chat Paper
正在生成论文摘要