Fully Non-autoregressive Neural Machine Translation - Tricks of the Trade.
ACL/IJCNLP(2021)
摘要
Fully non-autoregressive neural machine translation (NAT) is proposed to simultaneously predict tokens with single forward of neural networks, which significantly reduces the inference latency at the expense of quality drop compared to the Transformer baseline. In this work, we target on closing the performance gap while maintaining the latency advantage. We first inspect the fundamental issues of fully NAT models, and adopt dependency reduction in the learning space of output tokens as the basic guidance. Then, we revisit methods in four different aspects that have been proven effective for improving NAT models, and carefully combine these techniques with necessary modifications. Our extensive experiments on three translation benchmarks show that the proposed system achieves the new state-of-the-art results for fully NAT models, and obtains comparable performance with the autoregressive and iterative NAT systems. For instance, one of the proposed models achieves 27.49 BLEU points on WMT14 En-De with approximately 16.5X speed up at inference time.
更多查看译文
关键词
translation,neural,non-autoregressive
AI 理解论文
溯源树
样例
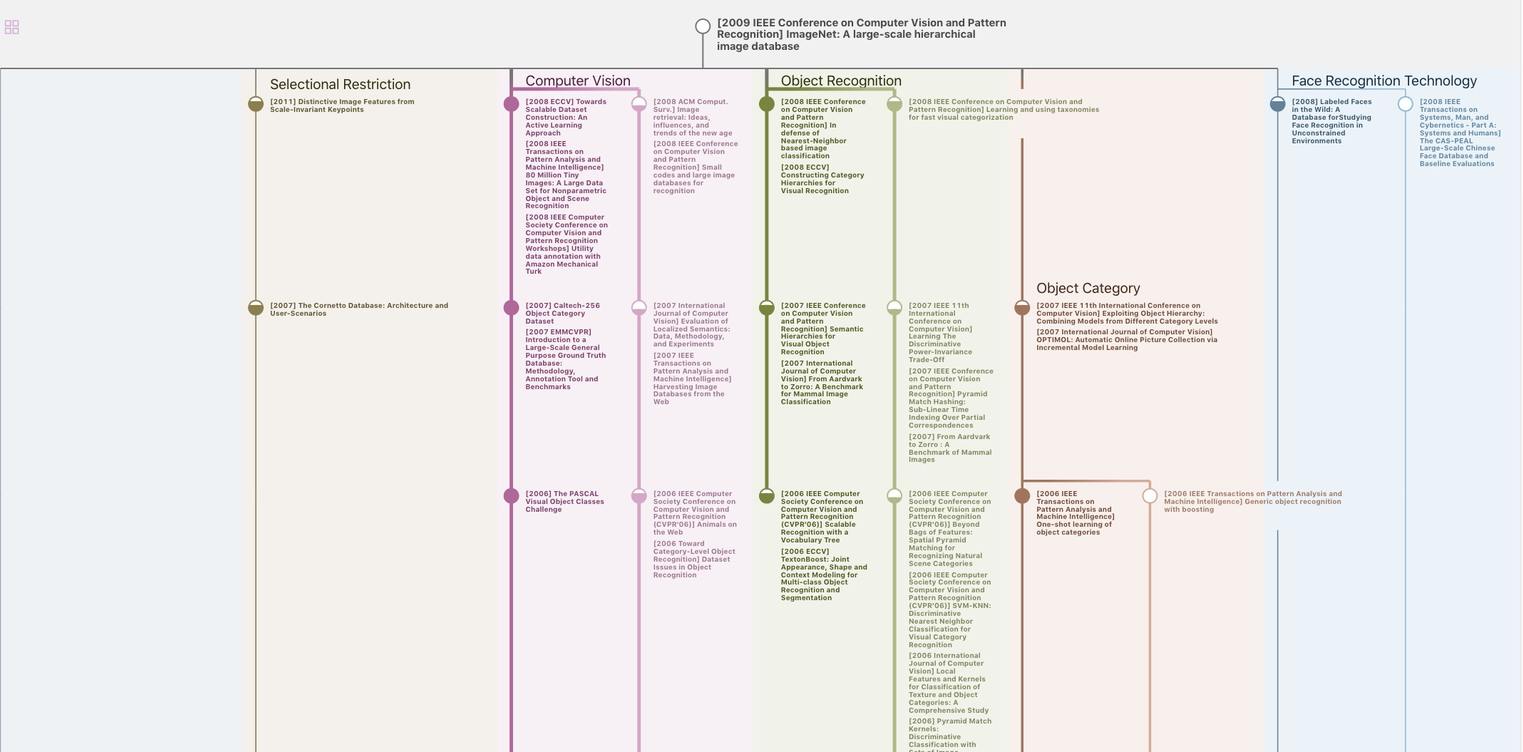
生成溯源树,研究论文发展脉络
Chat Paper
正在生成论文摘要