Inference Of Genetic Networks From Time-Series And Static Gene Expression Data: Combining A Random-Forest-Based Inference Method With Feature Selection Methods
FRONTIERS IN GENETICS(2020)
摘要
Several researchers have focused on random-forest-based inference methods because of their excellent performance. Some of these inference methods also have a useful ability to analyze both time-series and static gene expression data. However, they are only of use in ranking all of the candidate regulations by assigning them confidence values. None have been capable of detecting the regulations that actually affect a gene of interest. In this study, we propose a method to remove unpromising candidate regulations by combining the random-forest-based inference method with a series of feature selection methods. In addition to detecting unpromising regulations, our proposed method uses outputs from the feature selection methods to adjust the confidence values of all of the candidate regulations that have been computed by the random-forest-based inference method. Numerical experiments showed that the combined application with the feature selection methods improved the performance of the random-forest-based inference method on 99 of the 100 trials performed on the artificial problems. However, the improvement tends to be small, since our combined method succeeded in removing only 19% of the candidate regulations at most. The combined application with the feature selection methods moreover makes the computational cost higher. While a bigger improvement at a lower computational cost would be ideal, we see no impediments to our investigation, given that our aim is to extract as much useful information as possible from a limited amount of gene expression data.
更多查看译文
关键词
FANTOM5, gene expression, feature selection, random forest, genetic network inference
AI 理解论文
溯源树
样例
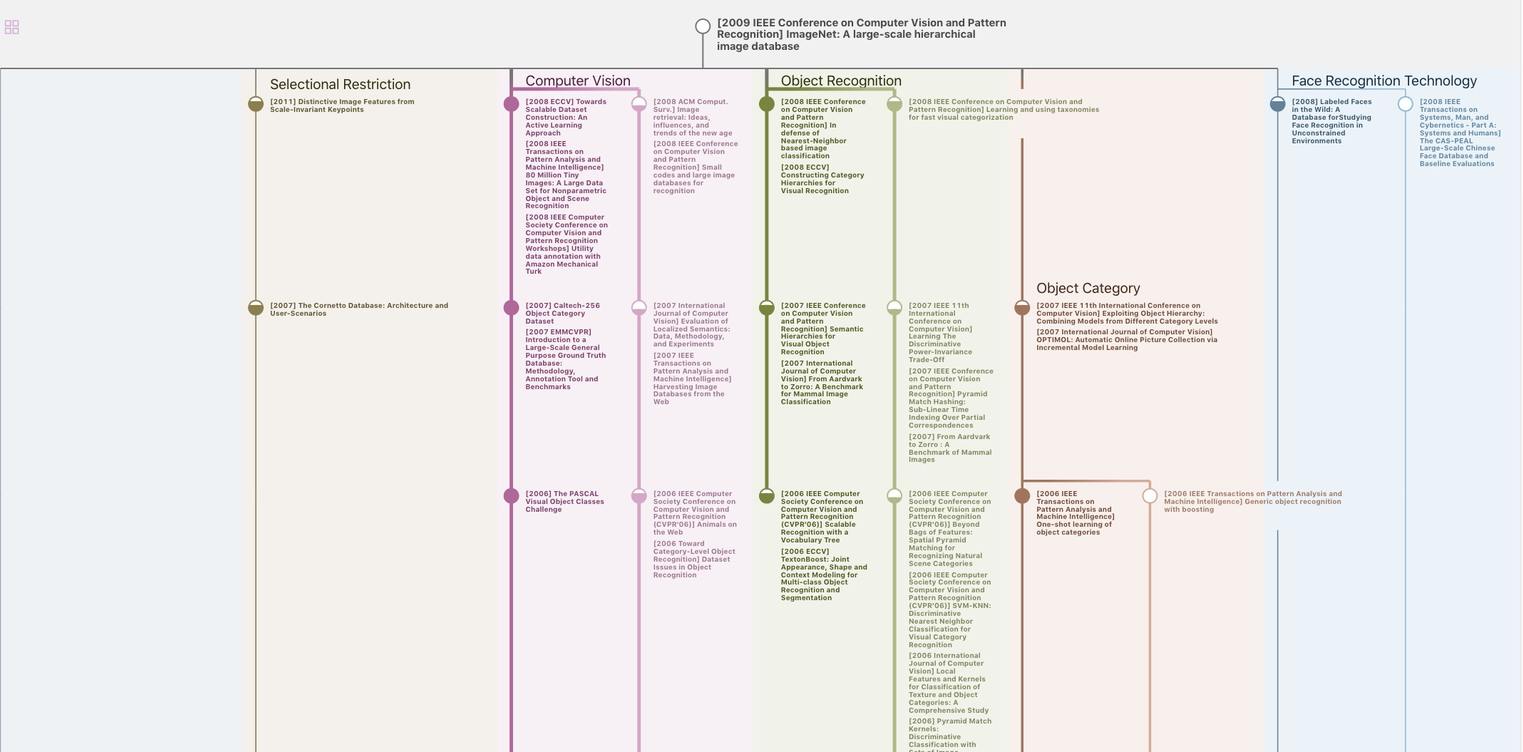
生成溯源树,研究论文发展脉络
Chat Paper
正在生成论文摘要