Feasibility of predicting pancreatic neuroendocrine tumor grade using deep features from unsupervised learning
Proceedings of SPIE(2020)
摘要
This paper aimed to investigate if deep image features extracted via sparse autoencoder (SAE) could be used to preoperatively predict histologic grade in pancreatic neuroendocrine tumors (pNETs). In this study, a total of 114 patients from two institutions were involved. The deep image features were extracted based on the sparse autoencoder network via a 2000-time iteration. Considering the possible prediction error due to the small patient data size, we performed 10-fold cross-validation. To find the optimal hidden size, we set the size as a range of 6-16. The maximum relevance minimum redundancy (mRMR) features selection algorithm was used to select the most histologic grade-related image features. Then the radiomics signature was generated by using the selected features with Support Vector Machine (SVM), Multivariable Logistic Regression (MLR) and Artificial Neural Networks (ANN) methods. The prediction performance was evaluated using AUC value.
更多查看译文
关键词
unsupervised learning,pancreatic neuroendocrine tumors,tumor quantification
AI 理解论文
溯源树
样例
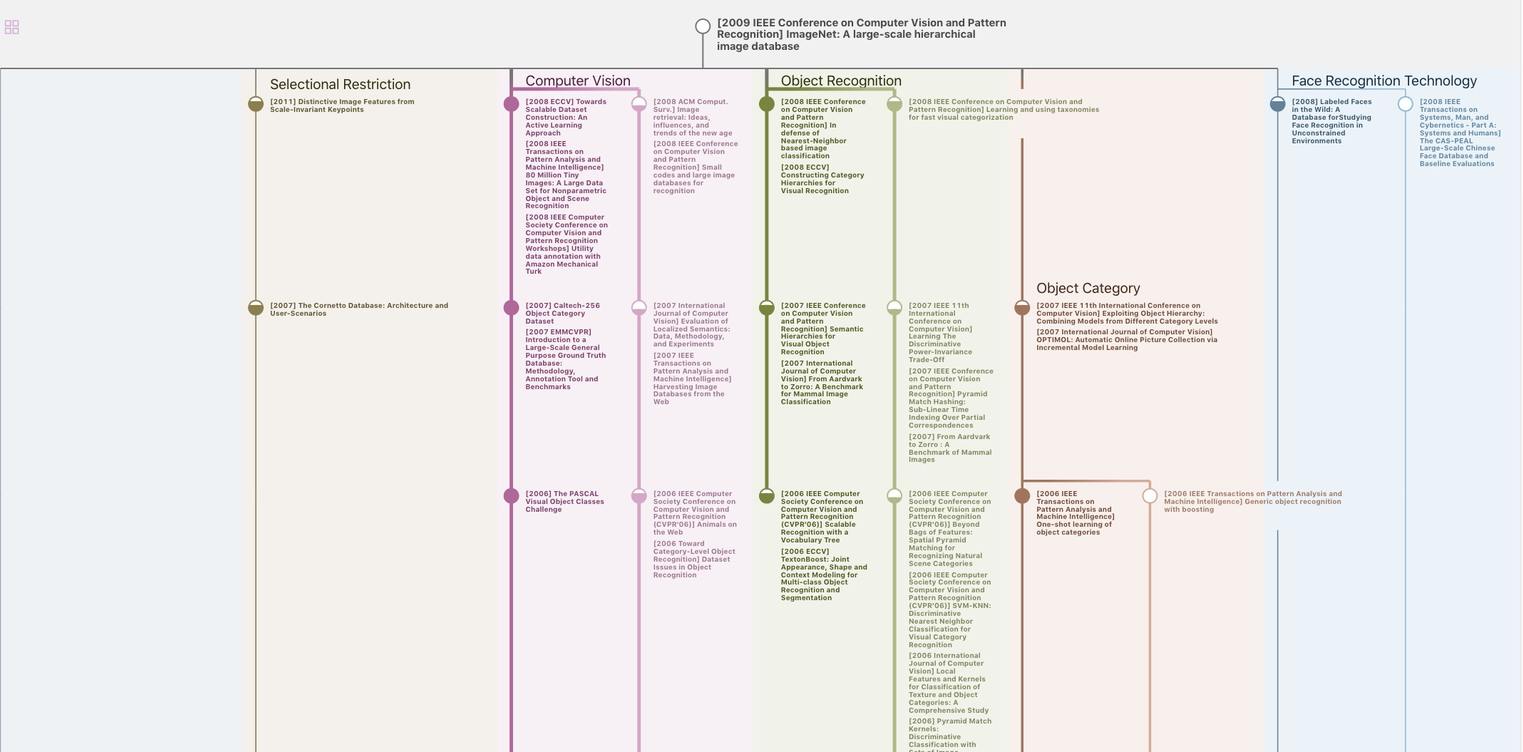
生成溯源树,研究论文发展脉络
Chat Paper
正在生成论文摘要