A Two Stage Adaptive Metropolis Algorithm
arxiv(2020)
摘要
We propose a new sampling algorithm combining two quite powerful ideas in the Markov chain Monte Carlo literature -- adaptive Metropolis sampler and two-stage Metropolis-Hastings sampler. The proposed sampling method will be particularly very useful for high-dimensional posterior sampling in Bayesian models with expensive likelihoods. In the first stage of the proposed algorithm, an adaptive proposal is used based on the previously sampled states and the corresponding acceptance probability is computed based on an approximated inexpensive target density. The true expensive target density is evaluated while computing the second stage acceptance probability only if the proposal is accepted in the first stage. The adaptive nature of the algorithm guarantees faster convergence of the chain and very good mixing properties. On the other hand, the two-stage approach helps in rejecting the bad proposals in the inexpensive first stage, making the algorithm computationally efficient. As the proposals are dependent on the previous states the chain loses its Markov property, but we prove that it retains the desired ergodicity property. The performance of the proposed algorithm is compared with the existing algorithms in two simulated and two real data examples.
更多查看译文
关键词
Markov chain Monte Carlo,metropolis-hastings,two-stage metropolis-hastings,adaptive metropolis,ergodicity
AI 理解论文
溯源树
样例
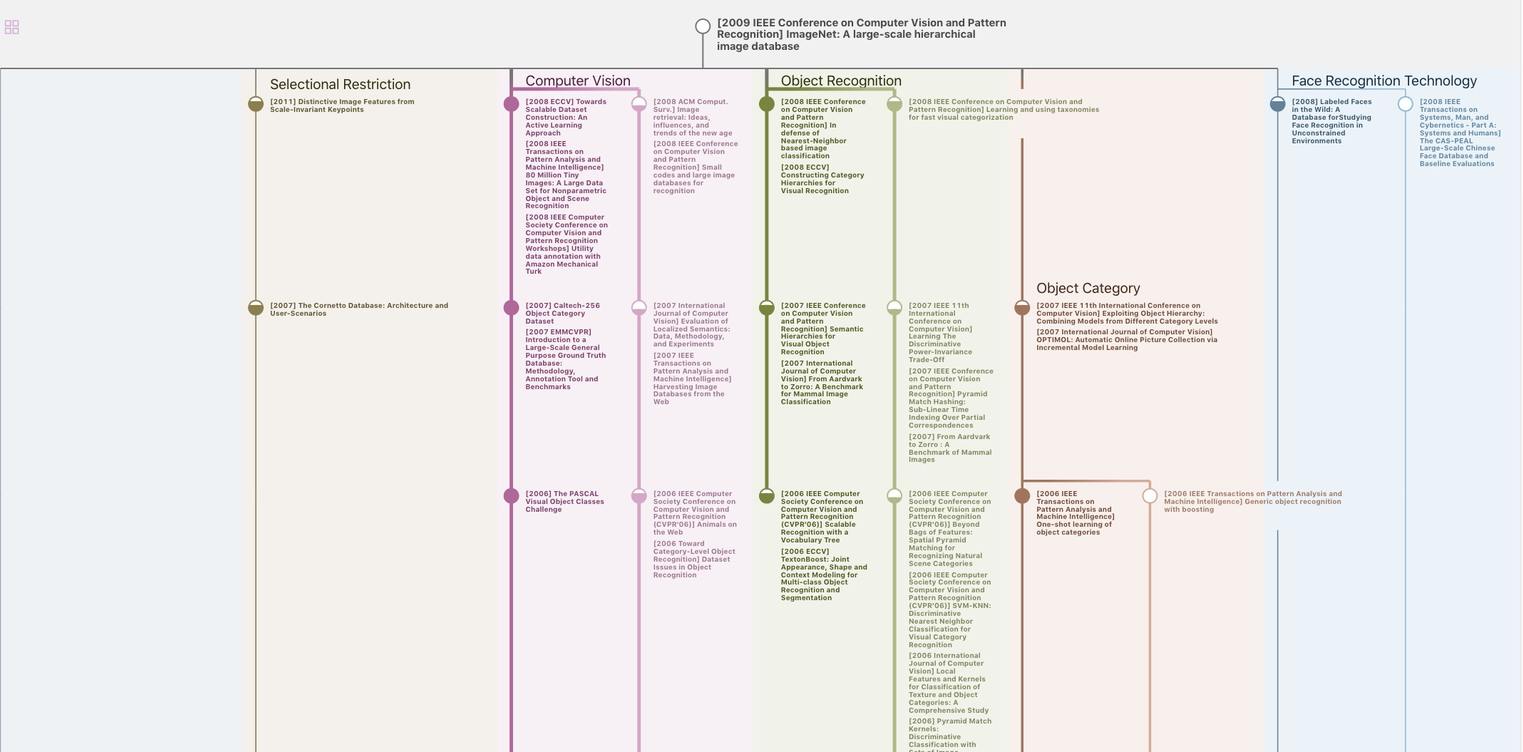
生成溯源树,研究论文发展脉络
Chat Paper
正在生成论文摘要