MrGCN: Mirror Graph Convolution Network for Relation Extraction with Long-Term Dependencies
CoRR(2020)
Abstract
The ability to capture complex linguistic structures and long-term dependencies among words in the passage is essential for many natural language understanding tasks. In relation extraction, dependency trees that contain rich syntactic clues have been widely used to help capture long-term dependencies in text. Graph neural networks (GNNs), one of the means to encode dependency graphs, has been shown effective in several prior works. However, relatively little attention has been paid to the receptive fields of GNNs, which can be crucial in tasks with extremely long text that go beyond single sentences and require discourse analysis. In this work, we leverage the idea of graph pooling and propose the Mirror Graph Convolution Network (MrGCN), a GNN model with pooling-unpooling structures tailored to relation extraction. The pooling branch reduces the graph size and enables the GCN to obtain larger receptive fields within less layers; the unpooling branch restores the pooled graph to its original resolution such that token-level relation extraction can be performed. Experiments on two datasets demonstrate the effectiveness of our method, showing significant improvements over previous results.
MoreTranslated text
Key words
relation extraction,mirror graph convolution network,long-term
AI Read Science
Must-Reading Tree
Example
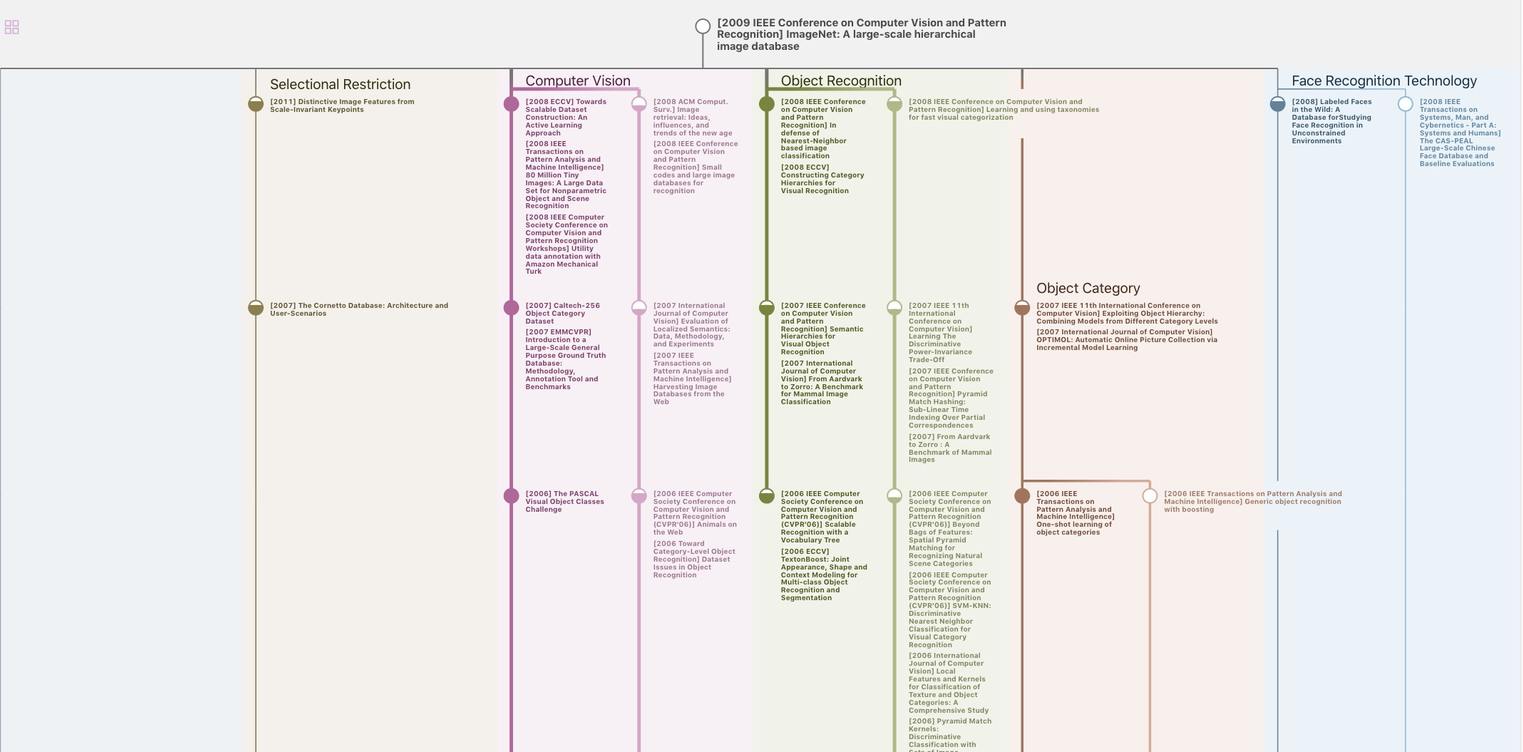
Generate MRT to find the research sequence of this paper
Chat Paper
Summary is being generated by the instructions you defined