A Robust and Domain-Adaptive Approach for Low-Resource Named Entity Recognition
2020 IEEE International Conference on Knowledge Graph (ICKG)(2020)
摘要
Recently, it has attracted much attention to build reliable named entity recognition (NER) systems using limited annotated data. Nearly all existing works heavily rely on domain-specific resources, such as external lexicons and knowledge bases. However, such domain-specific resources are often not available, meanwhile it's difficult and expensive to construct the resources, which has become a key obstacle to wider adoption. To tackle the problem, in this work, we propose a novel robust and domain-adaptive approach RDANER for low-resource NER, which only uses cheap and easily obtainable resources. Extensive experiments on three benchmark datasets demonstrate that our approach achieves the best performance when only using cheap and easily obtainable resources, and delivers competitive results against state-of-the-art methods which use difficultly obtainable domain-specific resources. All our code and corpora can be found on https://github.com/houking-can/RDANER.
更多查看译文
关键词
named entity recognition,low resource,domain adaptive
AI 理解论文
溯源树
样例
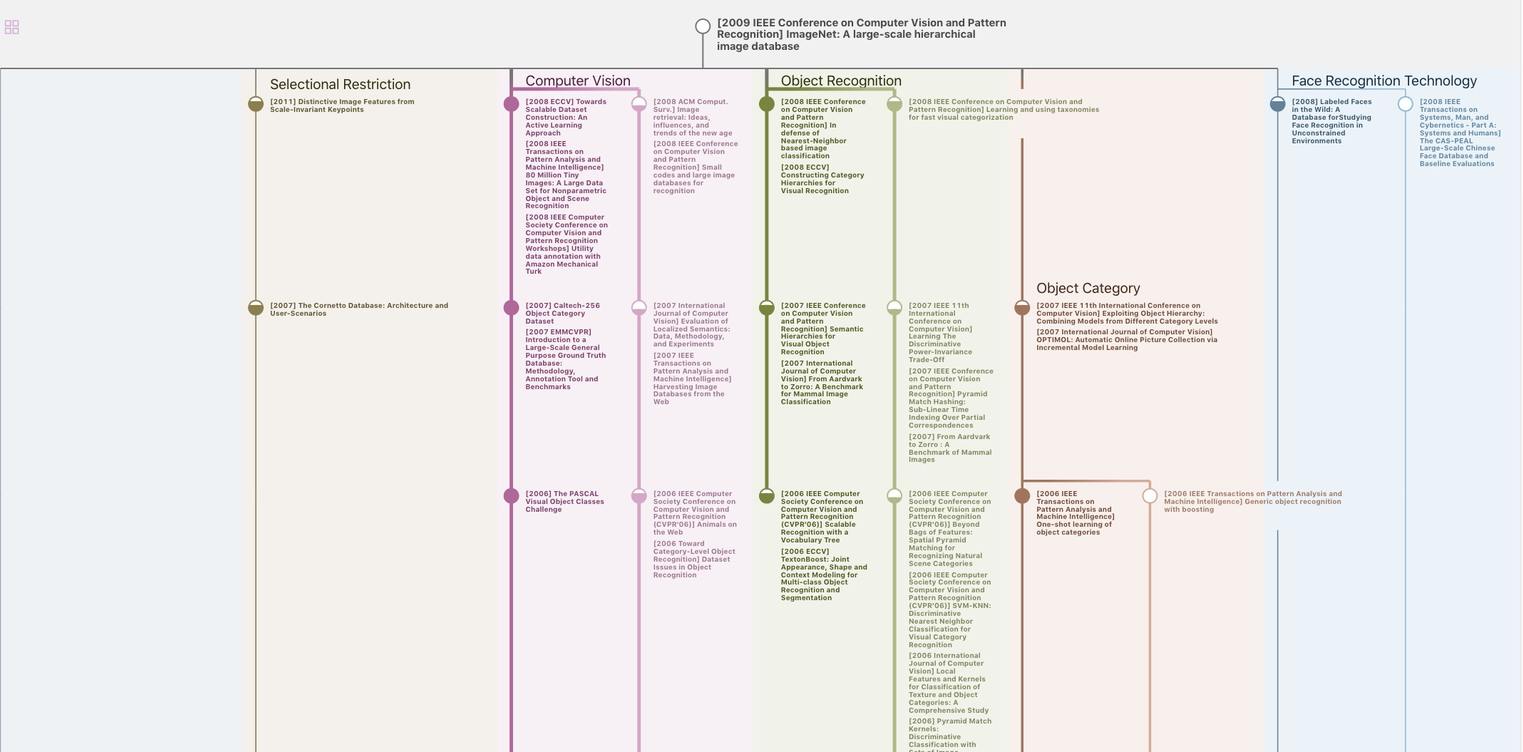
生成溯源树,研究论文发展脉络
Chat Paper
正在生成论文摘要