An Adaptive Rank Continuation Algorithm for General Weighted Low-rank Recovery
arxiv(2022)
摘要
This paper is devoted to proposing a general weighted low-rank recovery model and designing a fast SVD-free computational scheme to solve it. First, our generic weighted low-rank recovery model unifies several existing approaches in the literature.~Moreover, our model readily extends to the non-convex setting. Algorithm-wise, most first-order proximal algorithms in the literature for low-rank recoveries require computing singular value decomposition (SVD). As SVD does not scale appropriately with the dimension of the matrices, these algorithms become slower when the problem size becomes larger. By incorporating the variational formulation of the nuclear norm into the sub-problem of proximal gradient descent, we avoid computing SVD, which results in significant speed-up. Moreover, our algorithm preserves the rank identification property of nuclear norm [33] which further allows us to design a rank continuation scheme that asymptotically achieves the minimal iteration complexity. Numerical experiments on both toy examples and real-world problems, including structure from motion (SfM) and photometric stereo, background estimation, and matrix completion, demonstrate the superiority of our proposed algorithm.
更多查看译文
关键词
adaptive rank continuation algorithm,low-rank
AI 理解论文
溯源树
样例
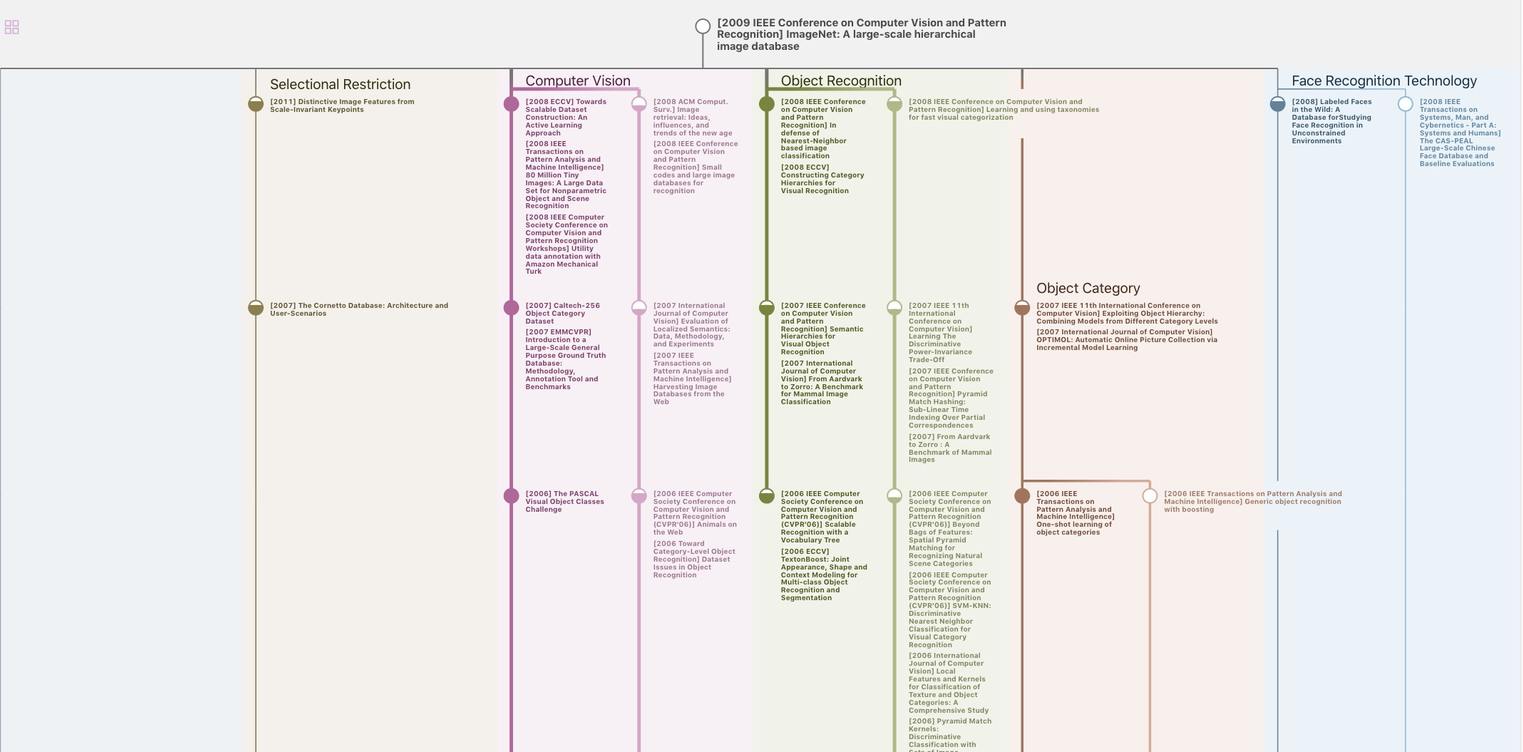
生成溯源树,研究论文发展脉络
Chat Paper
正在生成论文摘要