Temporal Contrastive Graph for Self-supervised Video Representation Learning
arxiv(2021)
摘要
Attempt to fully explore the fine-grained temporal structure and global-local chronological characteristics for self-supervised video representation learning, this work takes a closer look at exploiting the temporal structure of videos and further proposes a novel self-supervised method named Temporal Contrastive Graph (TCG). In contrast to the existing methods that randomly shuffle the video frames or video snippets within a video, our proposed TCG roots in a hybrid graph contrastive learning strategy to regard the inter-snippet and intra-snippet temporal relationships as self-supervision signals for temporal representation learning. Inspired by the neuroscience studies that the human visual system is sensitive to both local and global temporal changes, our proposed TCG integrates the prior knowledge about the frame and snippet orders into temporal contrastive graph structures, i.e., the intra-/inter- snippet temporal contrastive graph modules, to well preserve the local and global temporal relationships among video frame-sets and snippets. By randomly removing edges and masking node features of the intra-snippet graphs or inter-snippet graphs, our TCG can generate different correlated graph views. Then, specific contrastive losses are designed to maximize the agreement between node embeddings in different views. To learn the global context representation and recalibrate the channel-wise features adaptively, we introduce an adaptive video snippet order prediction module, which leverages the relational knowledge among video snippets to predict the actual snippet orders. Extensive experimental results demonstrate the superiority of our TCG over the state-of-the-art methods on large-scale action recognition and video retrieval benchmarks.
更多查看译文
关键词
Task analysis, Representation learning, Discrete cosine transforms, Legged locomotion, Feature extraction, Supervised learning, Streaming media, Video understanding, self-supervised learning, graph neural networks, spatial-temporal data analysis
AI 理解论文
溯源树
样例
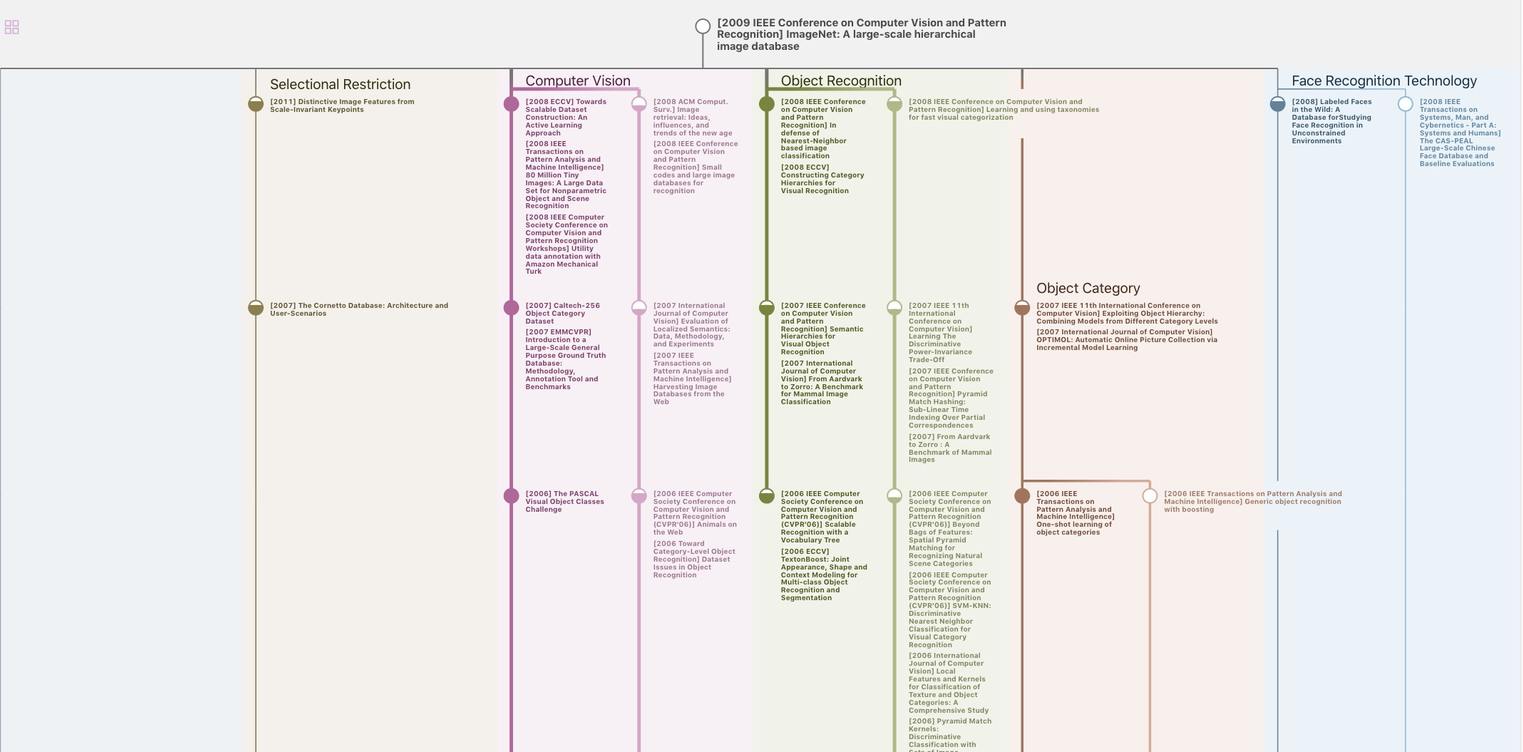
生成溯源树,研究论文发展脉络
Chat Paper
正在生成论文摘要