Learn by Guessing: Multi-step Pseudo-label Refinement for Person Re-Identification
PROCEEDINGS OF THE 17TH INTERNATIONAL JOINT CONFERENCE ON COMPUTER VISION, IMAGING AND COMPUTER GRAPHICS THEORY AND APPLICATIONS (VISAPP), VOL 4(2022)
摘要
Unsupervised Domain Adaptation (UDA) methods for person Re-Identification (Re-ID) rely on target domain samples to model the marginal distribution of the data. To deal with the lack of target domain labels, UDA methods leverage information from labeled source samples and unlabeled target samples. A promising approach relies on the use of unsupervised learning as part of the pipeline, such as clustering methods. The quality of the clusters clearly plays a major role in methods performance, but this point has been overlooked. In this work, we propose a multi-step pseudo-label refinement method to select the best possible clusters and keep improving them so that these clusters become closer to the class divisions without knowledge of the class labels. Our refinement method includes a cluster selection strategy and a camera-based normalization method which reduces the within-domain variations caused by the use of multiple cameras in person Re-ID. This allows our method to reach state-of-the-art UDA results on DukeMTMC -> Market1501 (source -> target). We surpass state-of-the-art for UDA Re-ID by 3.4% on Marken 501 -> DukeMTMC datasets, which is a more challenging adaptation setup because the target domain (DukeMTMC) has eight distinct cameras. Furthermore, the camera-based normalization method causes a significant reduction in the number of iterations required for training convergence.
更多查看译文
关键词
Unsupervised Domain Adaptation, Person Re-Identification, Pseudo-labels, Deep Learning
AI 理解论文
溯源树
样例
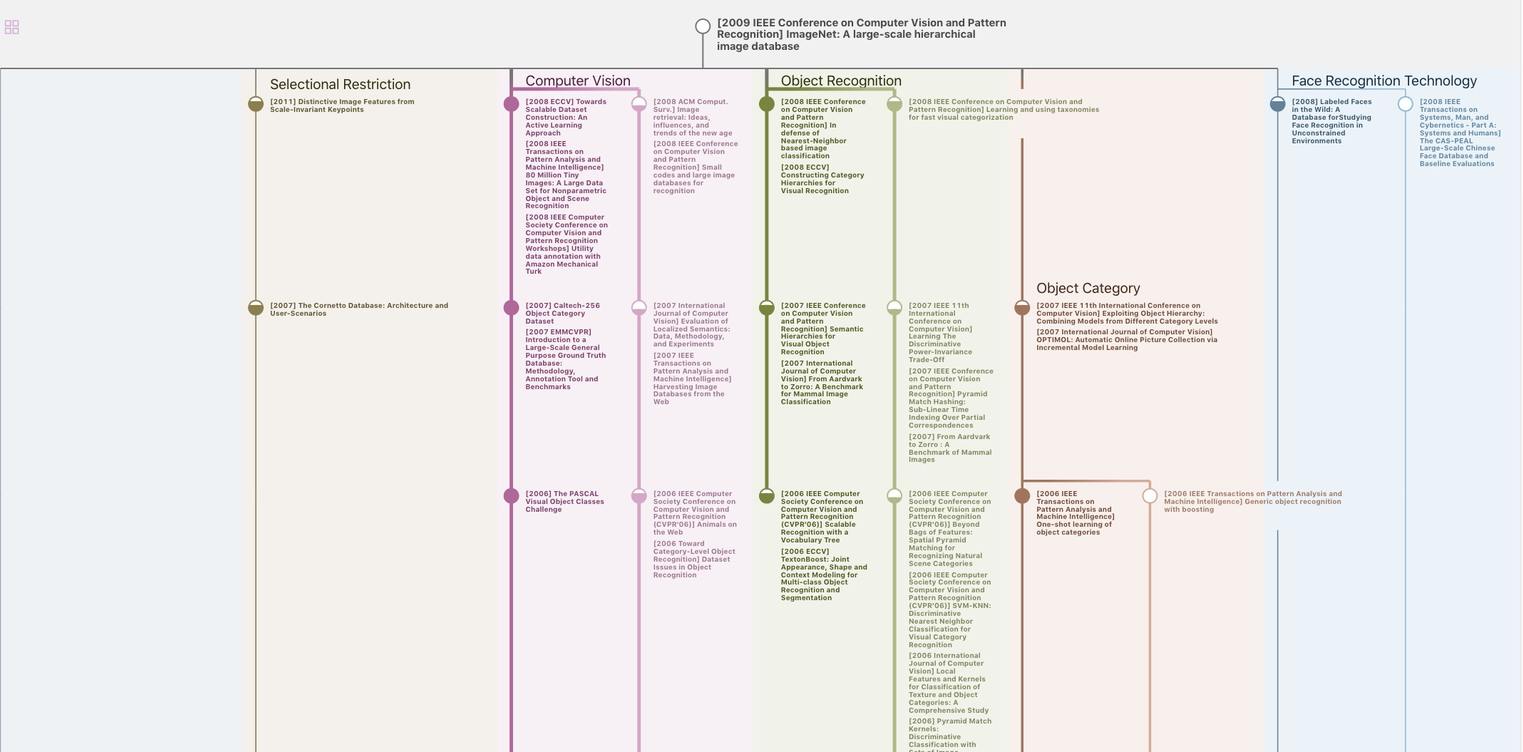
生成溯源树,研究论文发展脉络
Chat Paper
正在生成论文摘要